Multifidelity deep operator networks for data-driven and physics-informed problems
Journal of Computational Physics(2023)
Abstract
Operator learning for complex nonlinear systems is increasingly common in modeling multi-physics and multi-scale systems. However, training such high-dimensional operators requires a large amount of expensive, high-fidelity data, either from experiments or simulations. In this work, we present a composite Deep Operator Network (DeepONet) for learning using two datasets with different levels of fidelity to accurately learn complex operators when sufficient high-fidelity data is not available. Additionally, we demonstrate that the presence of low-fidelity data can improve the predictions of physics-informed learning with DeepONets. We demonstrate the new multi-fidelity training in diverse examples, including modeling of the ice-sheet dynamics of the Humboldt glacier, Greenland, using two different fidelity models and also using the same physical model at two different resolutions.
MoreTranslated text
Key words
Neural operator,Multifidelity,Operator learning,Physics-informed machine learning,Ice-sheet dynamics
AI Read Science
Must-Reading Tree
Example
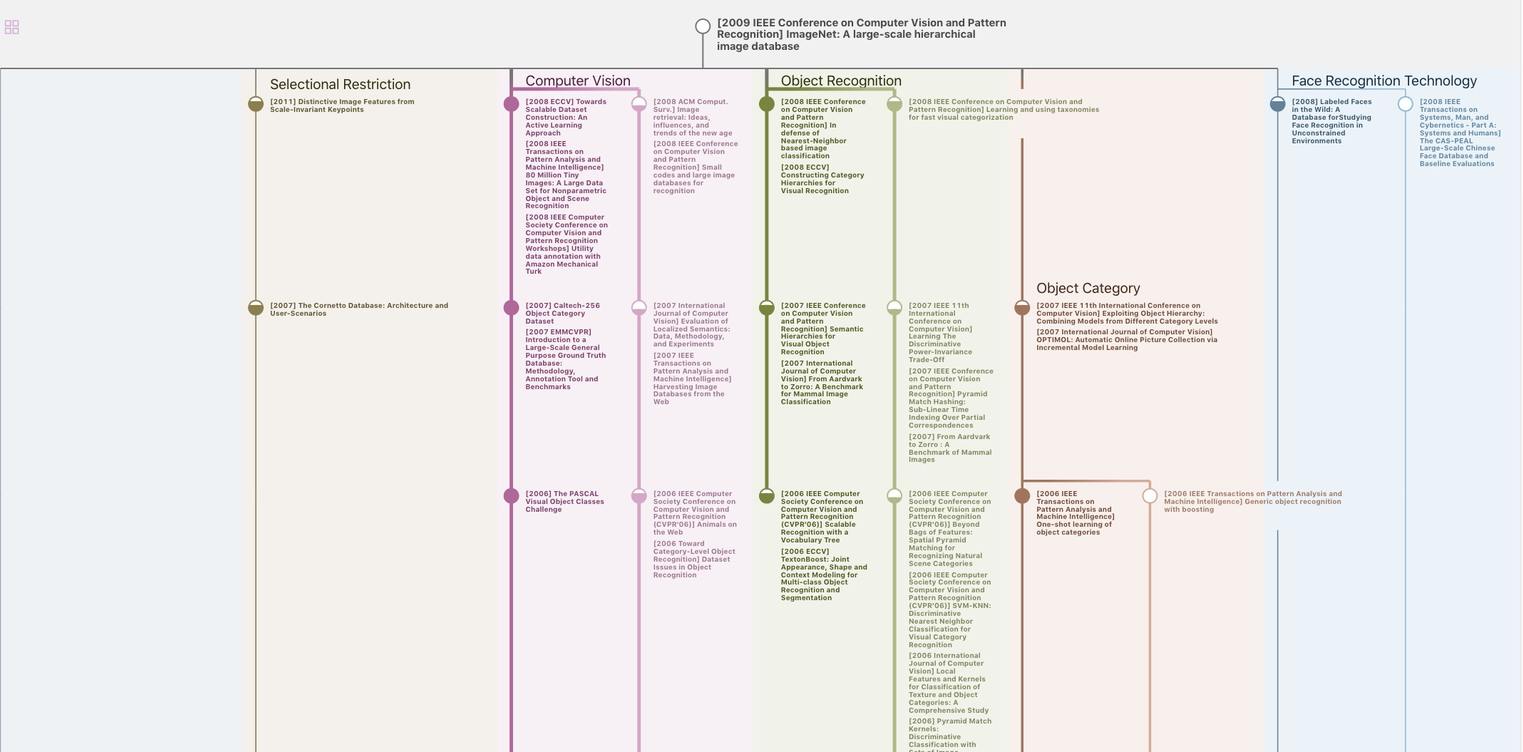
Generate MRT to find the research sequence of this paper
Chat Paper
Summary is being generated by the instructions you defined