Column generation-based prototype learning for optimizing area under the receiver operating characteristic curve
EUROPEAN JOURNAL OF OPERATIONAL RESEARCH(2024)
摘要
The traditional classification algorithms focus on the maximization of classification accuracy which might lead to poor performance in practice by forcing classifiers to overfit to the majority class. In order to overcome this issue, various approaches focus on the optimization of alternative loss functions such as the Area Under the Curve (AUC). AUC is a Receiver Operating Characteristics (ROC) metric that has been widely used to measure classification performance, especially when there are class imbalances. In this work, we propose a column generation (CG)-based algorithm called Ranking-CG, which learns a model, similar to the popular Ranking SVM, through approximate maximization of the AUC. Unlike the Ranking SVM, our algorithm utilizes a column generation method that iteratively adds features to control the model complexity effectively working as an internal feature selection procedure. Our experiments show that column generation can be an important tool to prevent overfitting. We extend the Ranking-CG by proposing a prototype generation method, denoted by Ranking-CG Prototype, that constructs reference points by solving a non-linear optimization problem. Based on the extensive experiments conducted on 74 binary classification problems, the Ranking-CG Prototype yields the best average test AUC among all competing methods by using significantly few features than other benchmarks.
更多查看译文
关键词
AUC,Ranking,SVM,Column generation,Binary classification
AI 理解论文
溯源树
样例
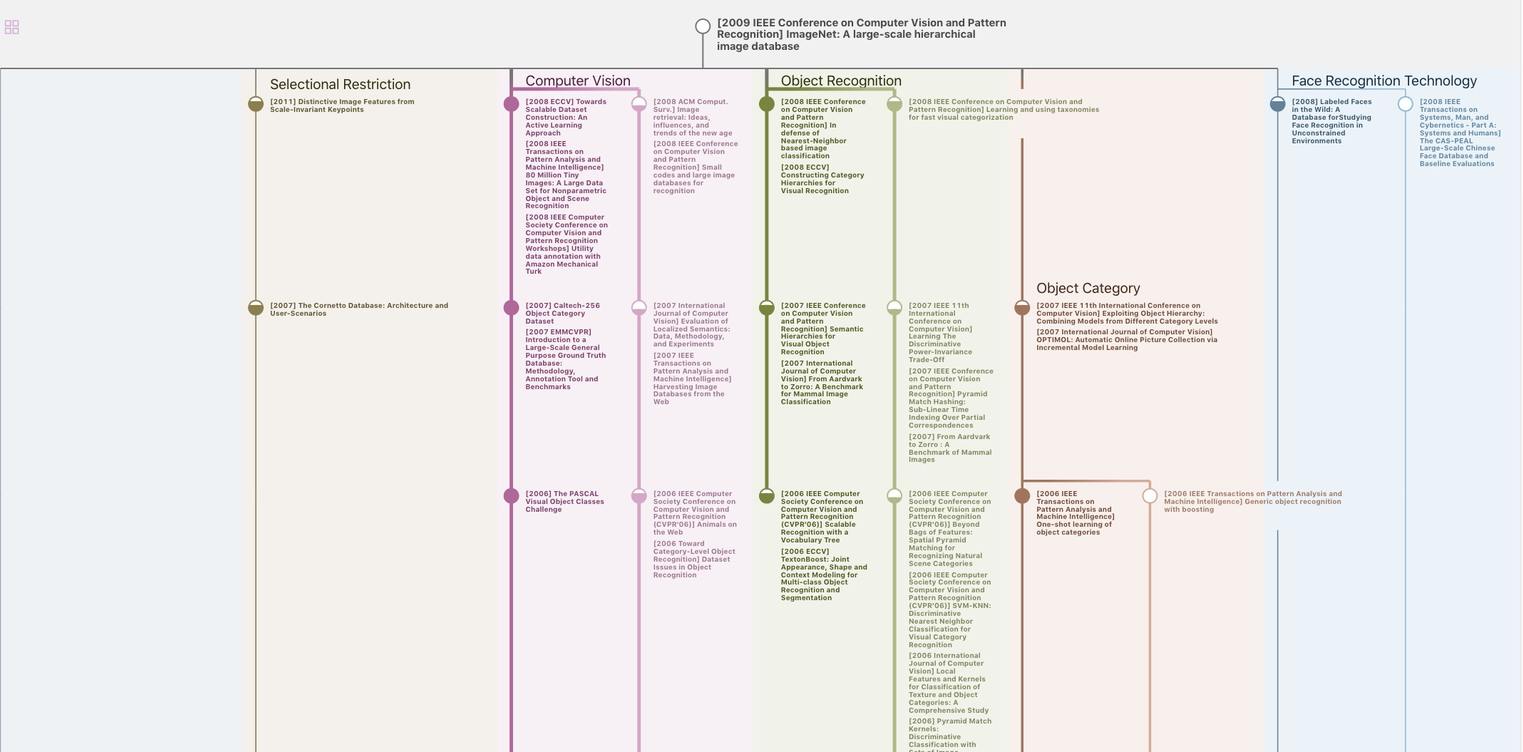
生成溯源树,研究论文发展脉络
Chat Paper
正在生成论文摘要