Message-passing on hypergraphs: detectability, phase transitions and higher-order information
JOURNAL OF STATISTICAL MECHANICS-THEORY AND EXPERIMENT(2024)
摘要
Hypergraphs are widely adopted tools to examine systems with higher-order interactions. Despite recent advancements in methods for community detection in these systems, we still lack a theoretical analysis of their detectability limits. Here, we derive closed-form bounds for community detection in hypergraphs. Using a message-passing formulation, we demonstrate that detectability depends on the hypergraphs' structural properties, such as the distribution of hyperedge sizes or their assortativity. Our formulation enables a characterization of the entropy of a hypergraph in relation to that of its clique expansion, showing that community detection is enhanced when hyperedges highly overlap on pairs of nodes. We develop an efficient message-passing algorithm to learn communities and model parameters on large systems. Additionally, we devise an exact sampling routine to generate synthetic data from our probabilistic model. Using these methods, we numerically investigate the boundaries of community detection in synthetic datasets, and extract communities from real systems. Our results extend our understanding of the limits of community detection in hypergraphs and introduce flexible mathematical tools to study systems with higher-order interactions.
更多查看译文
关键词
inference of graphical models,message-passing algorithms,statistical inference
AI 理解论文
溯源树
样例
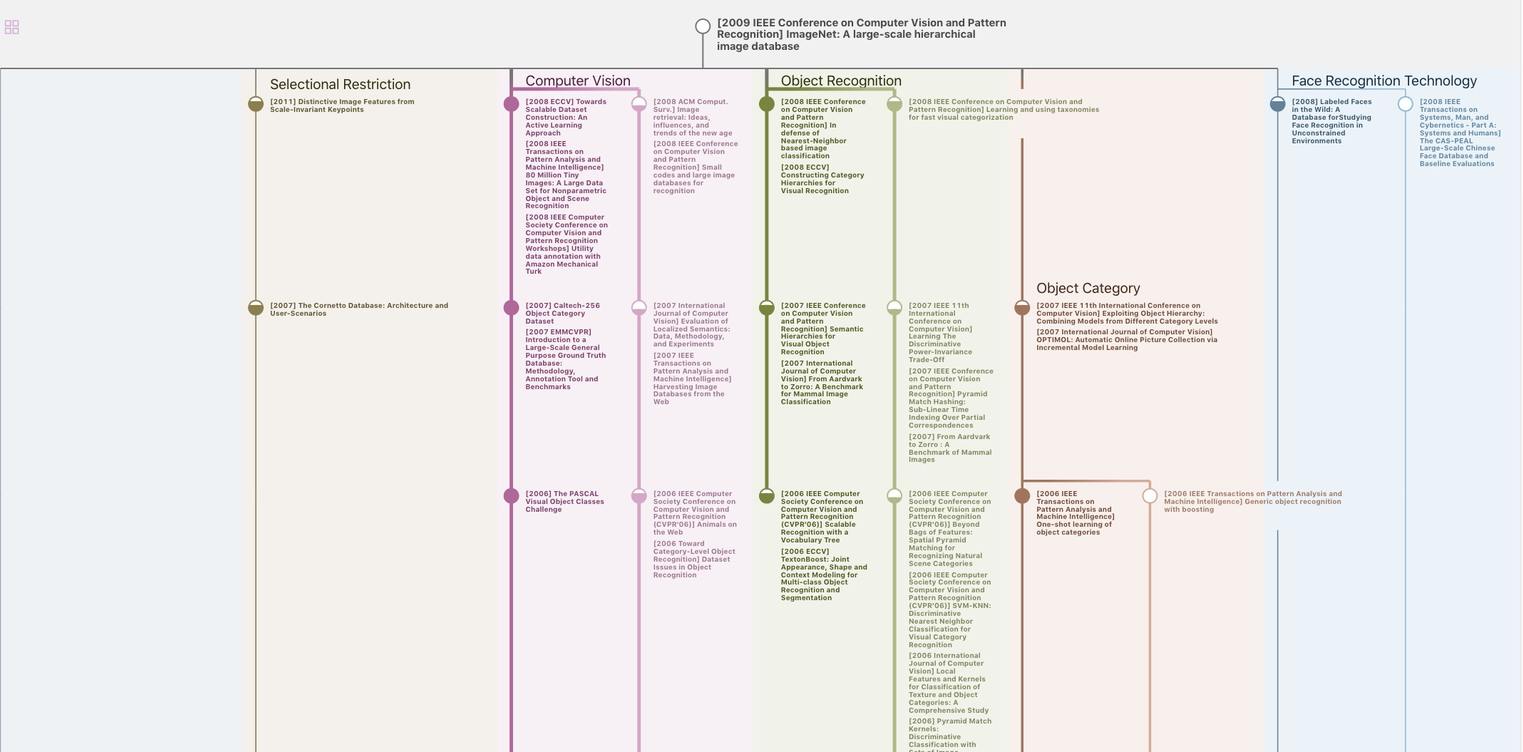
生成溯源树,研究论文发展脉络
Chat Paper
正在生成论文摘要