New Data Novelty Check and Distributed Learning for IoT Data Anomaly Detection
Proceedings of Eighth International Congress on Information and Communication Technology Lecture Notes in Networks and Systems(2023)
摘要
The Internet of things (IoT) is set to fundamentally change our daily lives with massive numbers of connected devices, generating large volumes of data every second. Data acquisition and data correctness verification for different processes have become of increasing concern. IoT data anomaly detection performance relies on accuracy and response time and both can be enhanced with a distributed learning and adaptive “online” update of the model. Retraining the model only when the distribution of the new collected data differs from the distribution of the data used to learn the model greatly streamlines the computational resources of the distributed learning infrastructure. It also improves learning data accuracy as the model is updated regularly rather than waiting for the retraining period, which is usually a fixed value and is set up manually. It’s a well-established fact that a machine learning model accurately predicts if the distribution of new data, on which predictions are made, is similar to the distribution of the model training data. However, over time, the new data retrieved may hold additional information that was not initially identified by the model when it was trained. Therefore, model deployment should not be a one-time exercise, but rather a continuous process. In this article, we propose a scalable, robust, and sustainable IoT data anomaly detection framework based on distributed learning and an efficient online model update. First, we investigate the distributed learning of different anomaly detection models, namely RNN, LSTM, and k-means clustering. These models are trained for anomaly detection tasks on two different IoT data use cases: ECG sensor data for the health industry and connected cars for the automotive industry. Then, we study the significance of identifying new data at an early stage to determine when to retrain the model for the purpose of improving anomaly detection accuracy. The research thus focuses on the ideal moment to retrain a model based on the data novelty detection in order to establish an efficient automated retraining module.
更多查看译文
关键词
detection
AI 理解论文
溯源树
样例
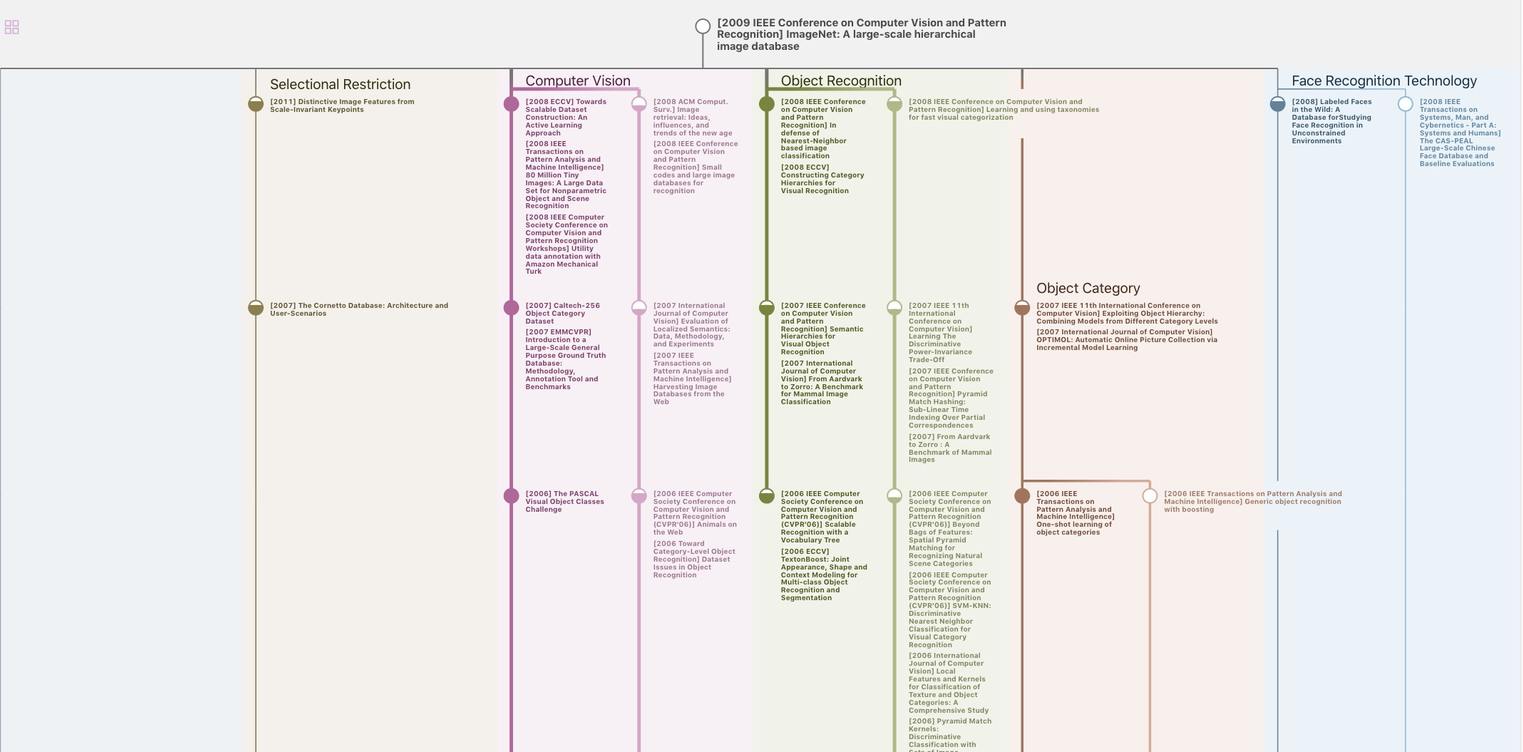
生成溯源树,研究论文发展脉络
Chat Paper
正在生成论文摘要