Graph-Based Semantic Embedding Refinement for Zero-Shot Remote Sensing Image Scene Classification
IEEE TRANSACTIONS ON AEROSPACE AND ELECTRONIC SYSTEMS(2024)
摘要
Zero-shot remote sensing image scene classification (ZS-RSISC) aims to identify remote sensing (RS) image scenes of unseen classes whose samples are unavailable in the training stage. To transfer knowledge from seen RS classes to unseen RS classes, existing methods either rely on laborious manual labeling to learn semantic features or directly use the word embeddings learned based on the general corpus and independently of zero-shot models. They ignore the complex interclass correlation information, which plays a vital role in communicating seen with unseen classes. Besides, current studies in ZS-RSISC impose the same penalty to equally constrain each class for the interclass separation and intraclass compactness, which results in unclear classification boundaries. In this article, we tackle ZS-RSISC via graph-based semantic embedding refinement (GSER) in an end-to-end manner. We propose semantic graph convolutional networks (S-GCNs) to explore the correlation structure among classes in a unified framework. The semantic graph embeddings are further refined by the learning of the semantic-guided class patterns and component patterns. Specifically, we propose adaptive additive separation (AAS) loss to adaptively adjust the appropriate penalty for each class and explicitly promote intraclass compactness and interclass separation. Further, instance-level alignment and class-level alignment are proposed to enhance the discriminative ability of the semantic-guided class patterns. To alleviate model bias toward seen classes, semantic-guided component patterns shared by seen and unseen classes are exploited via feature reconstruction. Extensive experiments of both the zero-shot and generalized zero-shot settings demonstrate the effectiveness of our proposed GSER.
更多查看译文
关键词
Semantics,Visualization,Feature extraction,Convolutional neural networks,Training,Task analysis,Remote sensing
AI 理解论文
溯源树
样例
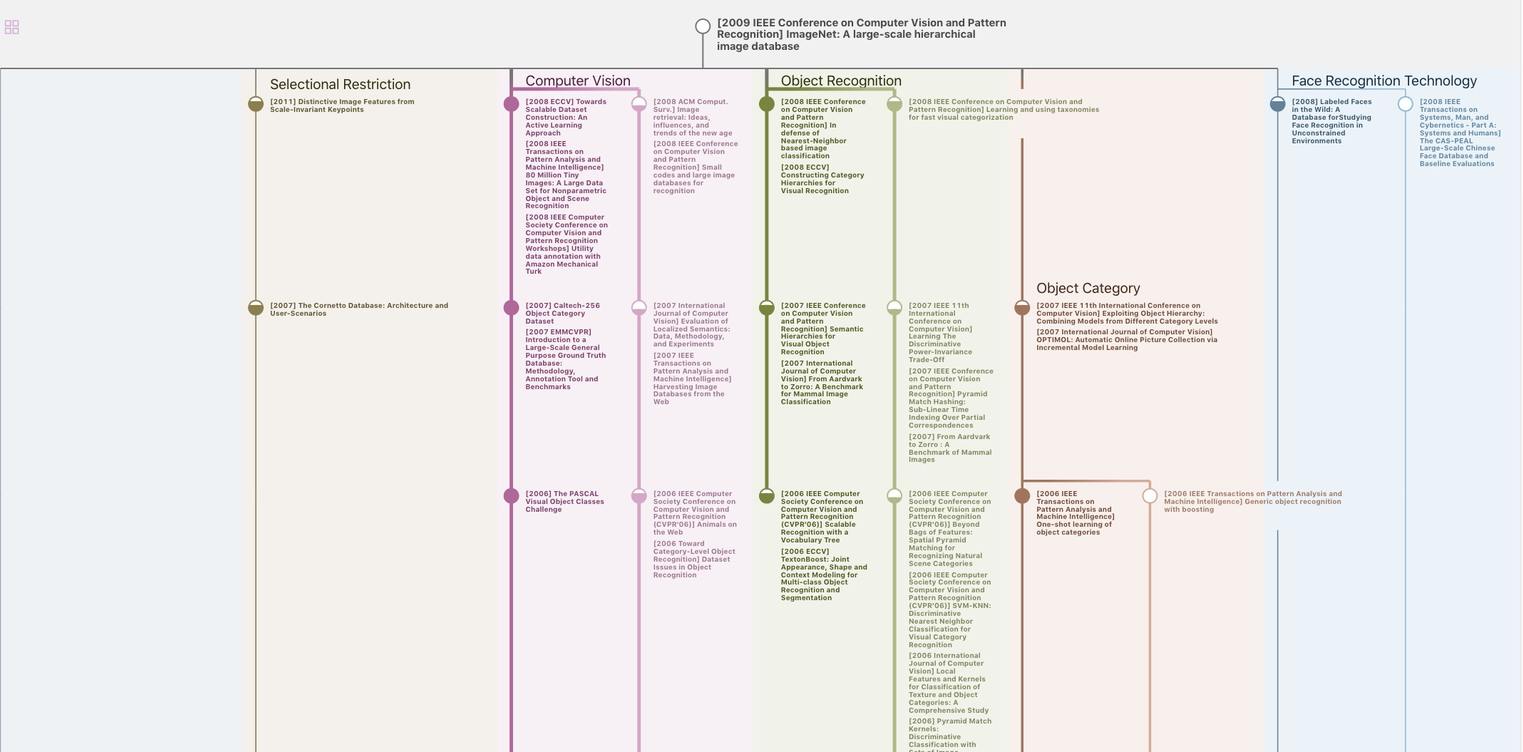
生成溯源树,研究论文发展脉络
Chat Paper
正在生成论文摘要