Local aggressive and physically realizable adversarial attacks on 3D point cloud
COMPUTERS & SECURITY(2024)
摘要
Deep learning models used to process 3D point cloud data exhibit a vulnerability to adversarial examples, which can result in potential misclassification. The research community has pivoted towards applying traditional optimization methods to generate adversarial examples specifically for the point cloud domain. However, these traditional optimization-based adversarial approaches come with their own set of limitations, including inconsistent point importance, non -optimal multi-label optimization, inflexible adversarial and perceptibility losses, and difficulty in constructing perceivable adversarial examples in physical environments. To surmount these challenges, we have introduced two novel techniques: a Local Aggressive Adversarial Attack (L3A) for simulated environments and a Normal Vector Metric Attack (NVMA) for physical environments. The L3A operates by enhancing the cost-effectiveness ratio between the attack and perceptibility aspects. This enhancement is realized through a combination of strategies, including local salient points, modeling example preference, and adjusting the goals for attack potency and indistinguishability. The NVMA generates physically printable adversarial point clouds by maintaining consistency in high-frequency regions between clean and adversarial examples. Our methods exhibit superior performance when tested against state -of -the -art point cloud deep learning models on benchmark datasets and physical models. This contribution enhances our understanding of adversarial attacks and defenses within the realm of 3D point cloud data. Our code is available at https:// github .com /Chenfeng1271 /L3A.
更多查看译文
关键词
Point clouds processing,Adversarial learning,Local adversarial attack,Physical attack,Deep learning
AI 理解论文
溯源树
样例
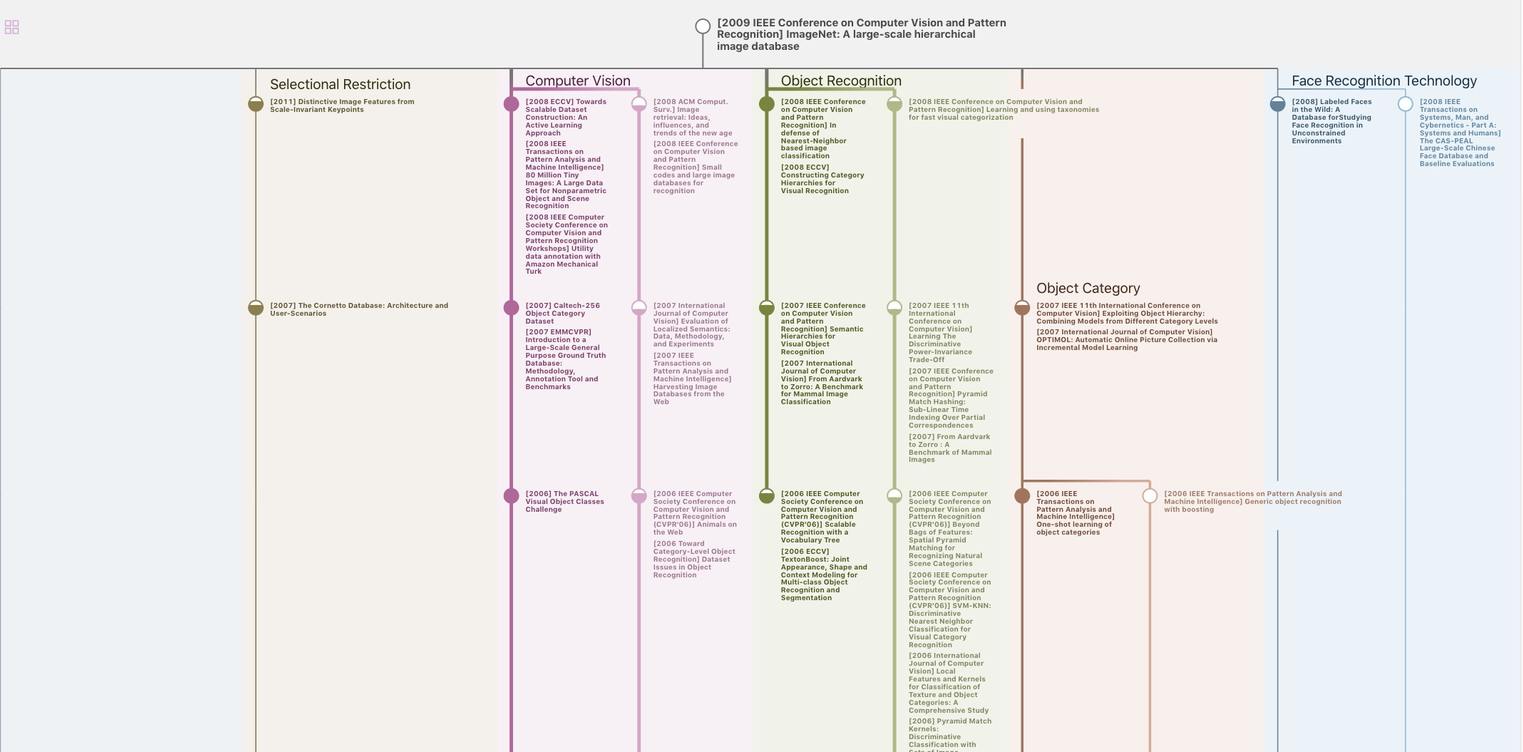
生成溯源树,研究论文发展脉络
Chat Paper
正在生成论文摘要