Proximal Policy Optimization Based USV Navigation Under Auxiliary Soft Policy Constraints
2024 IEEE 2nd International Conference on Control, Electronics and Computer Technology (ICCECT)(2024)
摘要
Due to their high flexibility and adaptability, un-manned surface vehicles (USVs) have become a crucial platform for the cost-effective expansion of marine tasks. In this paper, a deep reinforcement learning based USV navigation algorithm is proposed for achieving an end-to-end mapping between USV environmental perception and smooth actions. In our approach, we initially model the USV navigation task using the proximal policy optimization algorithm based on the actor-critic framework and design the USV's action control and state space, incorporating a well-defined single-step reward function to encourage rapid convergence of USV policies. Furthermore, to address potential issues of severe trajectory oscillations and non-smooth actions during USV navigation, we introduce a constraint term of auxiliary soft policy for continuous action changes of the USV in the actor network's objective function, thereby enhancing the stability of the USV navigation process. Finally, we validate the algorithm using Unity3D to design a USV navigation scenario. By comparing it with benchmark algorithms, our proposed algorithm not only accomplishes the USV navigation task but also exhibits higher overall smoothness in the navigation process.
更多查看译文
关键词
Unmanned surface vehicles,Autonomous navigation,Deep reinforcement learning,Smooth action
AI 理解论文
溯源树
样例
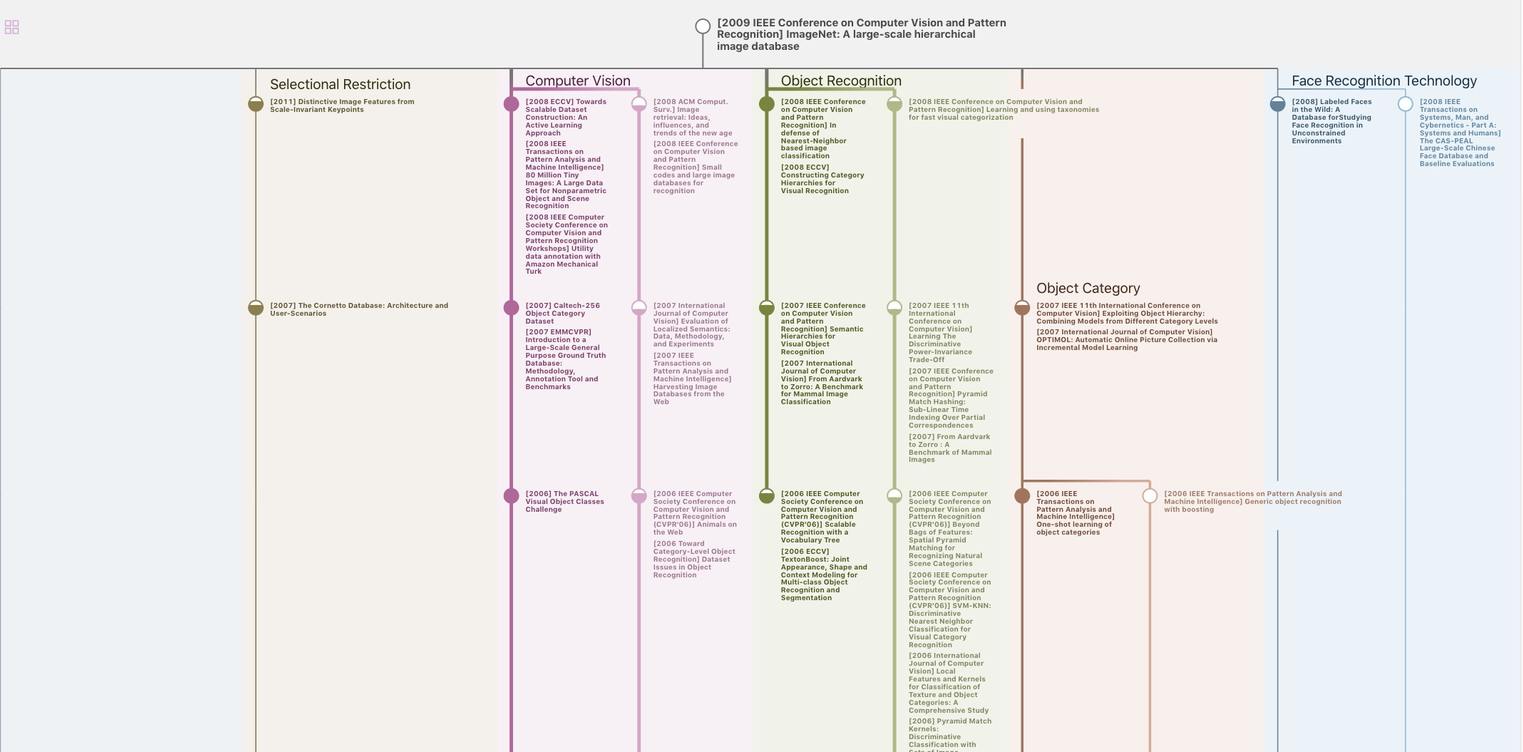
生成溯源树,研究论文发展脉络
Chat Paper
正在生成论文摘要