Robust Development of Active Learning-Based Surrogates for Induction Motor
IEEE TRANSACTIONS ON MAGNETICS(2024)
摘要
A robust open-source cloud-based workflow is developed for finite element (FE) data generation for active learning (AL)-based surrogate modeling. Special attention is paid to making the FE solution procedure as robust and fast as possible without human intervention by, e.g., implementing special convergence criteria, reliable parallel computation, and variable timestep length. In AL, a surrogate model automatically improves itself by iteratively querying more FE data. Using AL and large datasets generated with parallelized cloud FE simulations, we develop a surrogate model to rapidly predict induction machine steady-state torque, torque ripple, total losses, and current harmonic distortion, as a function of motor frequency, voltage, and slip. Results show that AL performs better than grid sampling and on average works as well as random sampling, but with some outputs, the results vary less with AL. In addition, accurate ripple estimation requires a much larger training dataset than the other variables.
更多查看译文
关键词
Cloud computing,data-driven modeling,finite element (FE) analysis,induction motors,machine learning (ML)
AI 理解论文
溯源树
样例
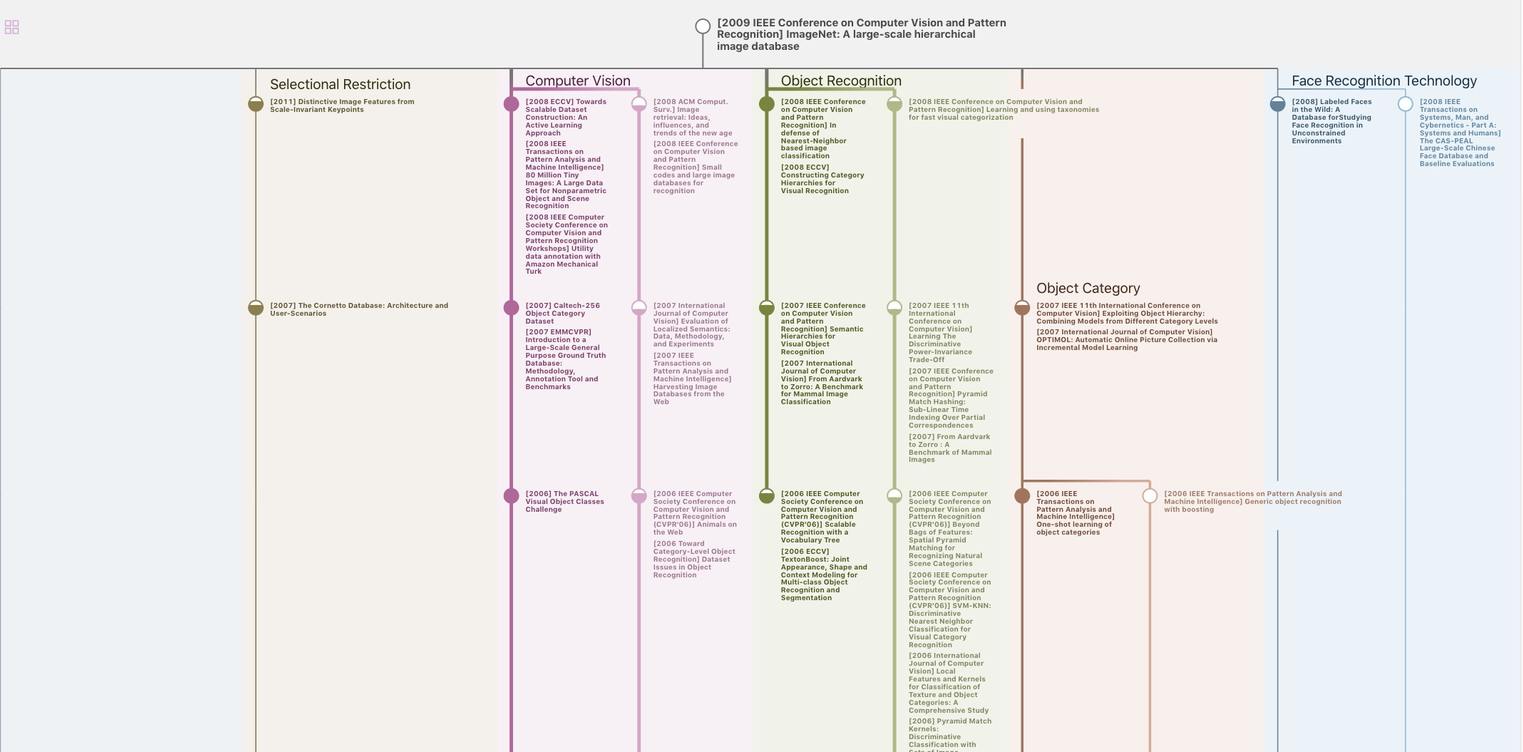
生成溯源树,研究论文发展脉络
Chat Paper
正在生成论文摘要