Full-Reference Image Quality Assessment via Low-Level and High-Level Feature Fusion
INTERNATIONAL JOURNAL OF PATTERN RECOGNITION AND ARTIFICIAL INTELLIGENCE(2023)
摘要
We propose a full-reference image quality assessment (FR-IQA) method by incorporating low-level and high-level image features. First, in contrast to the preexisting deep IQA methods, which only use the features extracted by the deep network, we not only use the image gradient to replace the low-level features in the first two stages of the deep network, but also combine them with the middle-stage features of the deep network to construct the new low-level features. The deep features of shallow layers contain some unwanted noise and further result in a decline in IQA performance. Second, we combine the global features extracted by the self-attention-based model with the semantic features extracted by the convolutional neural network to form the high-level features. Instead of directly using the self-attention-based model trained on the classification task, we first train a no-reference (NR) IQA regression model on a larger dataset and then use the global features of this NR-IQA model. The self-attention-based model can capture the internal connections of the image and is more effective in extracting global information due to its larger perceptual field. In the final pooling stage, we combine the average pooling and the standard deviation pooling to obtain the dispersion and concentration of the similarity maps for a more comprehensive description of quality. Experiments show that our FR-IQA method is able to obtain competitive results on three standard IQA datasets.
更多查看译文
关键词
Full-reference (FR),image quality assessment (IQA),low-level feature,high-level feature
AI 理解论文
溯源树
样例
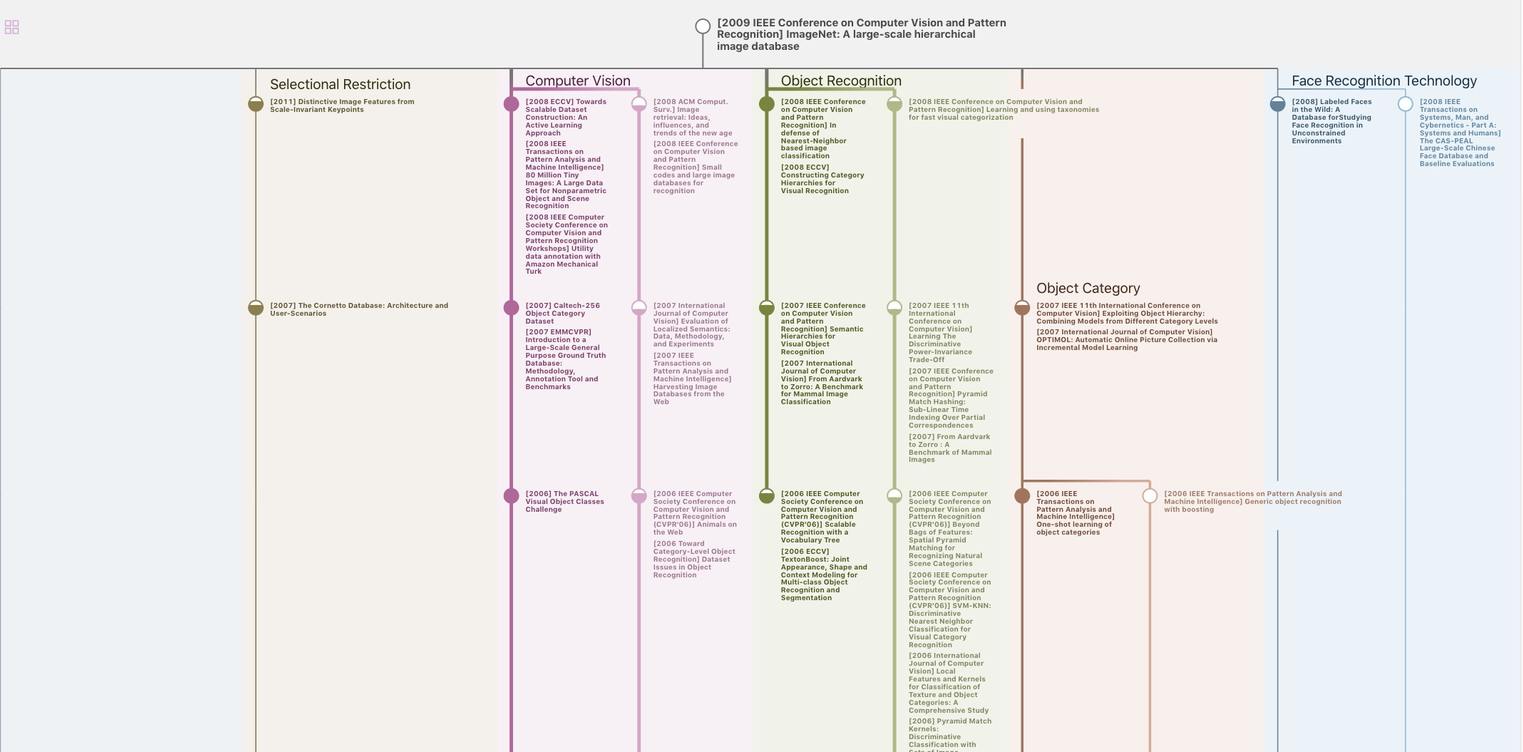
生成溯源树,研究论文发展脉络
Chat Paper
正在生成论文摘要