FCGCN: Feature Correlation Graph Convolution Network for Few-Shot Individual Identification
IEEE Transactions on Consumer Electronics(2023)
摘要
In the era of big data, Deep Learning (DL) technology has achieved breakthroughs in various tasks involving electromagnetic analysis and recognition signals. However, in practical application scenarios, intercepting and labeling electromagnetic signals is difficult, which is challenging to reach excellent performance for DL models as they require lots of samples. Therefore, in this paper, we study few-shot individual identification and propose a Feature Correlation Graph Convolution Network (FCGCN) method to solve this problem. Specifically, the proposed method includes graph structure mapping and identification classification. In more detail, the former is based on expert feature extraction and correlation coefficient calculation whereas the latter is based on the designed Graph Convolution Network (GCN) model. The experimental results, after applying the proposed method on the simulated 5G User Equipment (UE) signal dataset, show that the graph structure can fully represent the deviation between signals of different classes and the correlation within a single class. Moreover, this method reveals better performance in few-shot recognition than existing methods. When the number of samples in each category is 40, the average recognition accuracy of the different Signal-to-Noise-Ratios (SNRs) is 7% higher than that of the baseline model, and it gives better results under low SNR.
更多查看译文
关键词
Individual identification,few-shot recognition,graph convolution network,graph classification
AI 理解论文
溯源树
样例
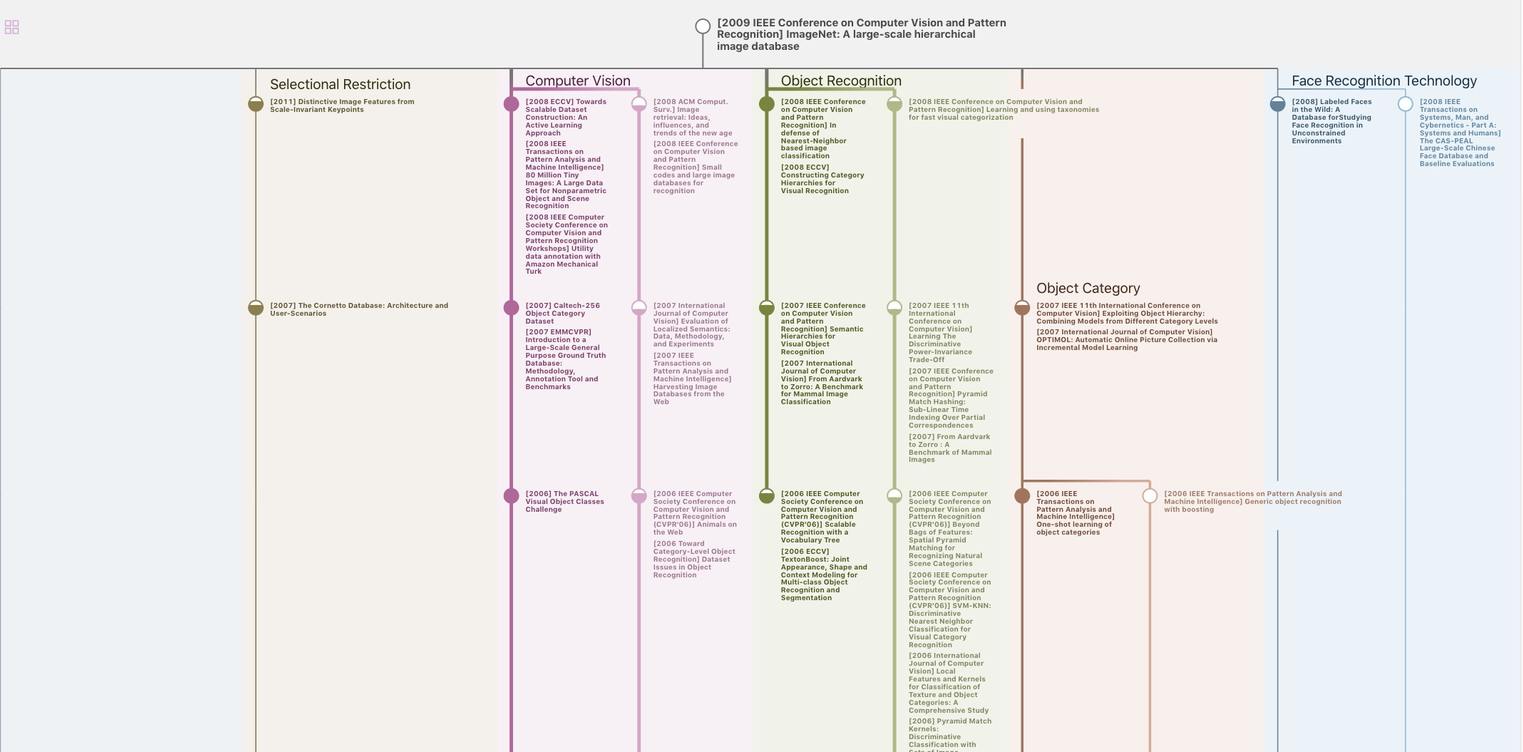
生成溯源树,研究论文发展脉络
Chat Paper
正在生成论文摘要