A Multi-truth Discovery Approach Based on Confidence Interval Estimation of Truths.
ADMA (5)(2023)
摘要
The rapid development of the Internet makes it easier to spread and obtain data. However, conflicting descriptions of an object from different sources make identifying trustworthy information challenging. This is known as the truth discovery task. In truth discovery, an object may have multiple values, such as a book written by multiple authors. Existing multi-truth discovery methods primarily focus on the probability of each candidate value being correct and provide a point estimate. However, practical applications face the problem of unbalanced object distribution, where a single point estimate may overlook critical confidence information. Additionally, ambiguous terms like “etc.” and “et. al” can lead to estimation deviations. To address these issues, we propose MTD_VCI, an optimization model for confidence perception of multiple truths to detect truth from unbalanced data distribution. MTD_VCI estimates the credibility score of each candidate value and considers the confidence interval to reflect the unevenness distribution, improving decision-making. Additionally, the number of values claimed by ambiguous sources is re-estimated using other sources as a reference. Experiment results on real-world and simulated datasets demonstrate that MTD_VCI produces better results and effective confidence intervals for each value.
更多查看译文
关键词
truths,discovery,confidence interval estimation,multi-truth
AI 理解论文
溯源树
样例
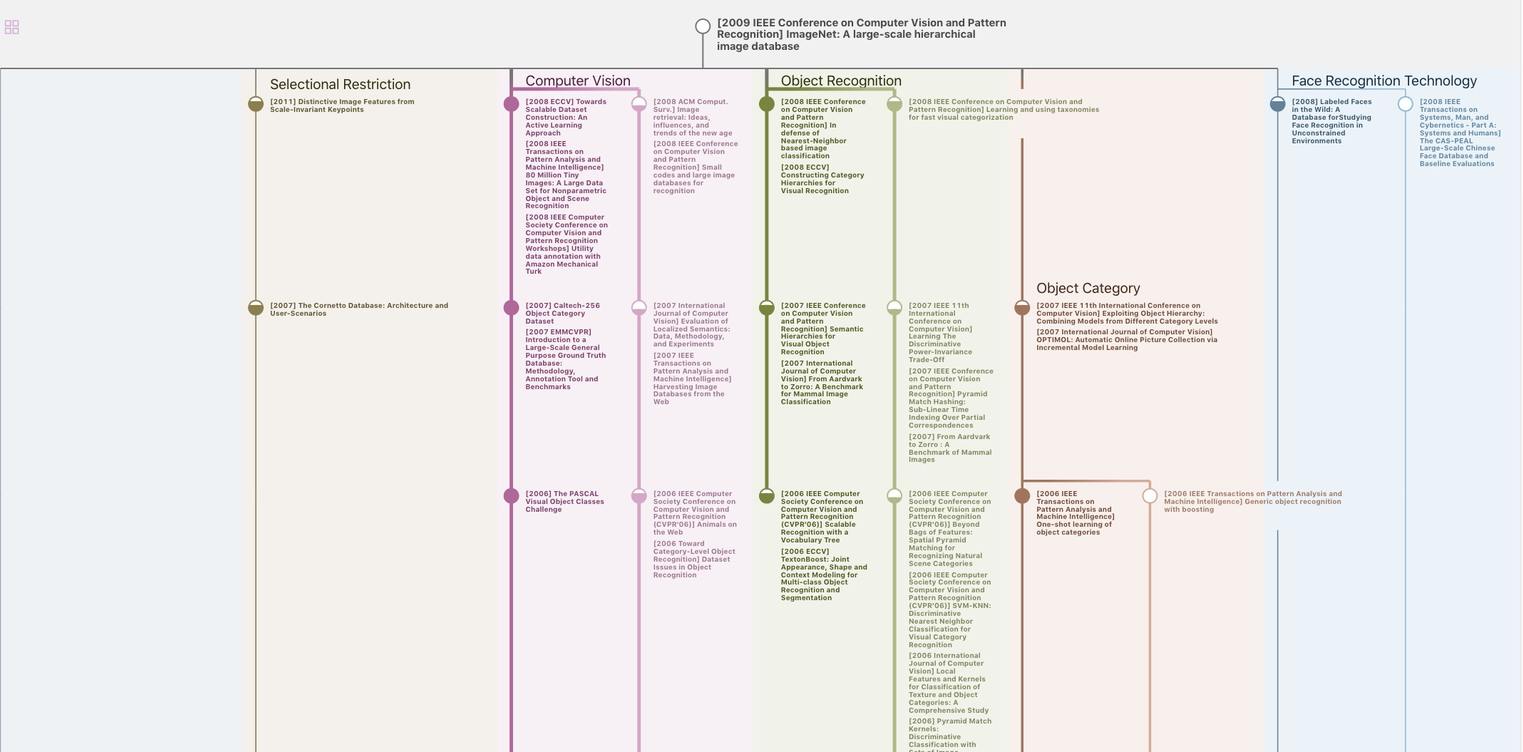
生成溯源树,研究论文发展脉络
Chat Paper
正在生成论文摘要