A Spatial Filter Temporal Graph Convolutional Network for decoding motor imagery EEG signals
EXPERT SYSTEMS WITH APPLICATIONS(2024)
摘要
Due to the influence of volume conduction, information redundancy exists between electrodes in electroen-cephalogram (EEG) signals, resulting in low spatial resolution. Furthermore, the current method of extracting EEG spatial features in Euclidean space cannot accurately depict the complex relationships among multiple electrodes, leading to poor decoding performance of EEG signals. A novel decoding framework for motor imagery EEG signals, known as the Spatial Filter Temporal Graph Convolutional Network (SF-TGCN), is proposed in this study. The spatial resolution of motor imagery EEG signals is enhanced by constructing the spatial filtering module using the Laplacian graph operator. Deep temporal features of the EEG signals are extracted using multiple layers of temporal convolutional modules, and the graph convolutional network module is employed to learn the topology of electrode connections, facilitating the decoding of motor imagery EEG signals. Superior performance is demonstrated by the proposed decoding model, achieving an average accuracy of 80.82% on the BCI IV 2a public dataset and 87.98% on a private dataset, as compared to other state-of-the-art decoding approaches.
更多查看译文
关键词
Electroencephalogram (EEG),Direct Transfer Function (DTF),Functional connectivity,Graph Convolutional Network (GCN),Brain-Computer Interface (BCI)
AI 理解论文
溯源树
样例
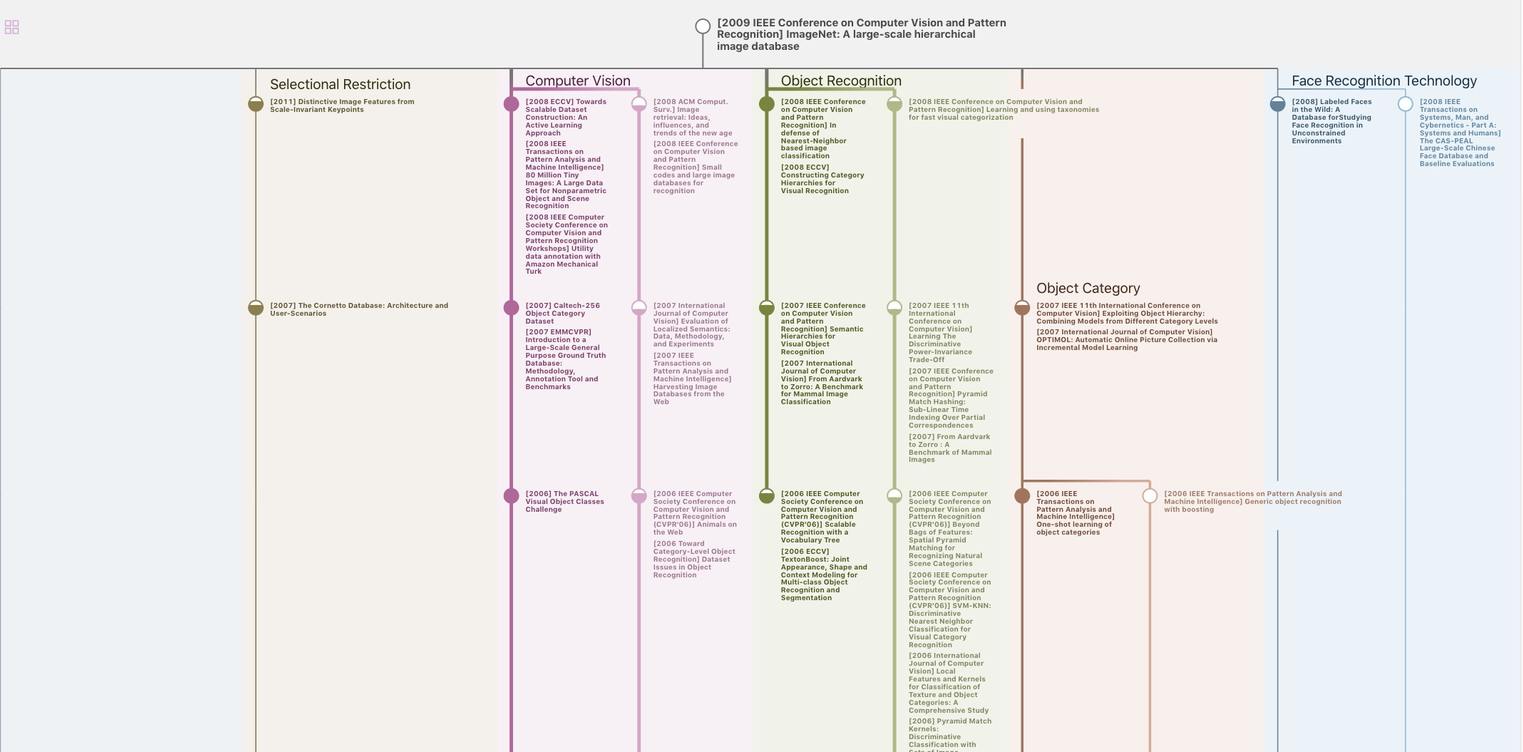
生成溯源树,研究论文发展脉络
Chat Paper
正在生成论文摘要