Machine Learning Study on the Electron Emission Properties of the Thermionic Cathode Impregnants
2023 24th International Vacuum Electronics Conference (IVEC)(2023)
摘要
The emission current density of thermionic cathode materials is predicted using machine learning methods. Firstly, four classical regression algorithms of linear regression (LR), random forest regression (RFR), XGBoost, and gradient boosting regression tree (GBRT) are used. The relation coefficient
$\boldsymbol{R}^{\mathbf{2}}$
is chosen for the model evaluation metrics, and the prediction results are 0.174, 0.677, 0.812, and 0.915, respectively. Secondly, to solve the problems of small training data and unstable prediction results, the small sample TrAdaboost regression algorithm is introduced to predict and adjust the parameters for each system with different percentages of each chemical component (612, 512, 411, and others), and the final prediction results
$\mathbf{R}^{\mathbf{2}}$
are obtained as 0.954, 0.95, 0.5 and 0.939. The prediction accuracy is significantly improved.
更多查看译文
关键词
Thermionic cathode material,Emission current density,Machine learning,Small sample learning
AI 理解论文
溯源树
样例
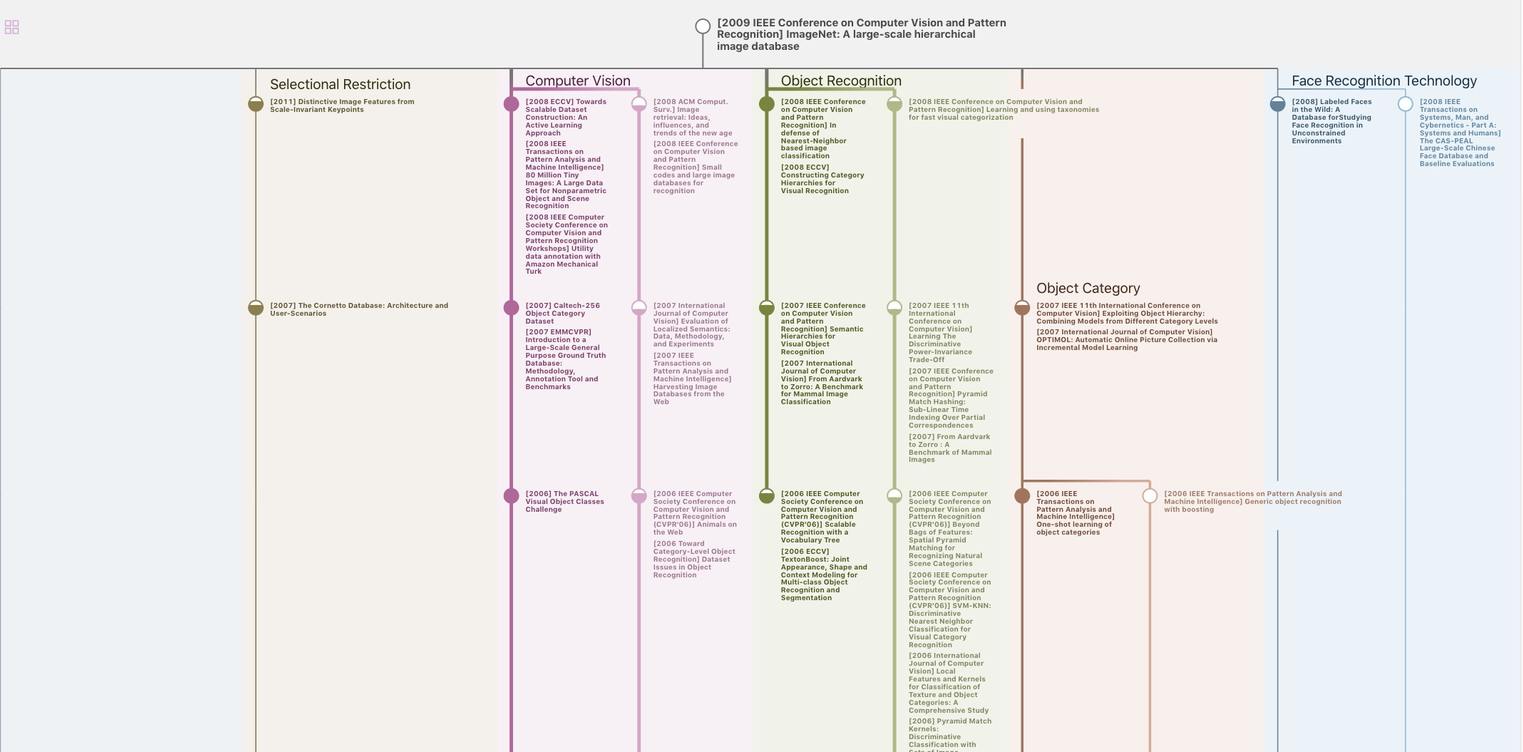
生成溯源树,研究论文发展脉络
Chat Paper
正在生成论文摘要