Low Revenue in Display Ad Auctions: Algorithmic Collusion vs. Non-Quasilinear Preferences
arxiv(2023)
摘要
The transition of display ad exchanges from second-price to first-price
auctions has raised questions about its impact on revenue, but evaluating these
changes empirically proves challenging. Automated bidding agents play a
significant role in this transition, often employing dynamic strategies that
evolve through exploration and exploitation rather than using the static
game-theoretical equilibrium strategies. Thus revenue equivalence between
first- and second-price auctions might not hold. Research on algorithmic
collusion in display ad auctions found that first-price auctions can induce
Q-learning agents to tacitly collude below the Nash equilibrium, which leads to
lower revenue compared to the second-price auction. Our analysis explores
widespread online learning algorithms' convergence behavior in both complete
and incomplete information models but does not find systematic deviance from
equilibrium behavior. Convergence for Q-learning depends on hyperparameters and
initializations, and algorithmic collusion also vanishes when Q-learning agents
are competing against other learning algorithms. The objective of bidding
agents in these auctions is typically to maximize return-on-investment or
return-on-spend, but not necessarily payoff maximization. The revenue
comparison under such utility functions is an open question. Analytical
derivations of equilibrium are challenging, but learning algorithms allow us to
approximate equilibria and predict the outcome when agents have such
non-quasilinear objectives. Our analysis shows that if learning agents aim to
optimize such objectives rather than payoff, then the second-price auction
achieves higher expected revenue compared to the first-price auction.
Understanding the intricate interplay of auction rules, learning algorithms,
and utility models is crucial in the ever-evolving world of advertising
markets.
更多查看译文
AI 理解论文
溯源树
样例
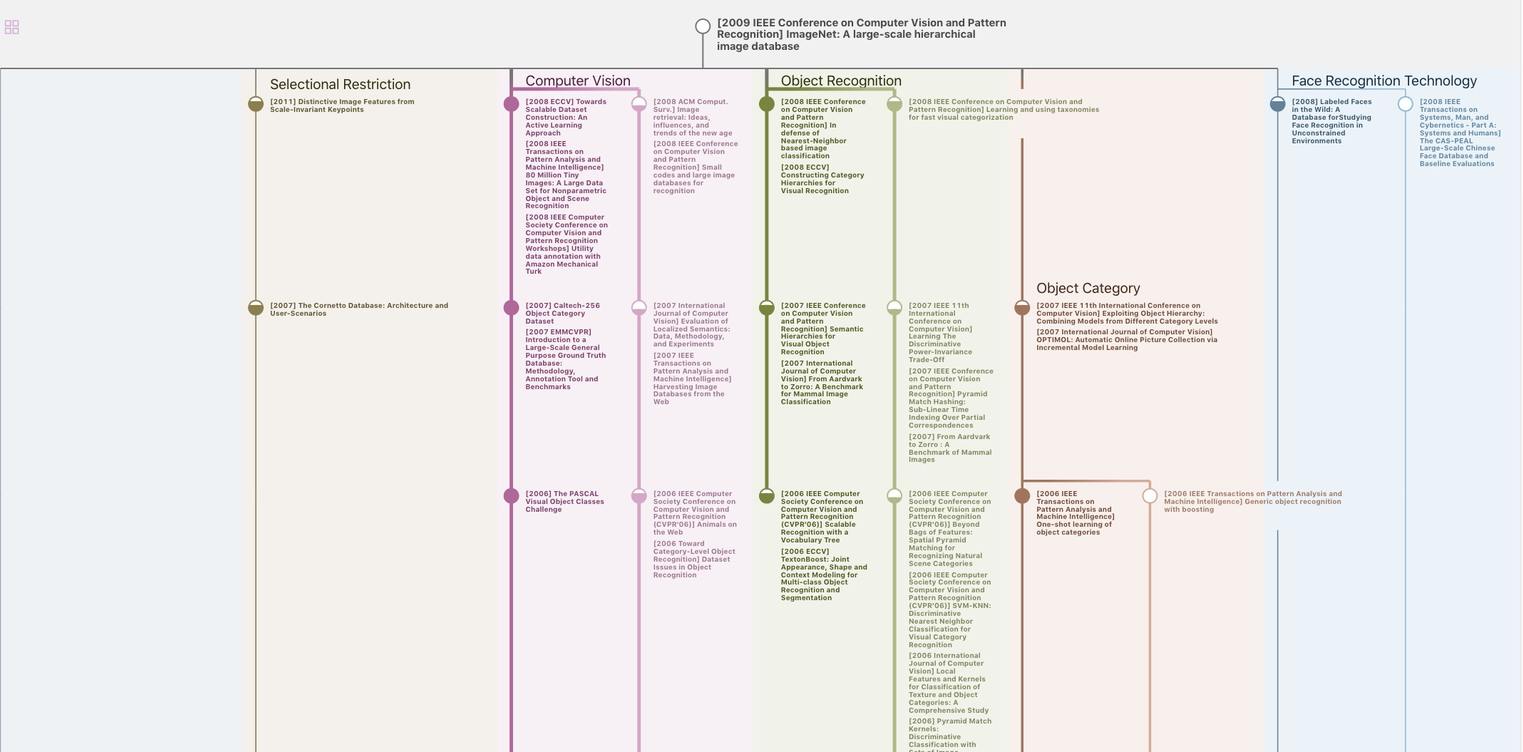
生成溯源树,研究论文发展脉络
Chat Paper
正在生成论文摘要