Self-Supervised Adversarial Variational Learning
PATTERN RECOGNITION(2024)
摘要
A natural approach for representation learning is to combine the inference mechanisms of VAEs and the generative abilities of GANs, within a new model, namely VAEGAN. Most existing VAEGAN models would jointly train the generator and inference modules, which has limitations when learning representations generated by a pre-trained GAN model without data. In this paper, we develop a novel hybrid model, called the Self-Supervised Adversarial Variational Learning (SS-AVL) which introduces a two-step optimization procedure training separately the generator and the inference model. The primary advantage of SS-AVL over existing VAEGAN models is that SS-AVL optimizes the inference models in a self-supervised learning manner where the samples used for training the inference models are drawn from the generator distribution instead of using real samples. This can allow SS-AVL to learn representations from arbitrary GAN models without using real data. Additionally, we employ information maximization into the context of increasing the maximum likelihood, which encourages SS-AVL to learn meaningful latent representations. We perform extensive experiments to demonstrate the effectiveness of the proposed SS-AVL model.
更多查看译文
关键词
Self-supervised learning,Variational Autoencoders (VAE),Generative Adversarial Nets (GAN),Representation learning,Mutual information
AI 理解论文
溯源树
样例
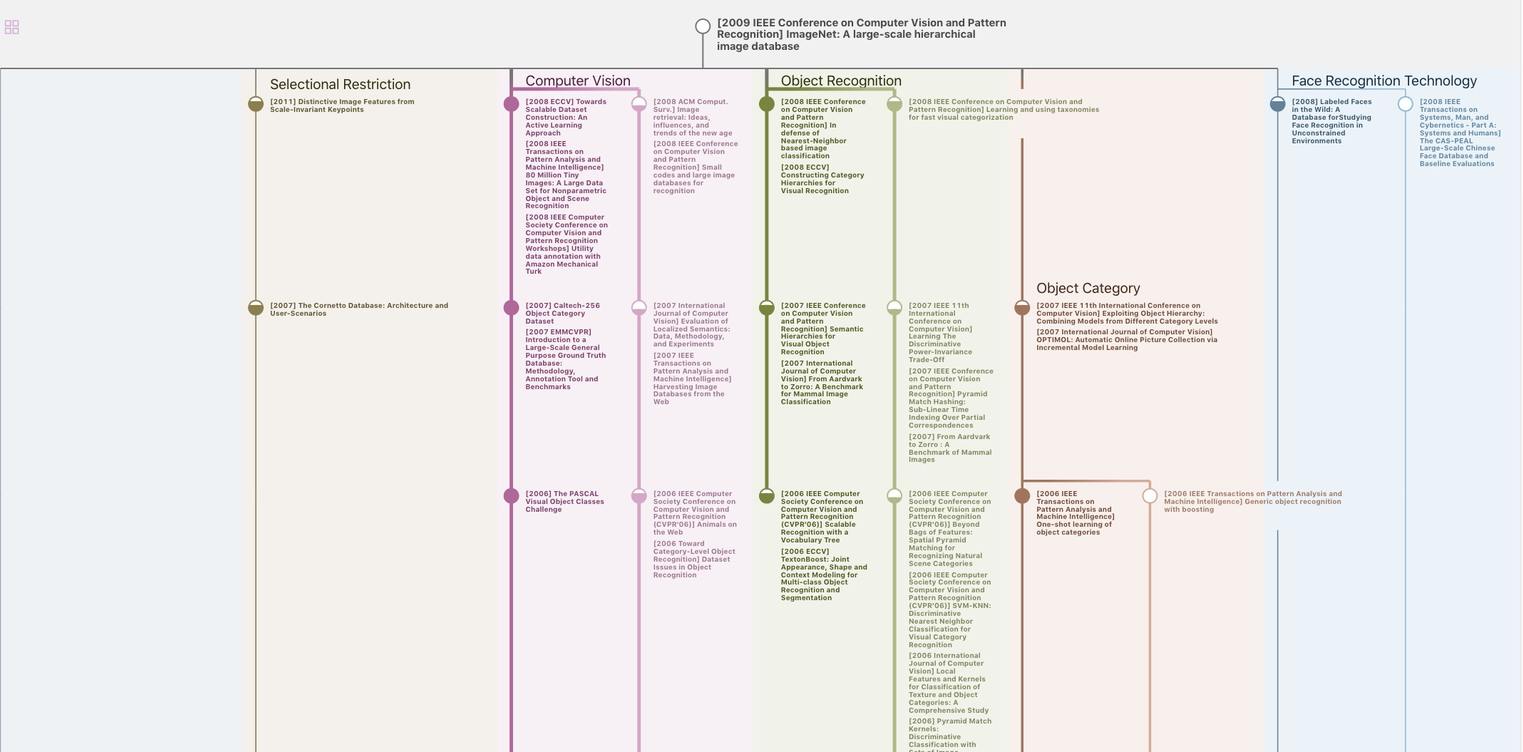
生成溯源树,研究论文发展脉络
Chat Paper
正在生成论文摘要