Unleashing the power of context: Contextual association network with cross-task attention for joint relational extraction
EXPERT SYSTEMS WITH APPLICATIONS(2024)
摘要
Extraction of entities and their relationships remains a challenge in information extraction tasks such as opinion recognition and event detection. Research efforts has been dedicated to training named entity recognition (NER) models and relation extraction (RE) models simultaneously, in order to leverage the semantic associations among such sub-tasks to improve their performance. However, how to reasonably capture the interdependence between these two sub-tasks is still not clear. Well trained joint extraction models often do not generalize well to examples from wide sources due to that existing strategies may overly rely on shallow heuristics via entity mentions and fail to make enough use of contextual associations among such sub-tasks. With this in mind, we propose a novel contextual association network for joint relational extraction (called CARE) with cross-task attention mechanism, which makes use of the correlated contextual information produced by the NER and RE sub-tasks explicitly, and achieves significantly better performance comparing with the state-of-the-art models. We use experimental results on four publicly available datasets to test the efficacy of our proposed model. Experimental results demonstrate that the proposed mechanism can use the semantic information produced by NER and RE sub-tasks to boost one another in a complementary way.
更多查看译文
关键词
Joint relational extraction,Named entity recognition,Cross-task attention,Span-based relation extraction
AI 理解论文
溯源树
样例
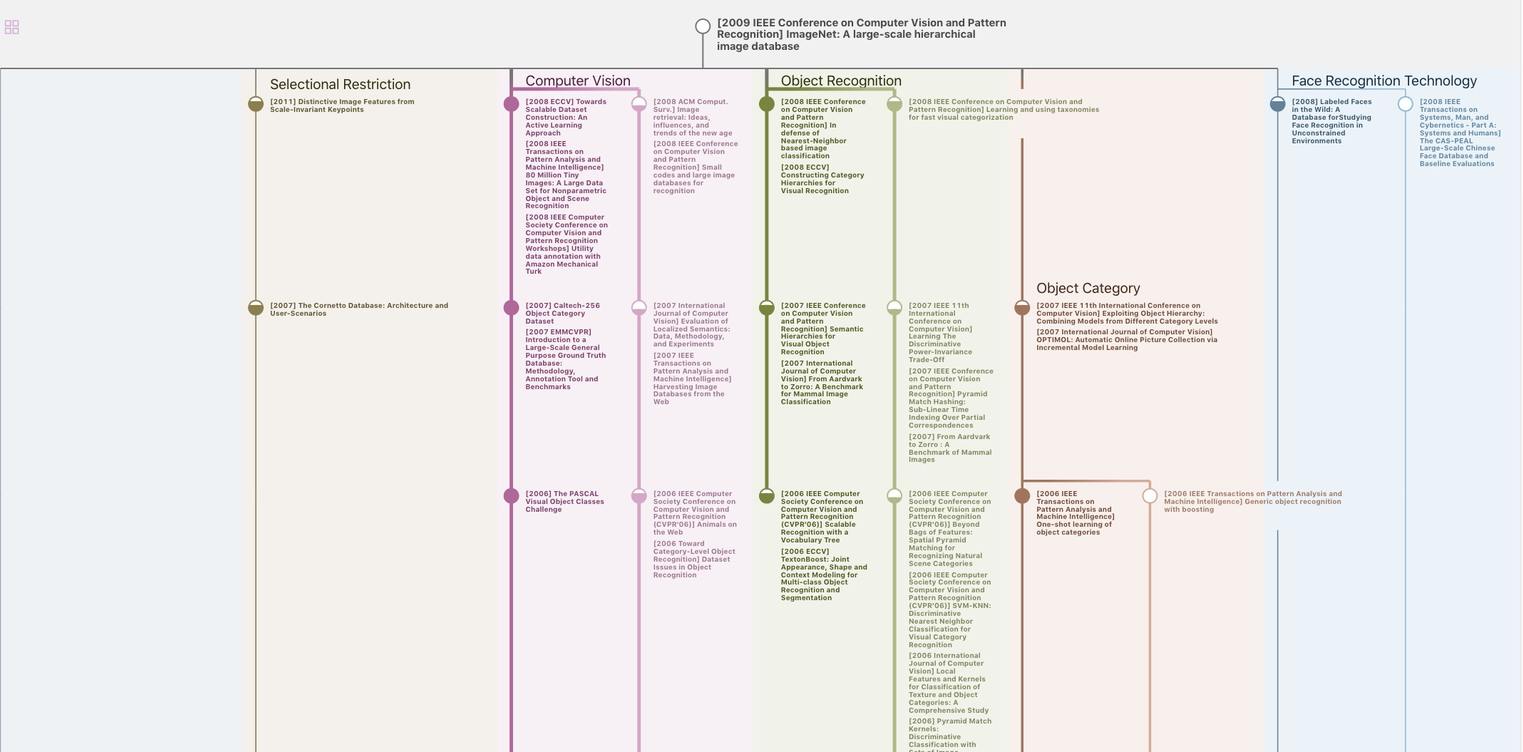
生成溯源树,研究论文发展脉络
Chat Paper
正在生成论文摘要