An Empirical Evaluation of the Data Leakage in Federated Graph Learning
IEEE TRANSACTIONS ON NETWORK SCIENCE AND ENGINEERING(2024)
摘要
Inspired by the successful application of dealing with graph-structured data, graph neural networks (GNNs) have captured significant research attention. Considering the privacy protection of the locally collected user data, federated graph learning (FGL) which shares graph embeddings or local models' gradient is proposed to decentralize GNNs training. While sharing the embedding or gradient in FGL is intriguing, the associated privacy risks are somehow unexplored. For instance, private data like graph structure and attributes could still leak out somehow. In this article, we investigate the problem of stealing graph data, including structure and attributes, in both vertical and horizontal federated learning (VFGL and HFGL). Specifically, we propose four types of inference attack: (i) Link inference attack (LIA) and (ii) Attribute inference attack (AIA), (iii) Graph reconstruction attack (GRA) and (iv) Graph feature attack (GFA). The first two methods are designed for VFGL, while the latter two attack methods are intended for HFGL. To the best of our knowledge, this is the first comprehensive study of data security in both VFGL and HFGL. Extensive experiments on 13 datasets and 6 models are carried out and the superior attack performance shows the effectiveness of the proposed methods, revealing the risk of FGL.
更多查看译文
关键词
Servers,Data privacy,Training,Data models,Privacy,Biological system modeling,Graph neural networks,Federated graph learning,graph reconstruction attack,link inference attack,defense
AI 理解论文
溯源树
样例
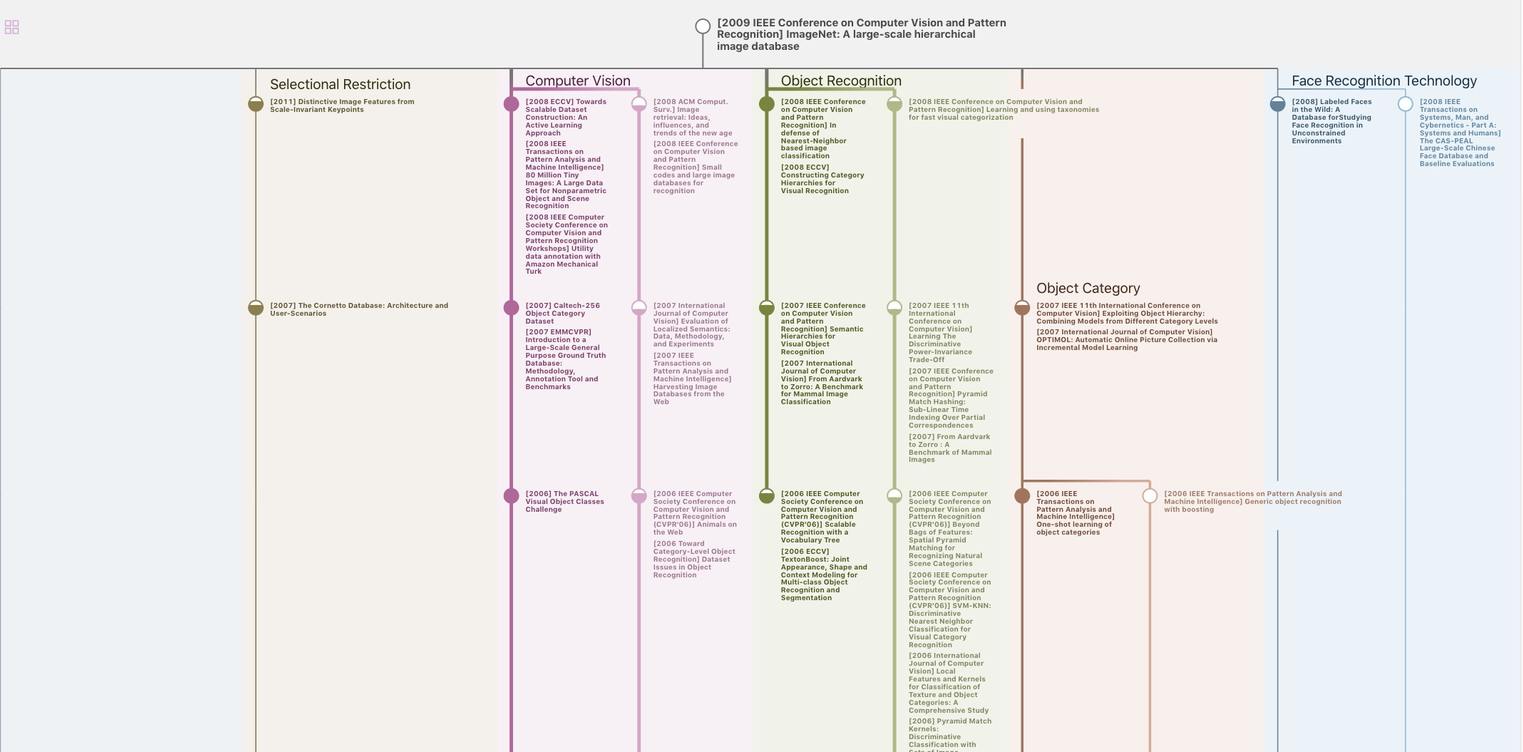
生成溯源树,研究论文发展脉络
Chat Paper
正在生成论文摘要