A semi-automated coding scheme for occupational injury data: An approach using Bayesian decision support system
EXPERT SYSTEMS WITH APPLICATIONS(2024)
摘要
Introduction: Over the past few years, classic Machine Learning approaches such as Multinomial Naive Bayes, Support Vector Machine as well as regularized Logistic Regression have been adapted to autocode injury narratives collected by the Bureau of Labour Statistics to reduce the manual effort needed to assign codes to these narratives. However, the effectiveness of these algorithms is yet to be explored on severe injury reports collected by the Occupational Safety and Health Administration (OSHA). This study aims to explore the performances of two Bayesian models for autocoding these reports, segregate narratives that require manual reviewing, and analyse the usefulness of presenting top k predictions for such reviews to human coders. Method: The severe injury reports collected by OSHA from January 2015 to February 2021 were used in this study. Firstly, Unigram (UNB) and Bigram (BNB) Naive Bayes models are used to classify the injury narratives, and their performance is analyzed. Furthermore, two filtering strategies are used a) Instances where the UNB and BNB models agree are autocoded b) Only cases where the two models agree and whose prediction probability is above a minimum threshold are autocoded. The remaining cases are filtered out to be reviewed and coded by manual coders. The sensitivity of top k predictions for the UNB, BNB, and UNB-BNB models are also compared and analyzed to aid human coders in assigning codes to the narratives that are filtered out. Results: For fully autocoded data, the sensitivity of the UNB model is 75.21%, and that of the BNB model is 75.17%. The filtering approach has an overall sensitivity of 88.17%, flagging 31% of the injury narratives for manual review. The UNB model performs slightly better than the BNB model, and the accuracy increases as cases where the two models agree are considered and a prediction probability threshold is set. For the top 5 predictions, a maximum F1-score of 55% is achieved by the UNB-BNB model.
更多查看译文
关键词
Occupational safety,Injury code,Naive Bayes Model,Prediction
AI 理解论文
溯源树
样例
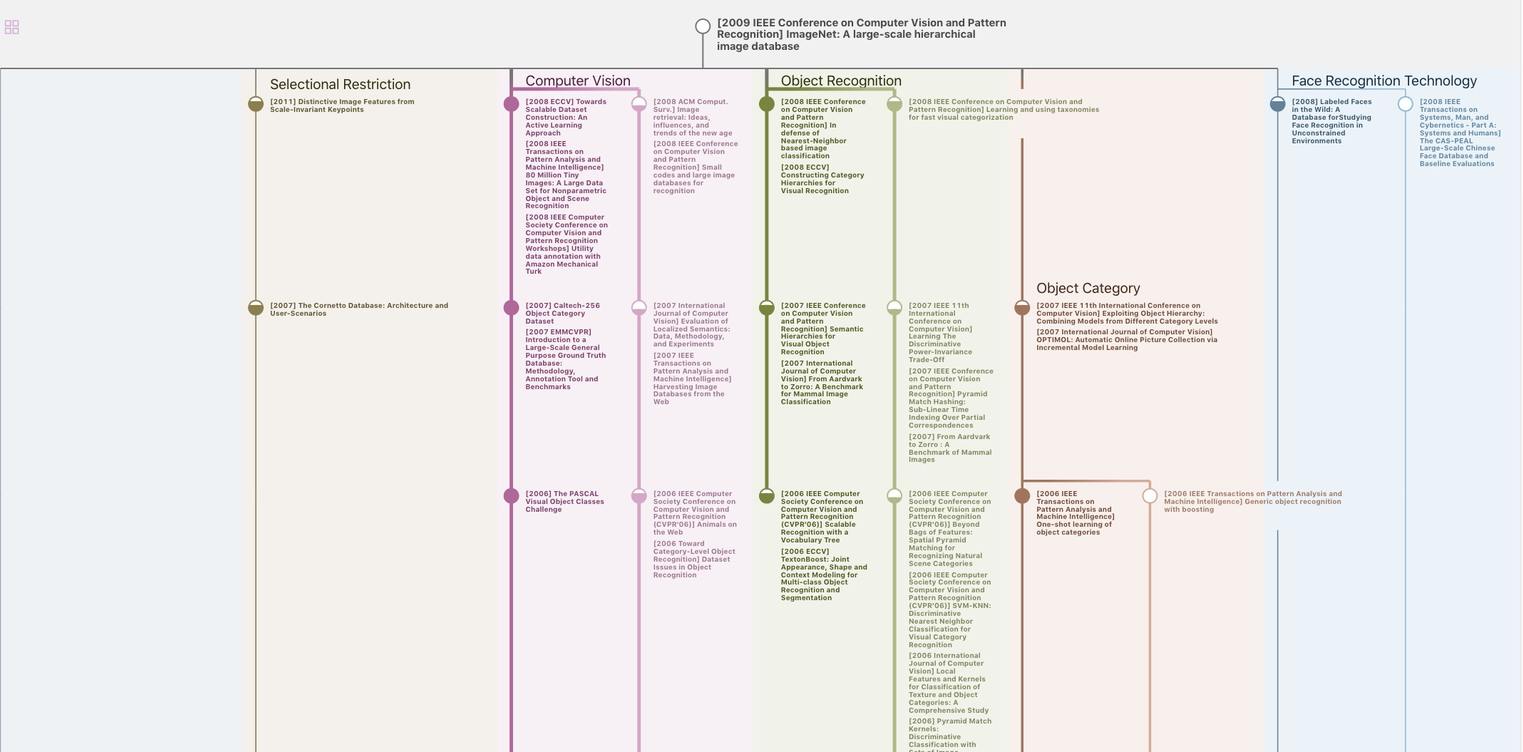
生成溯源树,研究论文发展脉络
Chat Paper
正在生成论文摘要