A prospective study: Advances in chaotic characteristics of serum Raman spectroscopy in the field of assisted diagnosis of disease
EXPERT SYSTEMS WITH APPLICATIONS(2024)
摘要
Chaos theory is an important branch of mathematics and its theory has been widely applied in many fields such as physics and medicine. Based on existing spectroscopic techniques, this paper used chaos theory as a research method for nonlinear time series to analyze Raman spectral time domain curves in order to improve the performance of disease diagnostic models and explore a new paradigm of spectroscopic technology for intelligent assisted disease diagnosis. We quantitatively identified the chaotic characteristics of time domain Raman spectra by three methods, extracted chaotic features such as correlation dimension and Kolmogorov entropy, and used the chaotic features as input to Extreme Learning Machine (ELM), Back Propagation Neural Network (BPNN), K-Nearest Neighbor (KNN) and Support Vector Machine (SVM) to diagnose patients with lung cancer (LC), glioma, renal cell carcinoma (RCC) and esophageal cancer (EC). The Raman spectra were also analyzed by traditional spectral feature extraction & modelling method, and the results of traditional spectral feature extraction & modelling method and chaotic feature modelling method were compared. The experimental results showed that the extraction of effective chaotic features in the full spectral range could achieve comparable diagnostic results with the traditional spectral feature extraction & modelling method. To further validate the effectiveness of chaos theory in Raman spectral data, the full spectrum was divided into three consecutive subsequences of 500-1000, 1000-1500, and 1500-2000 cm(-1), and the above experimental steps were repeated respectively, and the results of the traditional spectral feature extraction & modelling method and chaotic feature modelling were compared. The results showed that as the spectral range was split into consecutive subsequences, the diagnostic performance of the chaotic features in each subsequence performed better than that of conventional spectral analysis techniques. In this study, the technique bridges the gap in the application of chaotic signals to Raman spectroscopy techniques, focuses on global features in the time domain profile of Raman spectra, and demonstrates the significant value of chaos theory in artificial intelligence-assisted spectroscopic medical diagnosis.
更多查看译文
关键词
chaos theory,raman spectroscopy,intelligent assisted disease diagnosis,machine learning
AI 理解论文
溯源树
样例
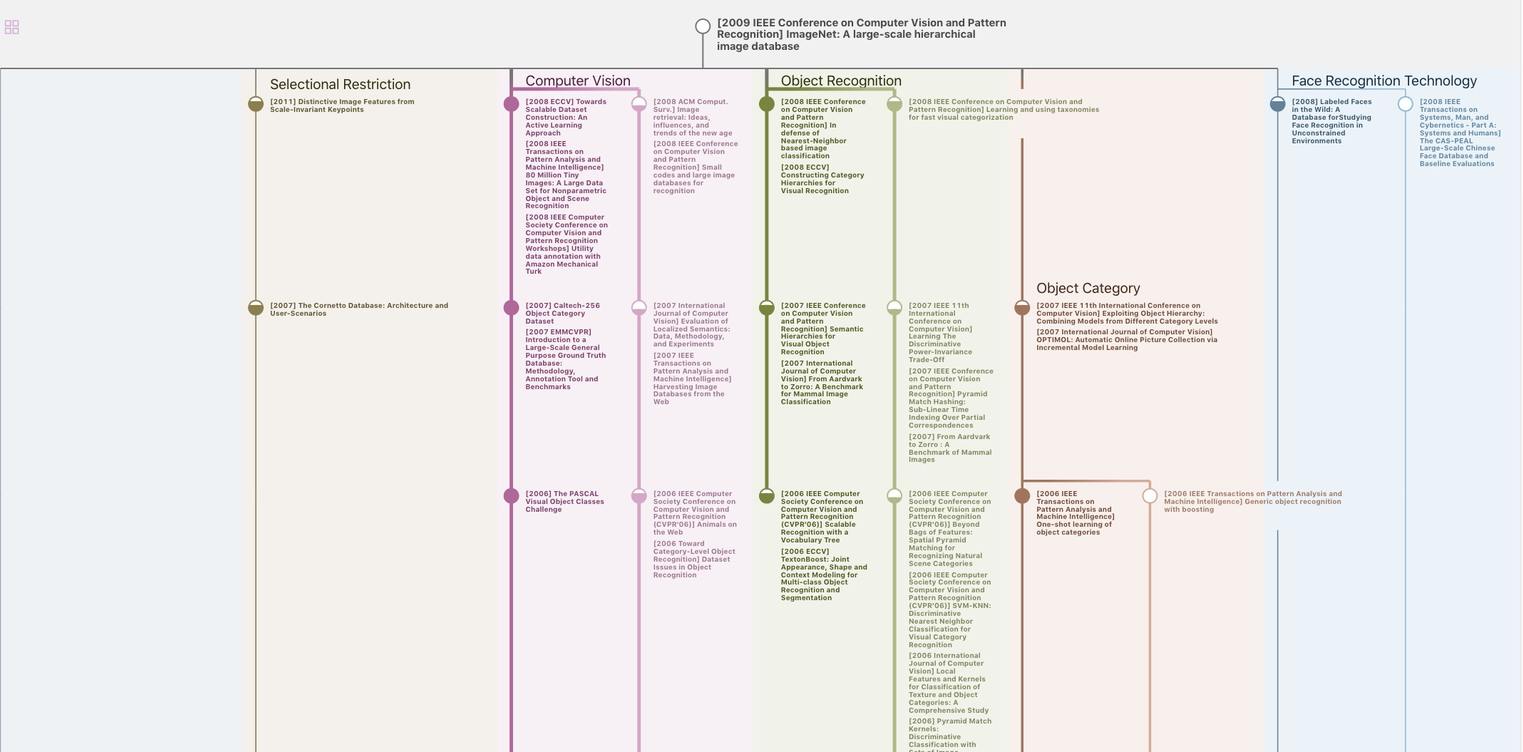
生成溯源树,研究论文发展脉络
Chat Paper
正在生成论文摘要