Typical Scenario Extraction of Distributed Rooftop Photovoltaic Power Output Using Improved Deep Convolutional Embedded Clustering
2023 6th International Conference on Energy, Electrical and Power Engineering (CEEPE)(2023)
摘要
The increase of the penetration rate of distributed rooftop photovoltaic (PV) in the distribution network brings uncertainties to the distribution network operation scenarios. It is difficult to meet the actual demand relying on manual operation to extract typical scenarios. To tackle this issue, this paper proposes an improved One-dimensional Deep Convolutional Embedded Clustering with ResNet Autoencoder (1D-RDCEC) based scenario reduction method to extract typical PV power output scenarios. Massive PV power output scenarios are generated by Conditional Generative Adversarial Networks (CGAN) with monthly labels, in order to provide sufficient and high-quality scenario set for the subsequent extraction of typical scenarios. 1D-RDCEC first uses a One-Dimensional Convolutional Autoencoder adding residual connection (1D-RCAE) to extract the latent features of PV power output. Then, a custom clustering layer is used to soft assign the extracted latent features. Finally, the clustering loss and reconstruction loss are combined as a joint optimization to extract typical scenarios of distributed rooftop PV power output. Experiments on Australian distribution network datasets have demonstrated the effectiveness of the proposed method.
更多查看译文
关键词
typical scenario extraction,distributed rooftop photovoltaic,deep convolutional embedded clustering,residual connection
AI 理解论文
溯源树
样例
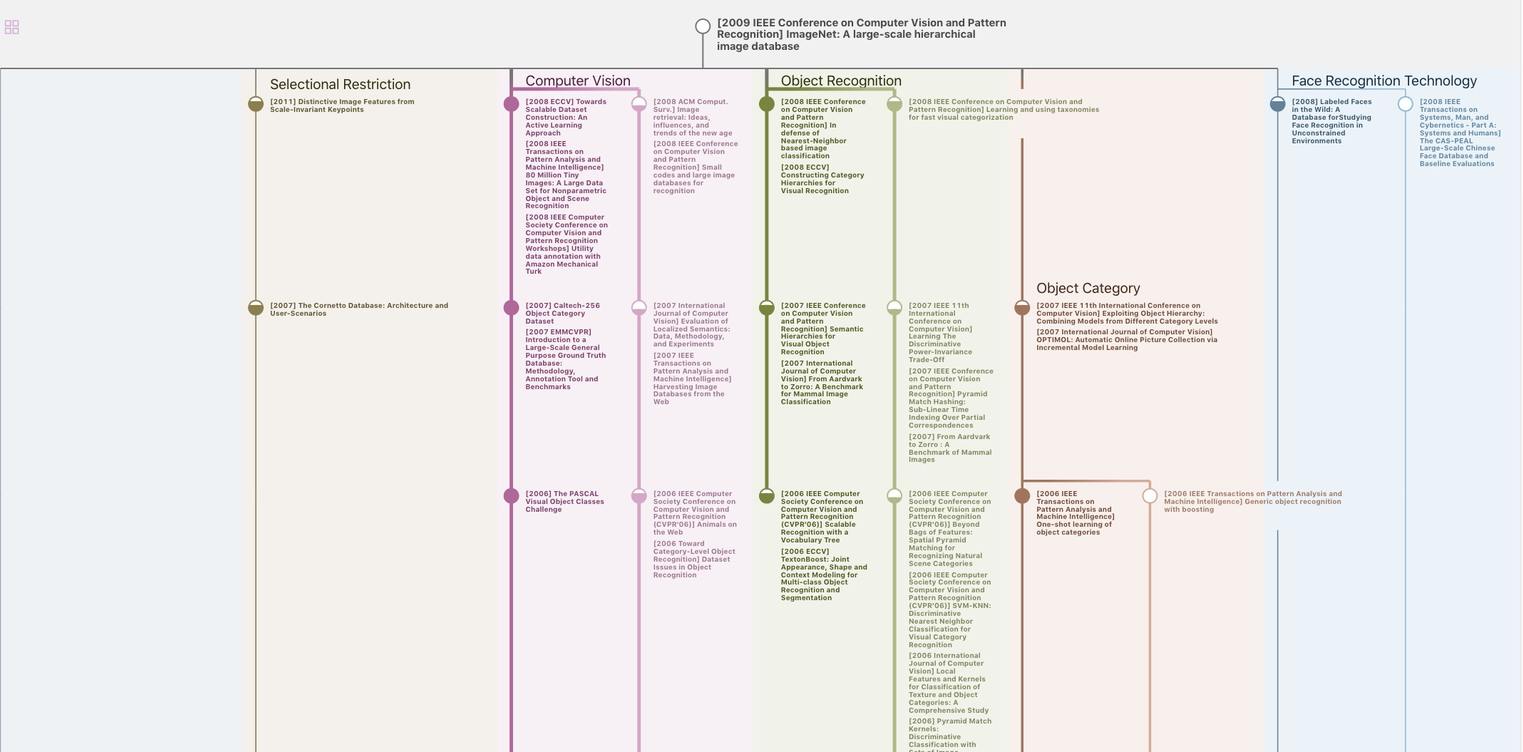
生成溯源树,研究论文发展脉络
Chat Paper
正在生成论文摘要