Short Communication: Localized Adversarial Artifacts for Compressed Sensing MRI
SIAM JOURNAL ON IMAGING SCIENCES(2023)
摘要
As interest in deep neural networks (DNNs) for image reconstruction tasks grows, their reliability has been called into question [V. Antun, F. Renna, C. Poon, B. Adcock, and A. C. Hansen, Proc. Natl. Acad. Sci. USA, 117 (2020), pp. 30088-30095; N. M. Gottschling, V. Antun, B. Adcock, and A. C. Hansen, The Troublesome Kernel: Why Deep Learning for Inverse Problems Is Typically Unstable, preprint, arXiv:2001.01258, 2020]. However, recent work has shown that, compared to total variation (TV) minimization, when appropriately regularized, DNNs show similar robustness to adversarial noise in terms of l(2)-reconstruction error [M. Genzel, J. Macdonald, and M. Marz, IEEE Trans. Pattern Anal., 45 (2022), pp. 1119-1134]. We consider a different notion of robustness, using the l(infinity)-norm, and argue that localized reconstruction artifacts are a more relevant defect than the l(2)-error. We create adversarial perturbations to undersampled magnetic resonance imaging measurements (in the frequency domain) which induce severe localized artifacts in the TV-regularized reconstruction. Notably, the same attack method is not as effective against DNN-based reconstruction. Finally, we show that this phenomenon is inherent to reconstruction methods for which exact recovery can be guaranteed, as with compressed sensing reconstructions with l(1)- or TV-minimization.
更多查看译文
关键词
compressed sensing,magnetic resonance imaging,deep neural networks,total variation,adversarial examples
AI 理解论文
溯源树
样例
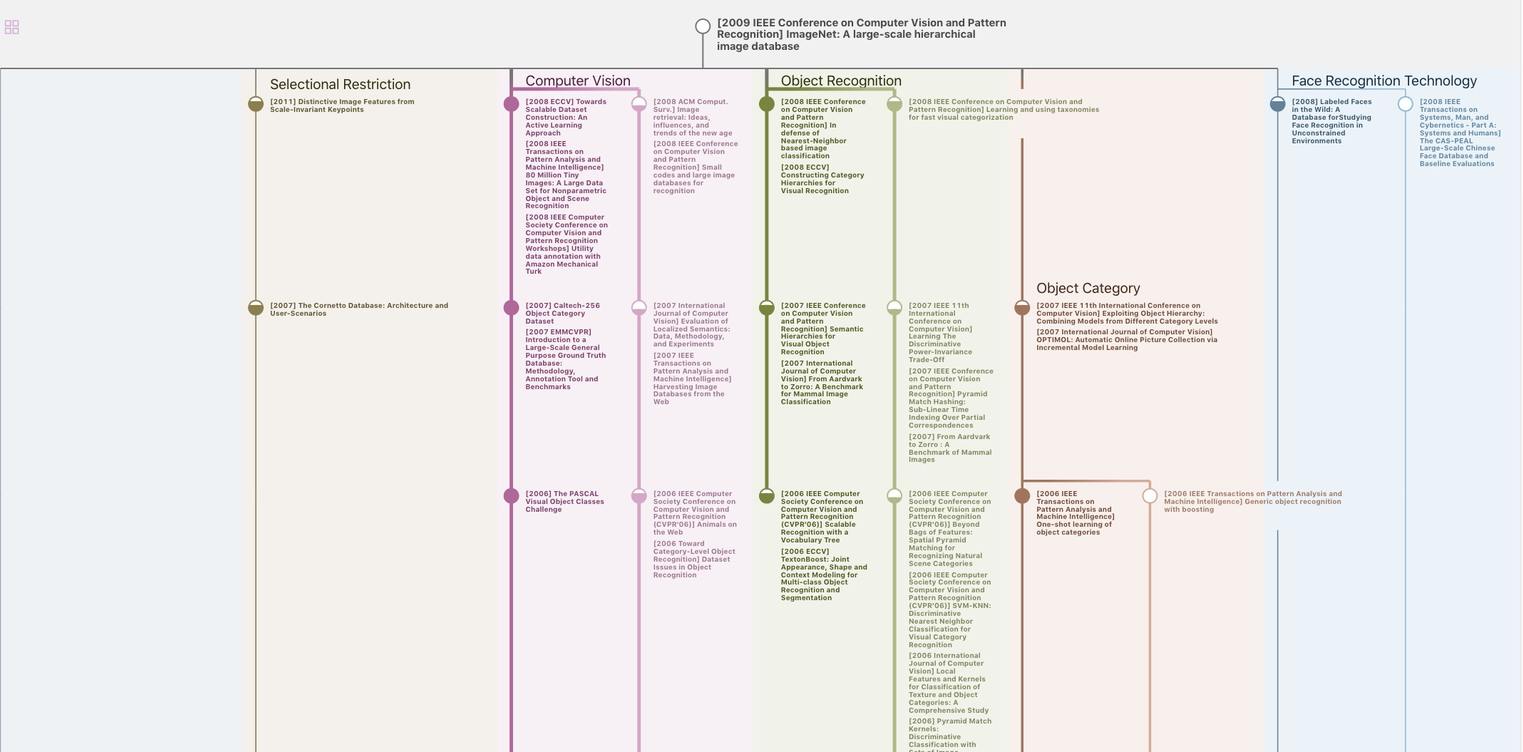
生成溯源树,研究论文发展脉络
Chat Paper
正在生成论文摘要