Inverse-Optimization-Based Uncertainty Set for Robust Linear Optimization
arxiv(2023)
Abstract
We consider solving linear optimization (LO) problems with uncertain objective coefficients. For such problems, we often employ robust optimization (RO) approaches by introducing an uncertainty set for the unknown coefficients. Typical RO approaches require observations or prior knowledge of the unknown coefficient to define an appropriate uncertainty set. However, such information may not always be available in practice. In this study, we propose a novel uncertainty set for robust linear optimization (RLO) problems without prior knowledge of the unknown coefficients. Instead, we assume to have data of known constraint parameters and corresponding optimal solutions. Specifically, we derive an explicit form of the uncertainty set as a polytope by applying techniques of inverse optimization (IO). We prove that the RLO problem with the proposed uncertainty set can be equivalently reformulated as an LO problem. Numerical experiments show that the RO approach with the proposed uncertainty set outperforms classical IO in terms of performance stability.
MoreTranslated text
AI Read Science
Must-Reading Tree
Example
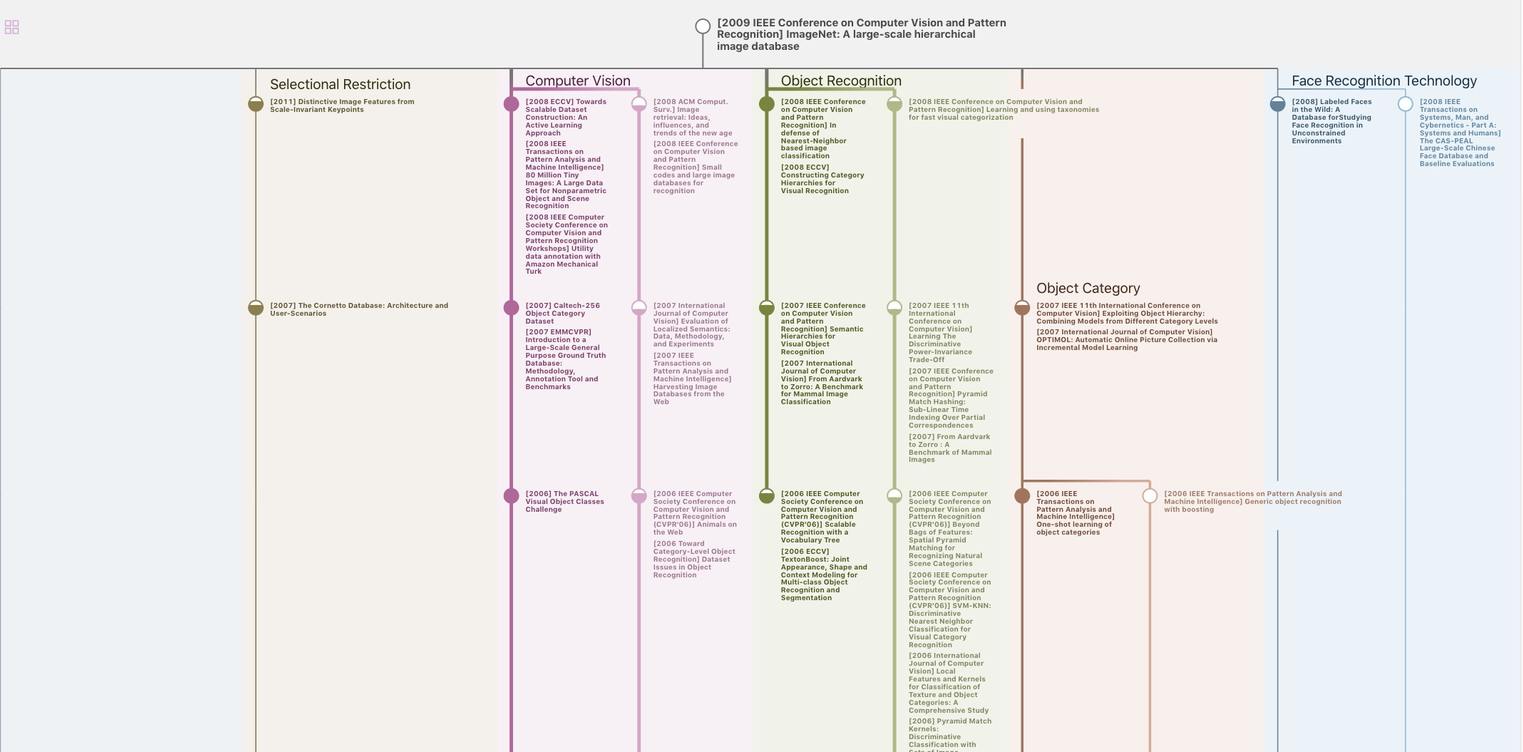
Generate MRT to find the research sequence of this paper
Chat Paper
Summary is being generated by the instructions you defined