Short-term urban rail transit passenger flow forecasting based on fusion model methods using univariate time series
Appl. Soft Comput.(2023)
摘要
Global urbanization has made the urban rail transit system an essential service for a growing population. To help urban rail transit stations design optimal operational plans, previous studies have devoted extensive efforts to passenger flow forecasting, especially short-term predictions. By considering the complex pattern of passenger flow, previous research investigated the feasibility of machine learning (ML) methods on different data features and found the limited application of a single ML method. Based on the dynamic historical passenger flow data at an urban rail station, this study proposes an ML-fusion strategy to enhance prediction accuracy, including data aggregation, time series forecasting model selection, and fusion model strategy. First, this study aggregates the data into working days, weekends, and hourly time series for single model development. Based on the predictive performance of single model development, this study selects XGBoost, AdaBoost, and LightGBM from the widely used ML-method pool. To overcome prediction errors caused by the discrepancy between characteristics of passenger flow and single prediction models, the proposed ML-fusion model combines single forecasting models with dynamically predicted passenger flow to enhance the accuracy and efficiency of the prediction. Based on the experimental results, the mean absolute error is 1.54, and the regression coefficient is 0.99, which is in close agreement with unity, which validates that the proposed ML-fusion method has displayed superiority over all other single models tested both in accuracy and stability.(c) 2023 Elsevier B.V. All rights reserved.
更多查看译文
关键词
Short-term passenger flow forecast,XGBoost algorithm,LightGBM algorithm,AdaBoost algorithm,Fusion model strategy
AI 理解论文
溯源树
样例
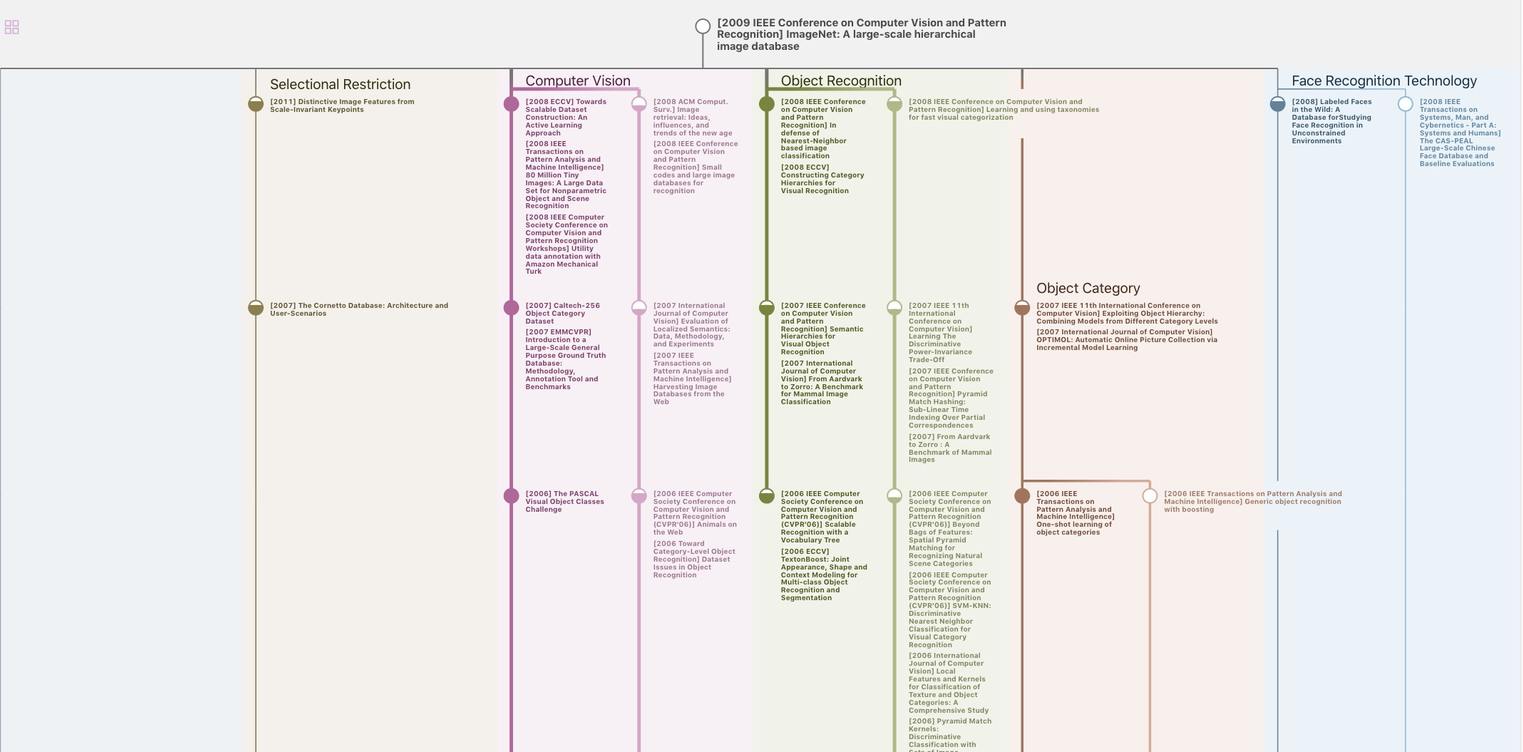
生成溯源树,研究论文发展脉络
Chat Paper
正在生成论文摘要