Condition monitoring for nuclear turbines with improved dynamic partial least squares and local information increment
ENGINEERING APPLICATIONS OF ARTIFICIAL INTELLIGENCE(2024)
摘要
Performing online condition monitoring for nuclear turbines in the rapidly changing environment is a challenging but imperative task to enhance the safety and reliability of nuclear power plants. Given the nonlinear and dynamic properties of nuclear turbine operation, this paper proposes an innovative method for condition monitoring. Specifically, the paper first redesigns time augmented matrices based on lagged data to reflect the process dynamics. Subsequently, a dynamic auto-regressive model, integrated with the variant of kernel partial least squares, is built between input and output variables, which represents auto-correlations and cross correlations of operation data simultaneously. The prediction of the model serves as the baseline for the monitoring indicator. Additionally, since the operation process involves variable working excitation and random noise, making static control limits insufficient to satisfy the requirements of condition monitoring, the proposed method utilizes a novel monitoring indicator based on local information increment. The indicator comprehensively incorporates the prediction value and past measurement for monitoring statistics and control limits. Finally, the proposed method is applied to a real nuclear turbine operation process, and the results are compared with three other methods to demonstrate its superiority.
更多查看译文
关键词
Nuclear turbine,Dynamic auto-regressive model,Kernel partial least squares,Local information increment,Condition monitoring,Quality-related detection
AI 理解论文
溯源树
样例
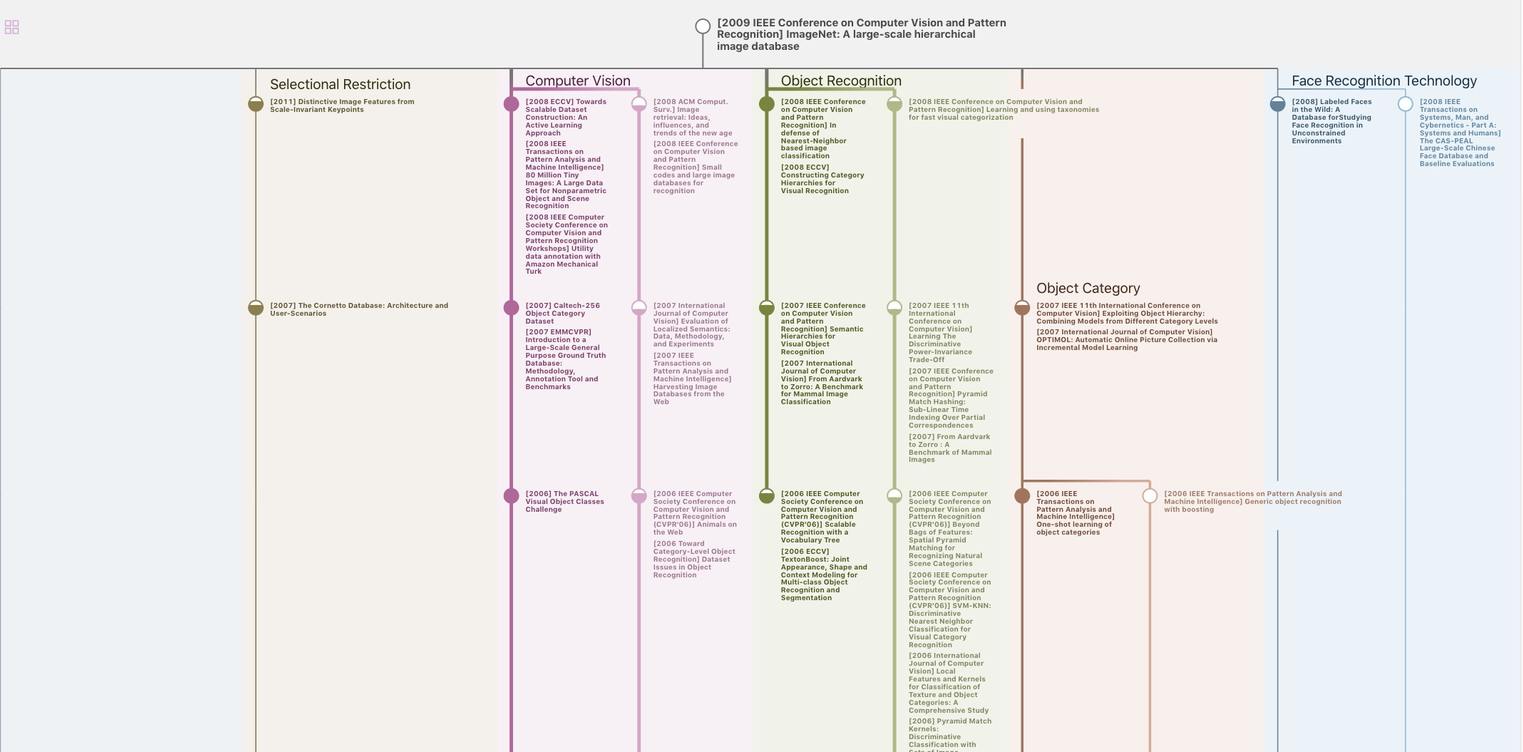
生成溯源树,研究论文发展脉络
Chat Paper
正在生成论文摘要