Region-scalable fitting-assisted medical image segmentation with noisy labels
EXPERT SYSTEMS WITH APPLICATIONS(2024)
摘要
To aid in diagnosis and analysis, deep learning has been applied to medical image analysis by many researchers. Most existing methods train models using many images with precise labels. However, medical images are inherently complex and noisy, making it difficult for medical image segmentation to obtain many accurate labels. That means the study of learning with noisy labels is particularly important. In order to make use of all the samples, some methods correct the noisy labels during the training process. However, in the correction process, a label correction error may occur according to the set policy. Therefore, we propose a method that incorporates image features based on region-scalable fitting (RSF) to assist in the label correction process, called RSF-assisted. We tested the performance of our RSF-assisted method on two learning frameworks of learning with noisy labels based on segmentation: mean-teacher-assisted confident learning (MTCL) and adaptive early-learning correction (ADELE). The experimental results indicate that the performance is improved by using RSF-assisted. To be specific, compared with the original methods, the mean intersection over union (mIOU) is improved by 2.41% after RSF-assisted on thoracic organs (SegTHOR) dataset. Similarly, the 95% Hausdorff distance (95HD) is reduced by up to 2.96 mm after RSF-assisted based on left atrium (LA) segmentation dataset.
更多查看译文
关键词
Medical image segmentation,Learning with noisy labels,Region-Scalable Fitting,Active contours
AI 理解论文
溯源树
样例
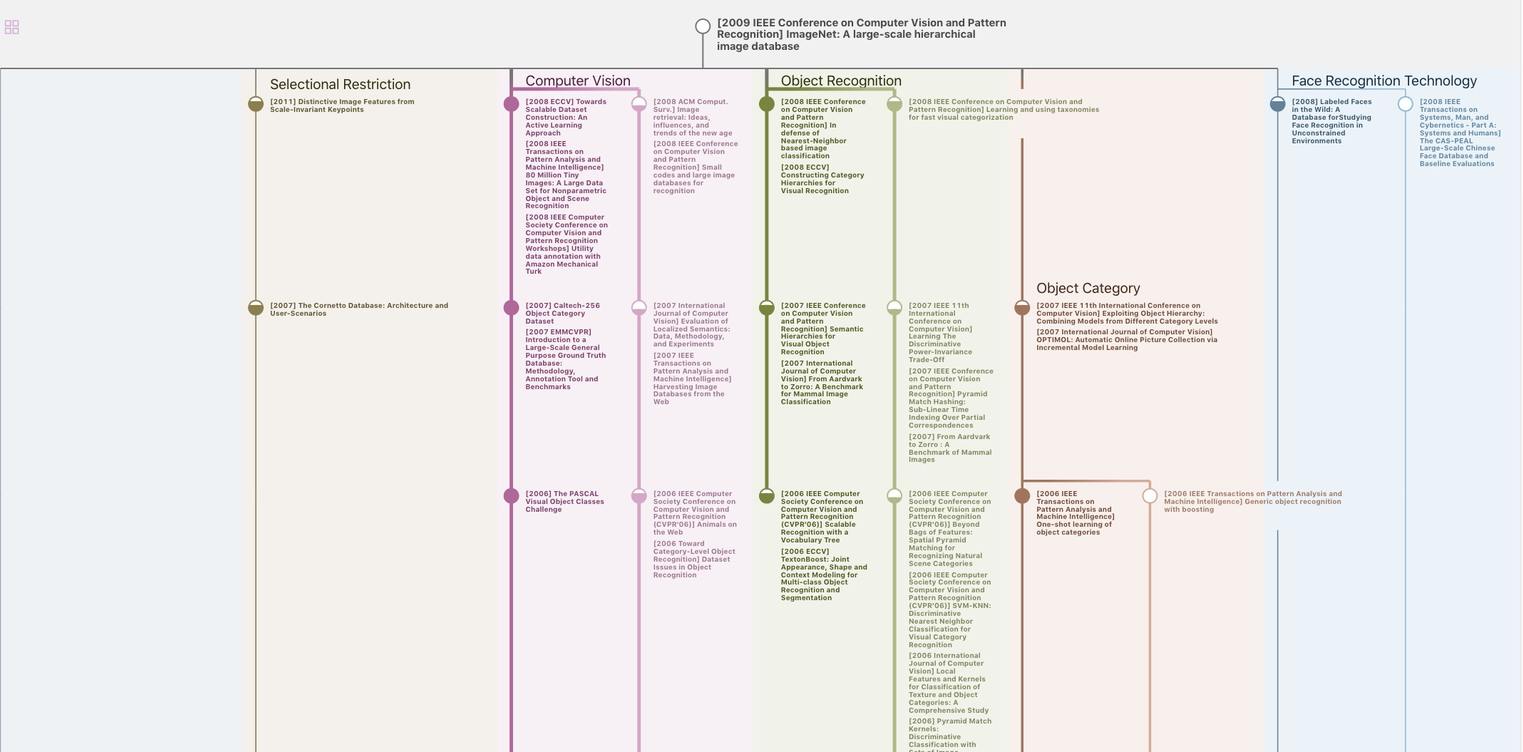
生成溯源树,研究论文发展脉络
Chat Paper
正在生成论文摘要