MIA-BAD: An Approach for Enhancing Membership Inference Attack and its Mitigation with Federated Learning
2024 International Conference on Computing, Networking and Communications (ICNC)(2023)
Abstract
The membership inference attack (MIA) is a popular paradigm for compromising
the privacy of a machine learning (ML) model. MIA exploits the natural
inclination of ML models to overfit upon the training data. MIAs are trained to
distinguish between training and testing prediction confidence to infer
membership information. Federated Learning (FL) is a privacy-preserving ML
paradigm that enables multiple clients to train a unified model without
disclosing their private data. In this paper, we propose an enhanced Membership
Inference Attack with the Batch-wise generated Attack Dataset (MIA-BAD), a
modification to the MIA approach. We investigate that the MIA is more accurate
when the attack dataset is generated batch-wise. This quantitatively decreases
the attack dataset while qualitatively improving it. We show how training an ML
model through FL, has some distinct advantages and investigate how the threat
introduced with the proposed MIA-BAD approach can be mitigated with FL
approaches. Finally, we demonstrate the qualitative effects of the proposed
MIA-BAD methodology by conducting extensive experiments with various target
datasets, variable numbers of federated clients, and training batch sizes.
MoreTranslated text
Key words
Federated Learning,Membership Inference Attack,Privacy,Security
AI Read Science
Must-Reading Tree
Example
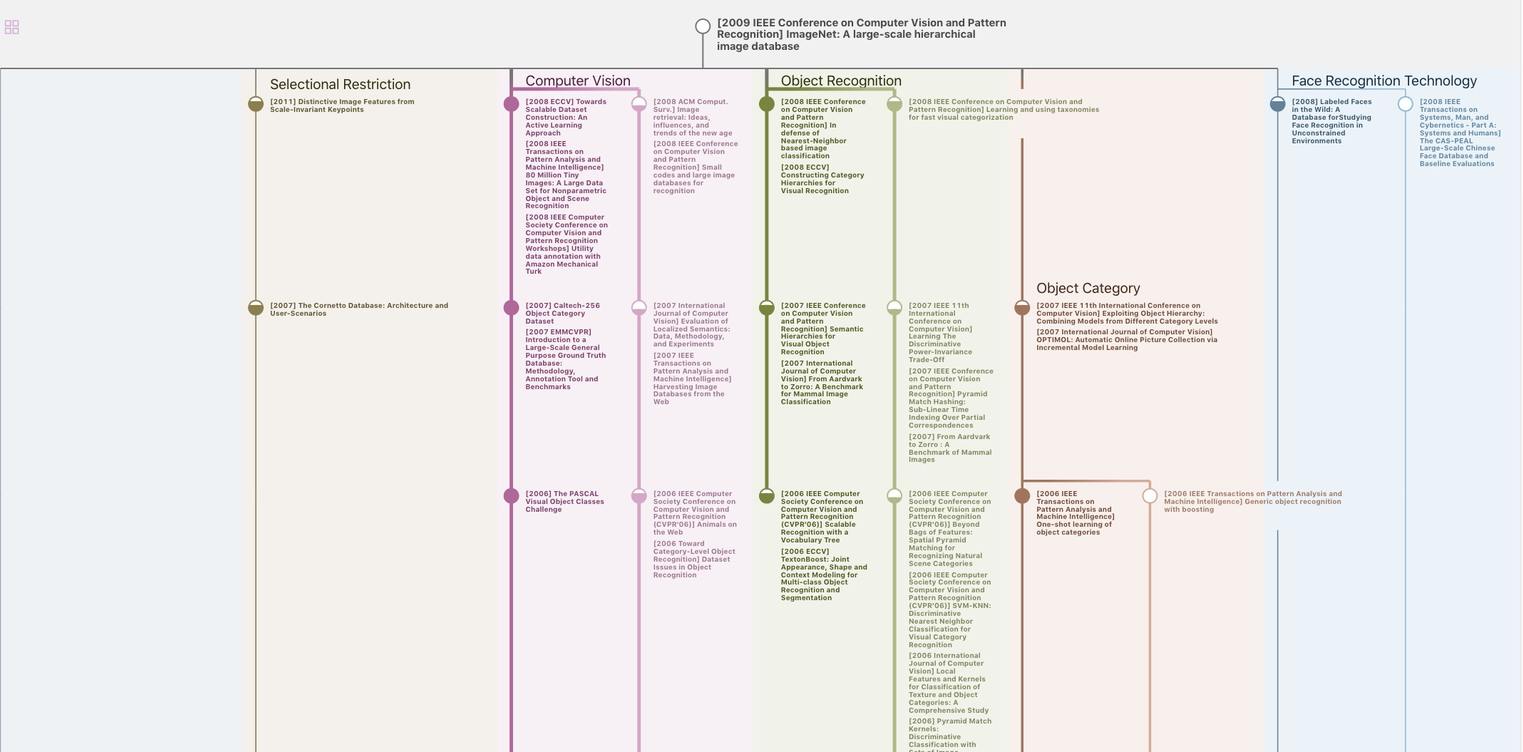
Generate MRT to find the research sequence of this paper
Chat Paper
Summary is being generated by the instructions you defined