Towards robustness and generalization of point cloud representation: A geometry coding method and a large-scale object-level dataset
Computational Visual Media(2024)
摘要
Robustness and generalization are two challenging problems for learning point cloud representation. To tackle these problems, we first design a novel geometry coding model, which can effectively use an invariant eigengraph to group points with similar geometric information, even when such points are far from each other. We also introduce a large-scale point cloud dataset, PCNet184. It consists of 184 categories and 51,915 synthetic objects, which brings new challenges for point cloud classification, and provides a new benchmark to assess point cloud cross-domain generalization. Finally, we perform extensive experiments on point cloud classification, using ModelNet40, ScanObjectNN, and our PCNet184, and segmentation, using ShapeNetPart and S3DIS. Our method achieves comparable performance to state-of-the-art methods on these datasets, for both supervised and unsupervised learning. Code and our dataset are available at https://github.com/MingyeXu/PCNet184 .
更多查看译文
关键词
geometry coding,self-supervised learning,point cloud,classification,segmentation,3D analysis
AI 理解论文
溯源树
样例
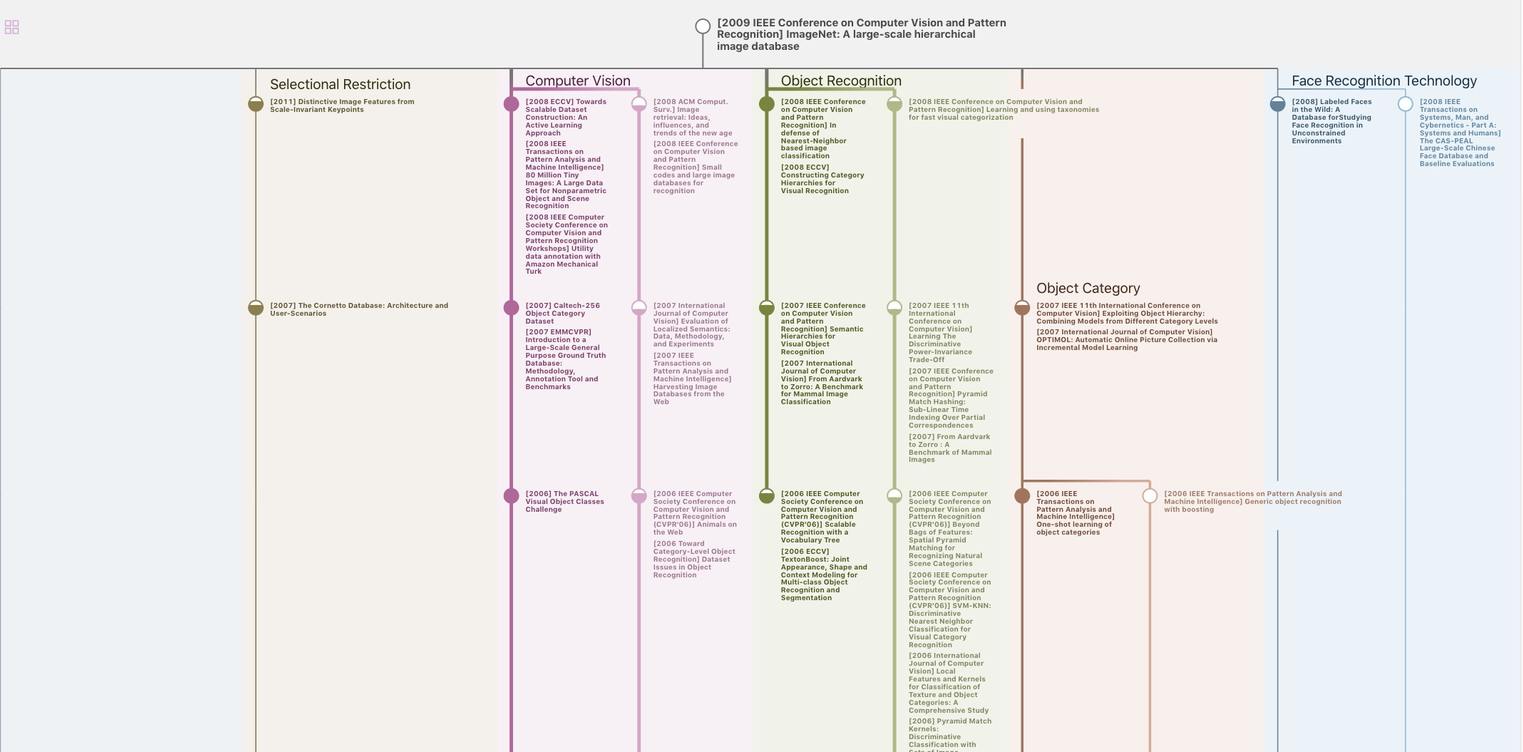
生成溯源树,研究论文发展脉络
Chat Paper
正在生成论文摘要