ThermoLink: Bridging Disulfide Bond and Enzyme Thermostability through Database Construction and Machine Learning Prediction
biorxiv(2023)
摘要
Disulfide bonds (SS bonds), covalently formed by sulfur atoms in cysteine residues, play a crucial role in protein folding and structure stability. Due to their significance, artificial disulfide bonds are often introduced to enhance the thermostability of proteins. Although an increasing number of tools can assist with this task, significant amounts of time and resources were often wasted due to inadequate consideration. To enhance the accuracy and efficiency of designing disulfide bonds for protein thermostability improvement, we first collected disulfide-bond data with protein thermostability data from extensive literature sources. Then, various sequence- and structure-based features were extracted, and machine learning models were constructed to predict whether a disulfide bond could improve protein thermostability. Among all models, the neighborhood context model using the Adaboost-DT algorithm performs the best, and the AUC-ROC score and accuracy are 0.773 and 0.714, respectively. Alongside this, we also found that AlphaFold2 exhibits a high superiority in predicting disulfide bonds, and the coevolutionary relationship between residue pairs, to some extent, could also guide artificial disulfide-bond design. The SS-bond data has been integrated into an online server, named ThermoLink, available at guolab.mpu.edu.mo/thermoLink.
### Competing Interest Statement
The authors have declared no competing interest.
更多查看译文
AI 理解论文
溯源树
样例
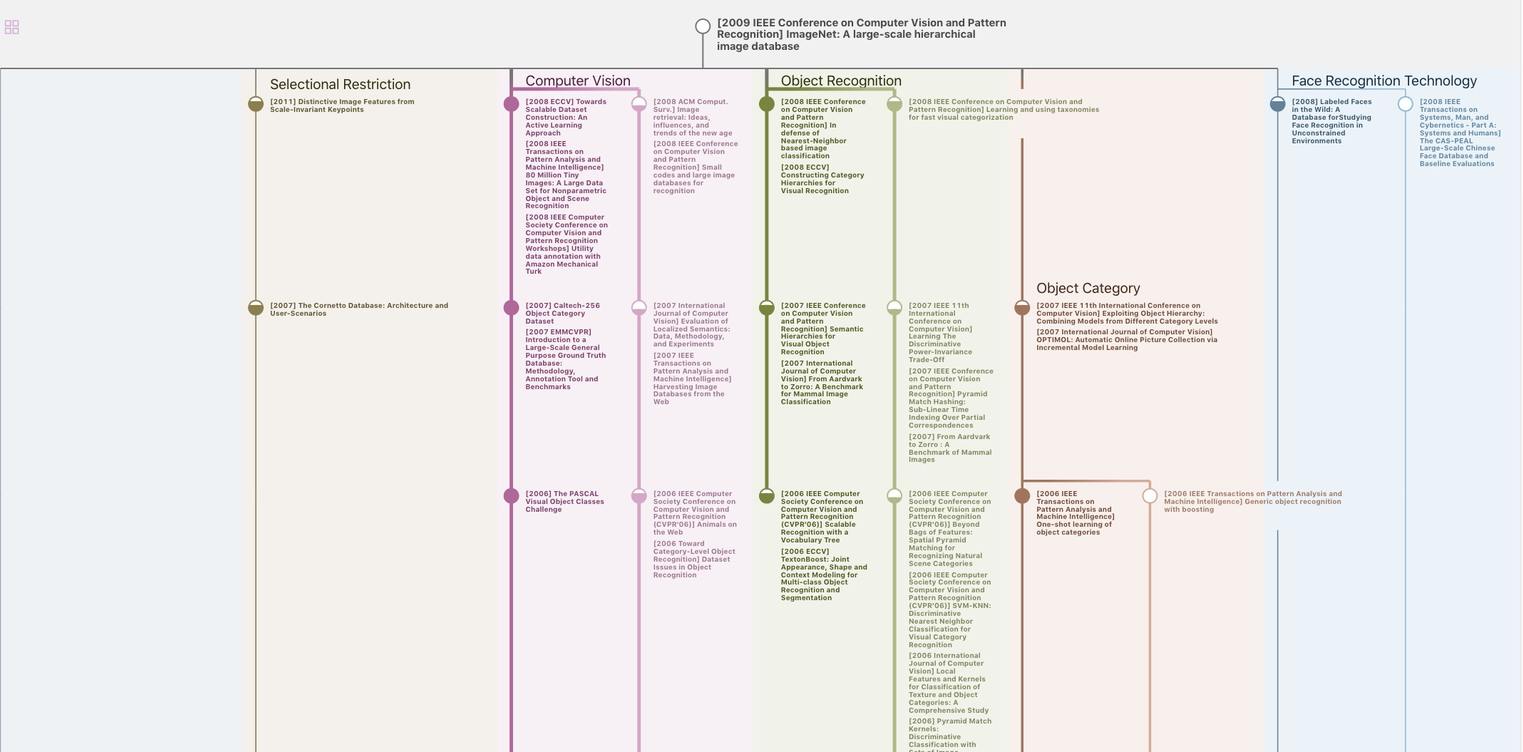
生成溯源树,研究论文发展脉络
Chat Paper
正在生成论文摘要