Nonlinear Manifold Learning and Model Reduction for Transonic Flows
AIAA JOURNAL(2023)
Abstract
It is aspirational to construct a nonlinear reduced-order model (ROM) with the ability to predict computational fluid dynamics (CFD) solutions accurately and efficiently. One major challenge is that the nonlinearity cannot be captured adequately by interpolation algorithm in low-dimensional space. To preserve the nonlinearity of CFD solutions for transonic flows, a new ROM is presented by integrating manifold learning into a constrained optimization, whereby a neighborhood preserving mapping is constructed by locally linear embedding (LLE) algorithm. Reconstruction errors are minimized in LLE by solving a least square problem subject to weight constraints. A loss function is proposed in the constrained optimization to preserve the geometric properties between high-dimensional space and low-dimensional manifolds. The proposed ROM is validated to predict nonlinear transonic flows over RAE 2822 airfoil and undeflected NASA Common Research Model with aspect ratio 9, in which nonlinearities are induced by shock waves. All results confirm that the ROM replicates CFD solutions accurately at fraction of the cost of CFD calculation or the full-order modeling.
MoreTranslated text
Key words
Uncertainty Quantification,Proper Orthogonal Decomposition,Undeflected Common Research Model,Common Research Model,Shock Waves,Reynolds Averaged Navier Stokes,Multidisciplinary Design and Optimization
AI Read Science
Must-Reading Tree
Example
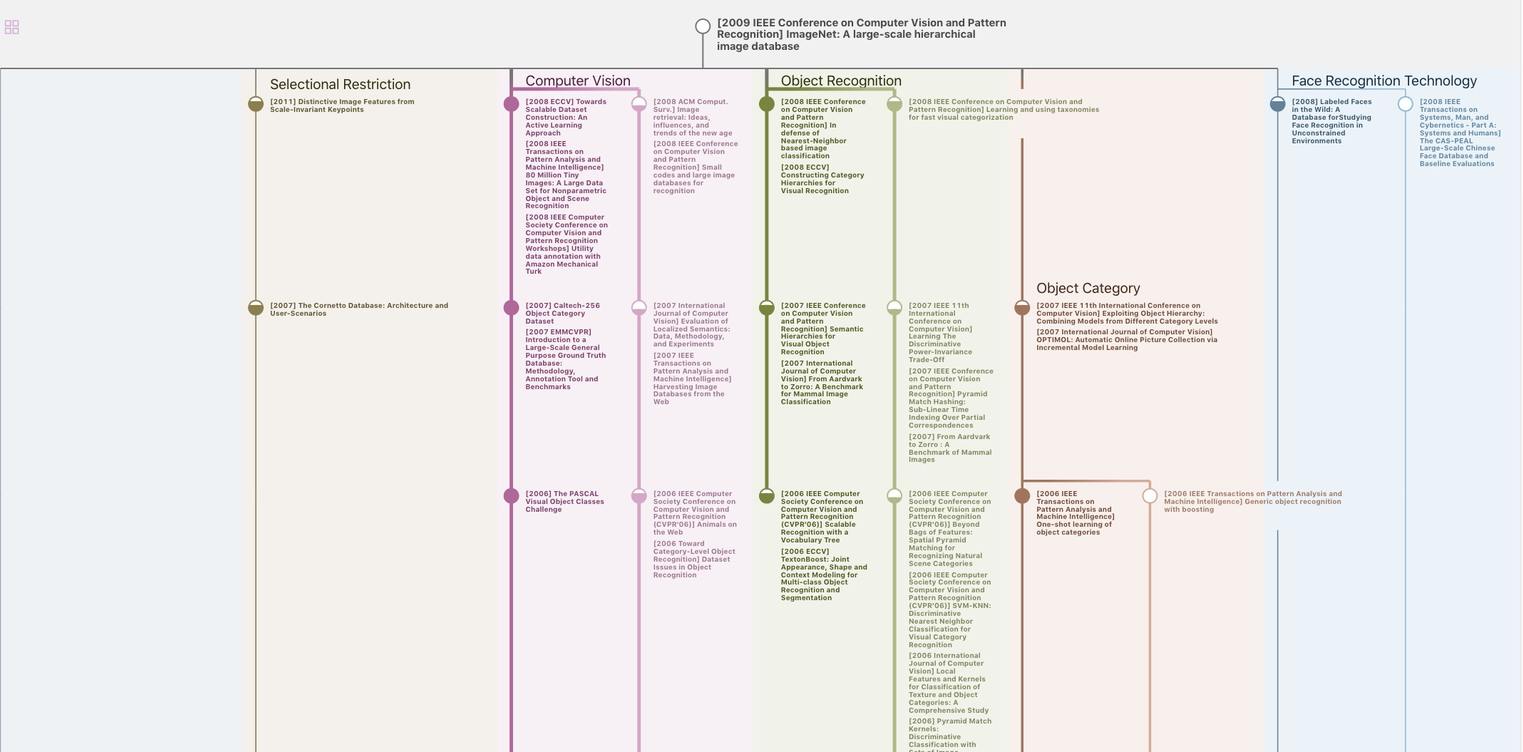
Generate MRT to find the research sequence of this paper
Chat Paper
Summary is being generated by the instructions you defined