Long-Term Trajectory Prediction of Hypersonic Glide Vehicle Based on Physics-Informed Transformer
IEEE TRANSACTIONS ON AEROSPACE AND ELECTRONIC SYSTEMS(2023)
摘要
The long-term prediction of hypersonic glide vehicle (HGV) trajectory based on radar tracking data still faces challenges. Existing approaches are hard to provide satisfactory performances in long-term HGV trajectory prediction tasks due to insufficient long-range dependency extraction ability and cumulative errors. To address the above issues, a trajectory prediction model physics-informed Transformer (PIT) is proposed in this work. The top$\tau$-mean attention mechanism and the generative decoder are designed in this model to extract the trajectories' long-range dependency and reduce the cumulative errors. Furthermore, the physical knowledge is applied at both the input and output ends of the model, which further improves the model's prediction accuracy even in the case of missing a small amount of radar tracking data. A simulated HGV trajectory dataset is established based on the dynamic equations for the PIT trajectory prediction model. The experimental results show that the PIT performs better than other state-of-the-art deep neural network models. The additional experiments on a small-scale dataset and datasets with missing tracking data further verify the excellent performance and robustness of the PIT in these cases.
更多查看译文
关键词
Trajectory,Predictive models,Neural networks,Transformers,Feature extraction,Mathematical models,Data models,Hypersonic glide vehicle (HGV),physics-informed,trajectory prediction
AI 理解论文
溯源树
样例
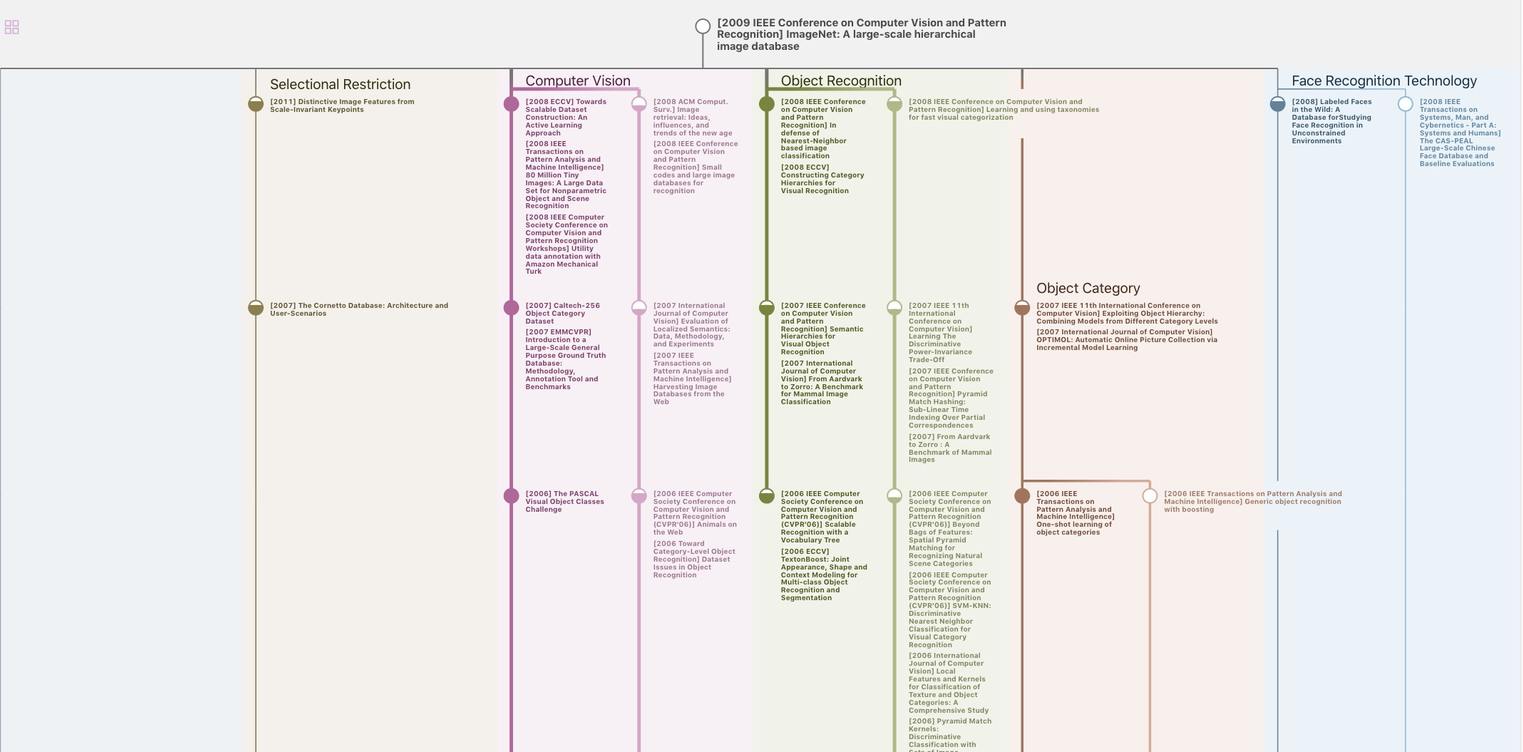
生成溯源树,研究论文发展脉络
Chat Paper
正在生成论文摘要