The effect of simulation variety on a deep learning-based military vehicle detector
Artificial Intelligence for Security and Defence Applications(2023)
Abstract
Deep learning has emerged as a powerful tool for image analysis in various fields including the military domain. It has the potential to automate and enhance tasks such as object detection, classification, and tracking. Training images for development of such models are typically scarce, due to the restricted nature of this type of data. Consequently, researchers have focused on using synthetic data for model development, since simulated images are fast to generate and can, in theory, make up a large and diverse data set. When using simulated training data it is important to consider the variety needed to bridge the gap between simulated and real data. So far it is not fully understood what variations are important and how much variation is needed. In this study, we investigate the effect of simulation variety. We do so for the development of a deep learning-based military vehicle detector that is evaluated on real-world images of military vehicles. To construct the synthetic training data, 3D models of the vehicles are placed in front of diverse background scenes. We experiment with the number of images, background scene variations, 3D model variations, model textures, camera-object distance, and various object rotations. The insight that we gain can be used to prioritize future efforts towards creating synthetic data for deep learning-based object detection models.
MoreTranslated text
Key words
Synthetic data, deep learning, object detection, simulation variation
AI Read Science
Must-Reading Tree
Example
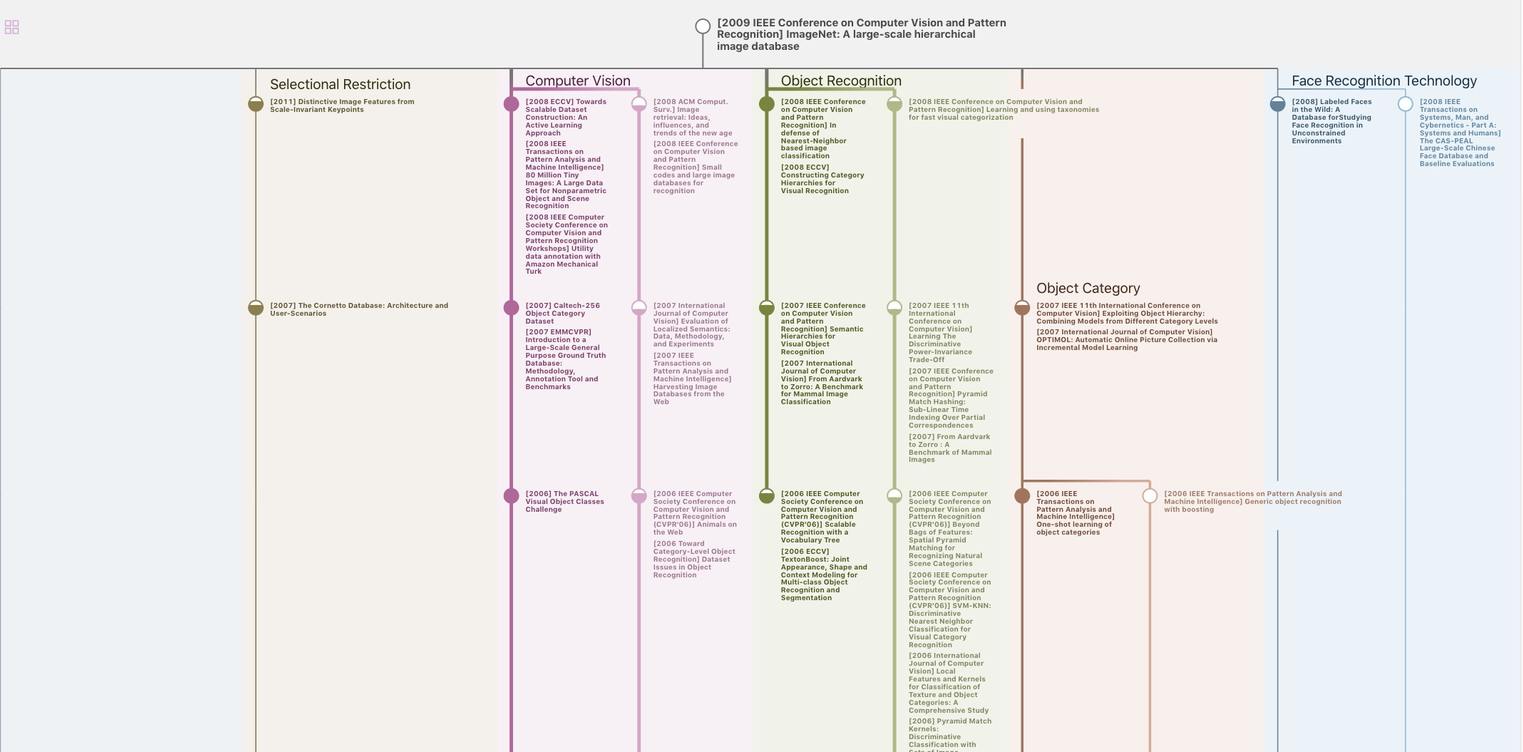
Generate MRT to find the research sequence of this paper
Chat Paper
Summary is being generated by the instructions you defined