An evolutionary functional link artificial neural network for assessment of compressive strength of concrete structures
AIN SHAMS ENGINEERING JOURNAL(2024)
摘要
Compressive strength (CS) has been considered as the utmost critical parameter while designing concrete structures. Usually, it is determined through laboratory tests, which are expensive, time consuming, and requires consumptions of materials. Therefore, correct prediction of CS before actual placement of concrete is highly desirable. The relationship among the constituent materials that forms concrete structures is highly nonlinear and necessitates application of intelligent methods. Though a few such methods like artificial neural network (ANN) based models are available in the literature; their performance in the context is limited to certain extent and they have their own merits and demerits. Hence, to address some of the limitations of ANN (non-higher order Neural Network) based models, this contribution proposed a hybrid model in which a flat network i.e., a type of higher order neural network-functional link artificial neural network (FLANN) is used as the base structure and genetic algorithm (GA) is employed to find out the optimal FLANN parameters (i.e., GA + FLANN). The training process comprises selection of connection weights, bias, as well as optimal number of basis functions of FLANN by GA rather fixing them earlier. Thus, an optimal FLANN is crafted on fly from exploitation of training data. The proposed model is used to assess the CS of concrete cements from datasets available in the literature considering samples of curing age at 3, 7, 14, 28, 56, and 91 days. A rolling window is used for input selection and five evaluation metrics are used for performance evaluation. On an average, the GA + FLANN obtained 0.477052 MAPE (mean absolute percentage of error), 0.598067 ARV (average relative variance), 0.593862 UT (U of Theil's statistic), 0.551452 NMSE (normalized mean squared error), and 0.206135 SD (standard deviation) values which are the lowest compared to others. The superiority of the model is established through comparative studies and statistical significance tests.
更多查看译文
关键词
Compressive strength,Artificial neural network,Functional link artificial neural network,Genetic algorithm,Evolutionary algorithm,Predictive model
AI 理解论文
溯源树
样例
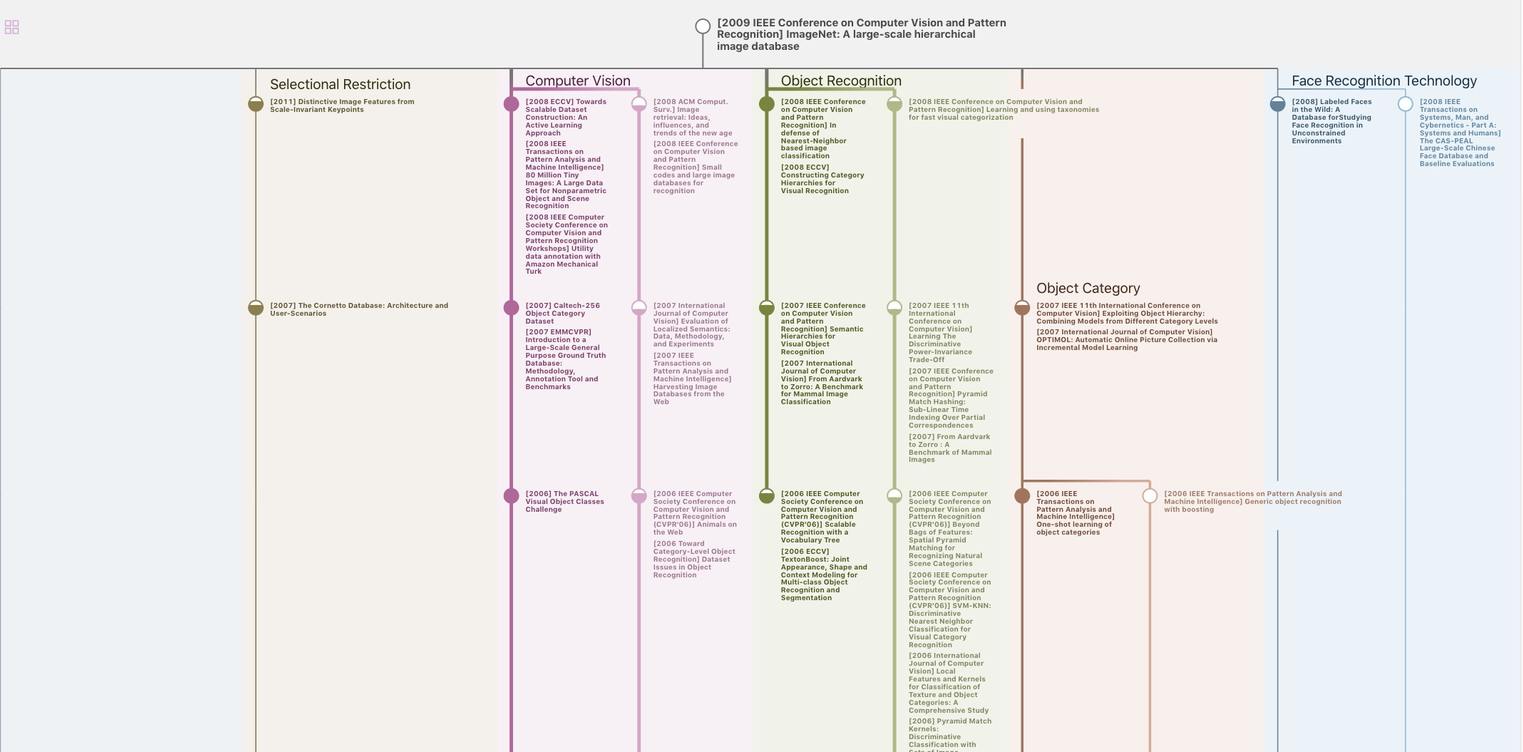
生成溯源树,研究论文发展脉络
Chat Paper
正在生成论文摘要