An efficient deep-learning-based solution for the recognition of relative changes in mental workload using wearable sensors
2023 IEEE SENSORS(2023)
摘要
In this work, a new solution for the automatic recognition of relative changes in mental workload is proposed. Wearable sensors were used to collect EEG, EDA, PPG and eye-tracking data from 26 human subjects while performing the n-back task with three difficulty levels n is an element of {1, 2, 3}. The objective is to recognize whether the mental workload is increasing, decreasing or stable by comparing the current signals' window with a previous one. The proposed 3-class classifier uses mainly CNN layers with a novel merging layer that systematically captures the interactions between local segments of the two inspected windows. In fact, it is inspired by the competitive success of both transformer- and CNN-based networks in time series classification. While the proposed solution exploits the efficiency of CNN networks, it also enjoys, similar to transformers, the capacity of capturing the interactions between local events of the sequence thanks to the proposed merging layer. In terms of accuracy, experimental results show the superiority of the proposed solution over classical CNN, BiLSTM and transformer networks on eye-direction, PPG and EEG data while its performance is comparable with the transformer networks on eye-pupil-diameter and EDA data. The average training time per epoch is considerably smaller than the ones of transformer and BiLSTM networks as shown in the experimental results.
更多查看译文
关键词
Mental workload (MWL),deep neural networks (DNNs),time series classification (TSC),eye-tracking,photoplethysmogram (PPG),electroencephalogram (EEG),electrodermal activity (EDA),n-back task,transformer neural network,convolutional neural network (CNN)
AI 理解论文
溯源树
样例
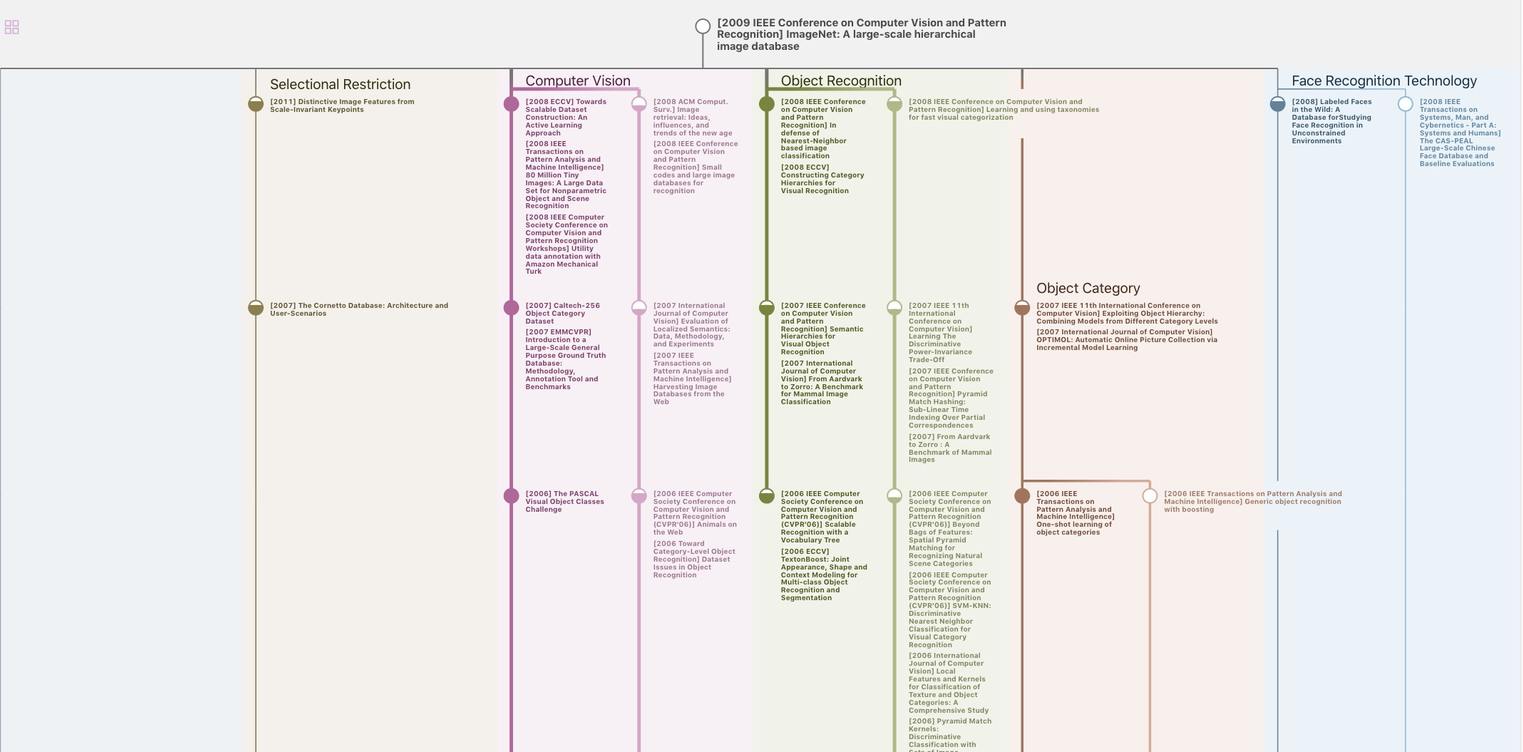
生成溯源树,研究论文发展脉络
Chat Paper
正在生成论文摘要