Market Sentiment Analysis Based on Social Media and Trading Volume for Asset Price Movement Prediction.
ADMA (1)(2023)
摘要
As more and more netizens participate in financial market transactions, online discussions on asset price movements are becoming more comprehensive and timely. Online text, especially from social media, has the potential to be an important data source for financial opinion mining. Market sentiment analysis mainly includes direct analysis methods in the form of text-based surveys and indirect inference methods based on structured data such as price, trading volume, and volatility. In theory, the former is helpful for us to understand investor sentiment earlier, but due to the difficulty of obtaining a sufficient number of objective survey samples, its obtained research attentions are far less than the latter. To combine the advantages and offset the weakness of these two approaches, this paper uses Valence Aware Dictionary and Sentiment Reasoner (VADER) and Fast Fourier Transform (FFT) to construct social media sentiment indexes based on plenty of daily discussion texts about Bitcoin (BTC) and S &P500 (SPX) from Reddit for analyzing their interaction with prices. We also propose a new time series synchronization verification method called Rolling Time-lagged Cross-correlation (RTLCC) surface, and corresponding feature constructing methods, in which RTLCC helps us observe Time-lagged Cross-correlation from the perspective of Rolling Correlation while determining the hyperparameters (Window Size & Time Offset) for features construction. Finally, based on these features, we use four machine learning classifiers for modeling and verify the effectiveness of the proposed market sentiment analysis pipeline, in which on the prediction of 10-day price movements, the best model achieves 89.9% in accuracy (ACC) and 92.5% in AUC.
更多查看译文
关键词
sentiment analysis,trading volume,market,prediction,social media
AI 理解论文
溯源树
样例
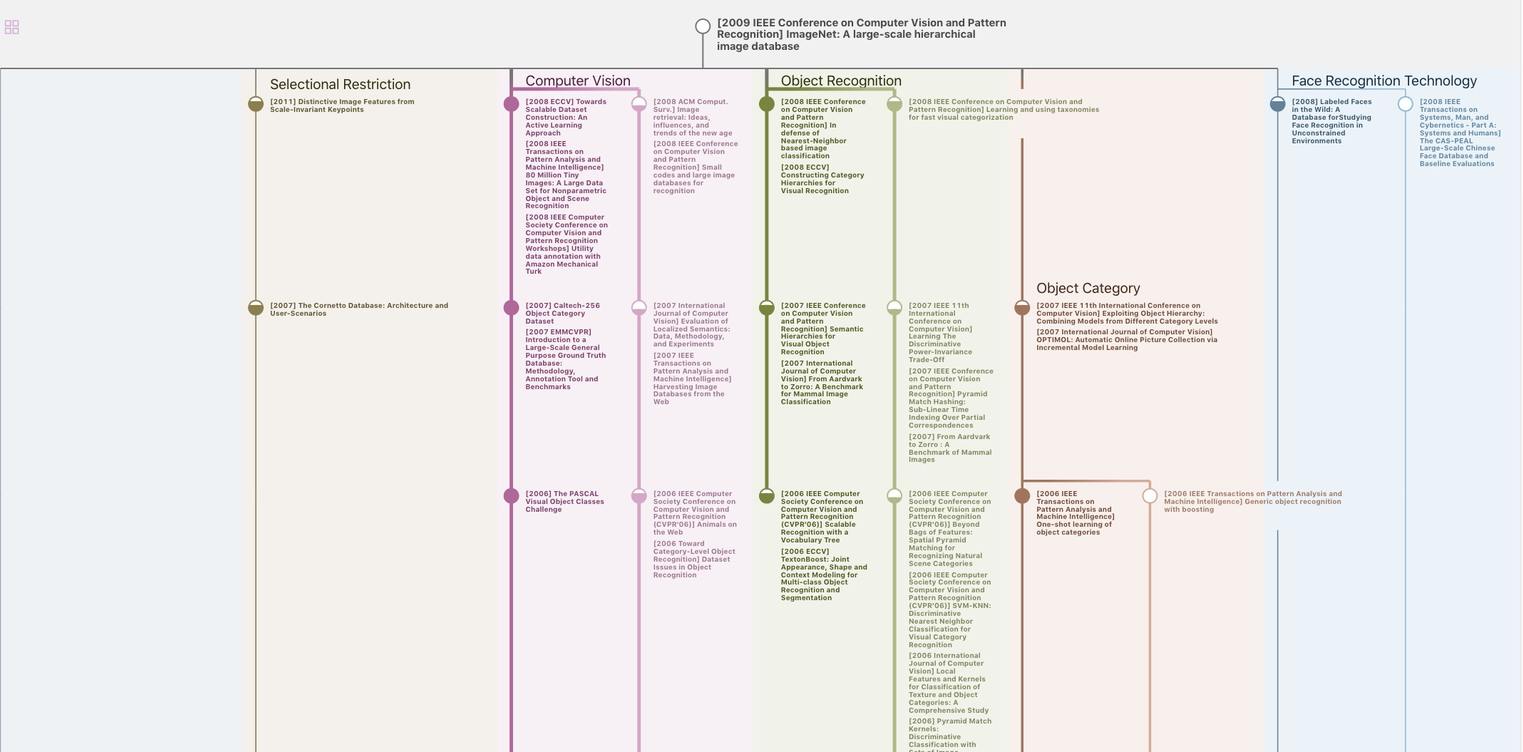
生成溯源树,研究论文发展脉络
Chat Paper
正在生成论文摘要