Efficient Graph Collaborative Filtering with Multi-layer Output-Enhanced Contrastive Learning.
ADMA (1)(2023)
摘要
Recently, Contrastive Learning (CL) is becoming a mainstream approach to reduce the influence of data sparsity in recommendation system. However, existing methods do not fully explore the relationship between the outputs of different Graph Neural Network (GNN) layers and fail to fully utilize the capacity of combining GNN and CL for better recommendation. Within this paper, we introduce a novel approach based on CL, called efficient Graph collaborative filtering with multi-layer output-enhanced Contrastive Learning (GmoCL). It maximizes the benefits derived from the information propagation property of GNN with multi-layer aggregation to obtain better node representations. Specifically, the construction of CL tasks involves considerations from both intra-layer and inter-layer perspectives. The goal of intra-layer CL task is to exploit the semantic similarities of different users (or items) on a certain GNN layer. The inter-layer CL task aims to make the outputs of different GNN layers of the same user (or item) more similar. Additionally, we propose the strategy of negative sampling in the inter-layer CL task to learn the better node representations. The efficacy of the suggested approach is validated through comprehensive experiments conducted on five publicly available datasets.
更多查看译文
关键词
efficient graph collaborative filtering,contrastive learning,multi-layer,output-enhanced
AI 理解论文
溯源树
样例
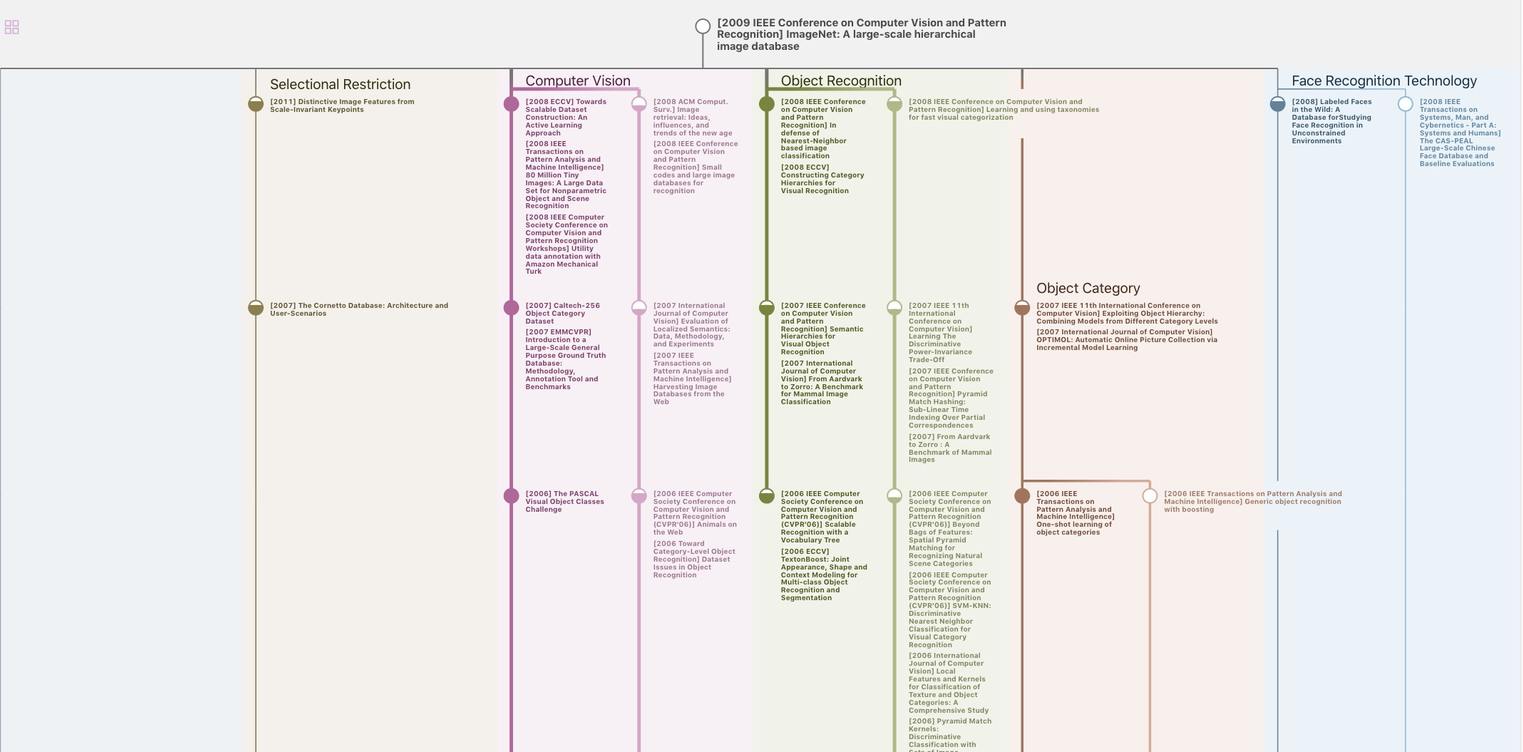
生成溯源树,研究论文发展脉络
Chat Paper
正在生成论文摘要