Exploiting sparsity and statistical dependence in multivariate data fusion: an application to misinformation detection for high-impact events
Machine Learning(2023)
摘要
With the evolution of social media, cyberspace has become the de-facto medium for users to communicate during high-impact events such as natural disasters, terrorist attacks, and periods of political unrest. However, during such high-impact events, misinformation can spread rapidly on social media, affecting decision-making and creating social unrest. Identifying the spread of misinformation during high-impact events is a significant data challenge, given the multi-modal data associated with social media posts. Advances in multi-modal learning have shown promise for detecting misinformation; however, key limitations still make this a significant challenge. These limitations include the explicit and efficient modeling of the underlying non-linear associations of multi-modal data geared at misinformation detection. This paper presents a novel avenue of work that demonstrates how to frame the problem of misinformation detection in social media using multi-modal latent variable modeling and presents two novel algorithms capable of modeling the underlying associations of multi-modal data. We demonstrate the effectiveness of the proposed algorithms using simulated data and study their performance in the context of misinformation detection using a popular multi-modal dataset that consists of tweets published during several high-impact events.
更多查看译文
关键词
Data fusion,Independent vector analysis,Multi-modal learning,Misinformation detection,Deep learning
AI 理解论文
溯源树
样例
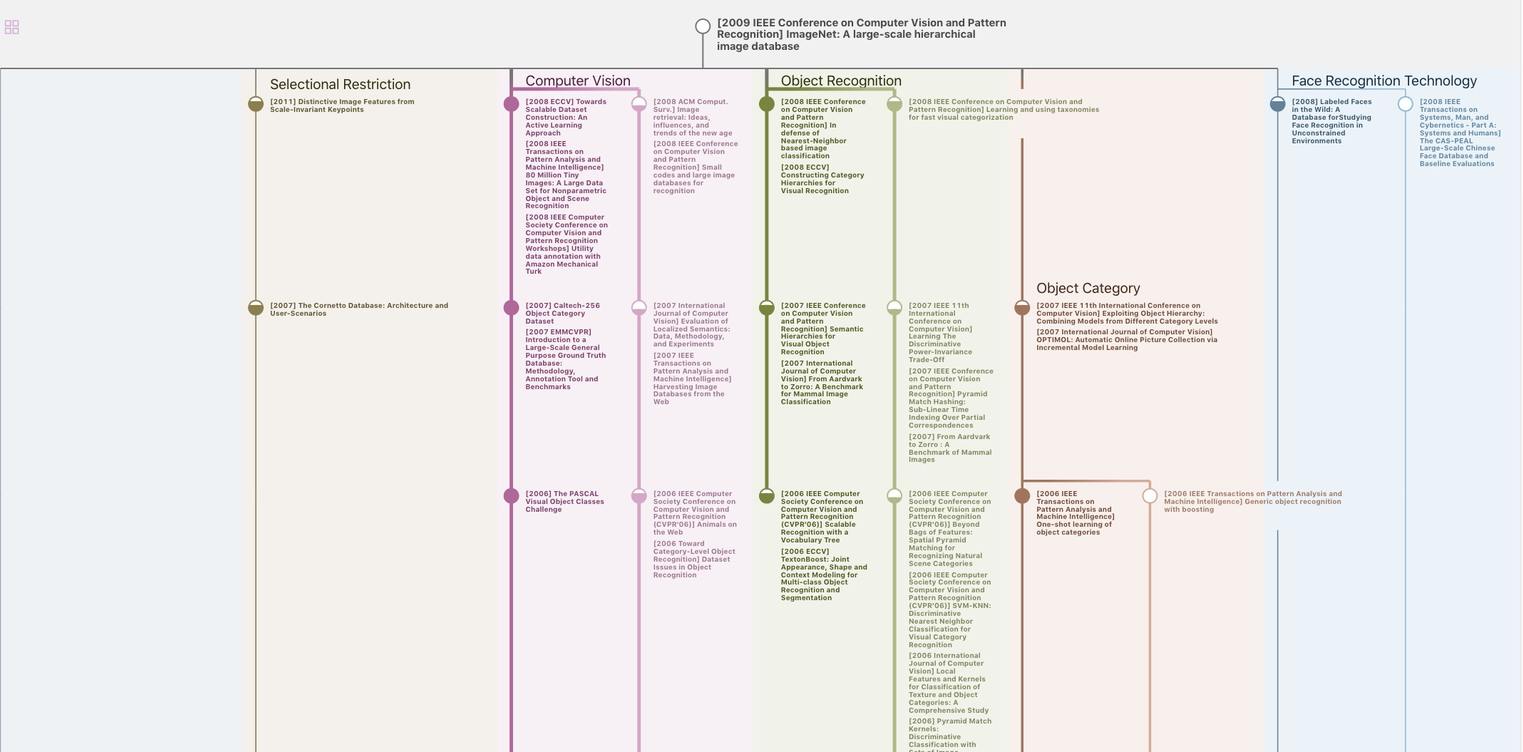
生成溯源树,研究论文发展脉络
Chat Paper
正在生成论文摘要