rgfc-Forest: An enhanced deep forest method towards small-sample fault diagnosis of electromechanical system
EXPERT SYSTEMS WITH APPLICATIONS(2024)
摘要
Deep forest models offer a promising alternative to traditional deep neural networks by demanding fewer training samples and hyperparameters. However, existing deep forest fault diagnosis models encounter persistent challenges such as insufficient representation of multi-grained spatial information and redundancy of cascaded forest features. To address the above challenges, an enhanced deep forest method called random multi-grained fusion cascade forest (rgfc-Forest) is presented for fault diagnosis of electromechanical systems with limited training samples. First, a random multi-grained scanning module is designed to improve feature information learning. Subsequently, a feature fusion cascade forest module is constructed to improve the representativeness of features in multi-grained scanning and cascade forest delivery while ensuring data diversity. Finally, a decision tree self-growth strategy is combined to refine the classification capability of the high-level forest. To evaluate the effectiveness of our proposed method, we applied it to experimental data related to motor system and gearbox faults. Our results demonstrate significant improvements over existing methods: With just 20 samples per class, our method achieved an average accuracy of 84.41% for motor System Diagnosis. Similarly, for the gearbox system, we attained an impressive accuracy of up to 92.72% with the same limited dataset. These outcomes underscore the superior feature representation and fault classification capabilities of our approach compared to both benchmark deep forest models and mainstream deep learning methods when confronted with small training datasets.
更多查看译文
关键词
Fault diagnosis,Small training samples,rgfc-Forest,Random multi-grained scanning,Feature fusion cascade forest
AI 理解论文
溯源树
样例
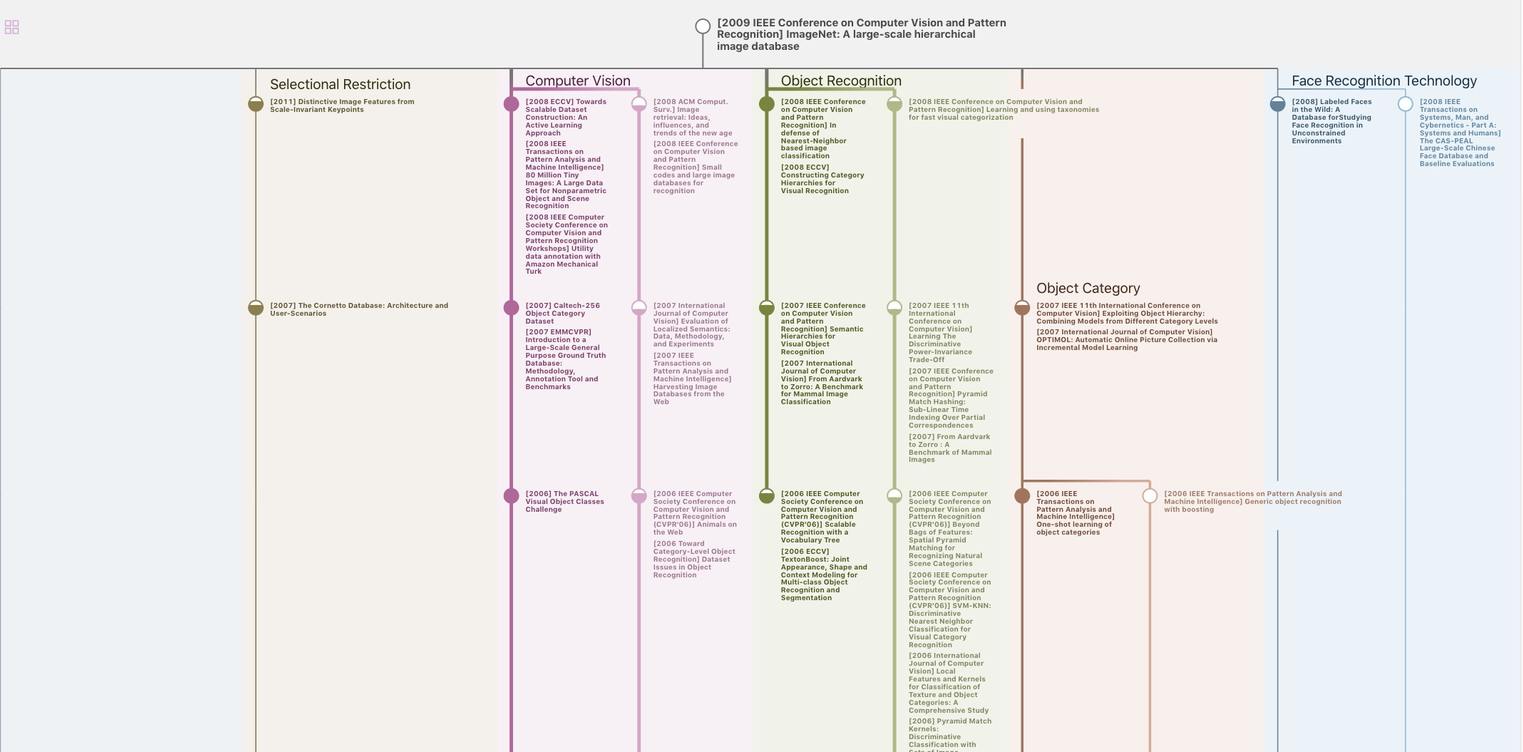
生成溯源树,研究论文发展脉络
Chat Paper
正在生成论文摘要