Disease Cluster Specific learning for Patient Treatment Prediction on High Dimensional EHR Data
2023 9th International Conference on Advanced Computing and Communication Systems (ICACCS)(2023)
摘要
Disease treatment Prediction is considered as significant research in the data analytics in medical domains. The autoencoder model is found be the highly productive model for clustering the disease treatment trajectory data. The processed dataset contains the disease related features which helps in transforming the data in high dimensional to the data in low dimensional way. Preprocessing of the trajectory data is carried out using missing value imputation and normalization process. The primary features are computed by the autoencoder learning process in a subsequent step while retaining the least reconstruction error. In order to avoid over fitting problems, the model also includes multiple layers to represent the different feature types and clustering. Additionally, the Loss function and encoder function are tuned along with all of the model's hyper parameters. In map structure, this encoder function technique is considered for mapping feature model with the latent feature model. To end, computation of the hyperparameter to be introduced for prediction of the disease treatment. Detailed experiments analyses were performed on EHR datasets to compare current architecture with conventional approaches. The experiment's results show that disease cluster specific clustering processes high dimensional data with great effectiveness and increased scalability
更多查看译文
关键词
Patient Trajectory Data,Disease Treatment Prediction,Deep Learning,Autoencoder,Electronic Health Record,Reconstruction Error
AI 理解论文
溯源树
样例
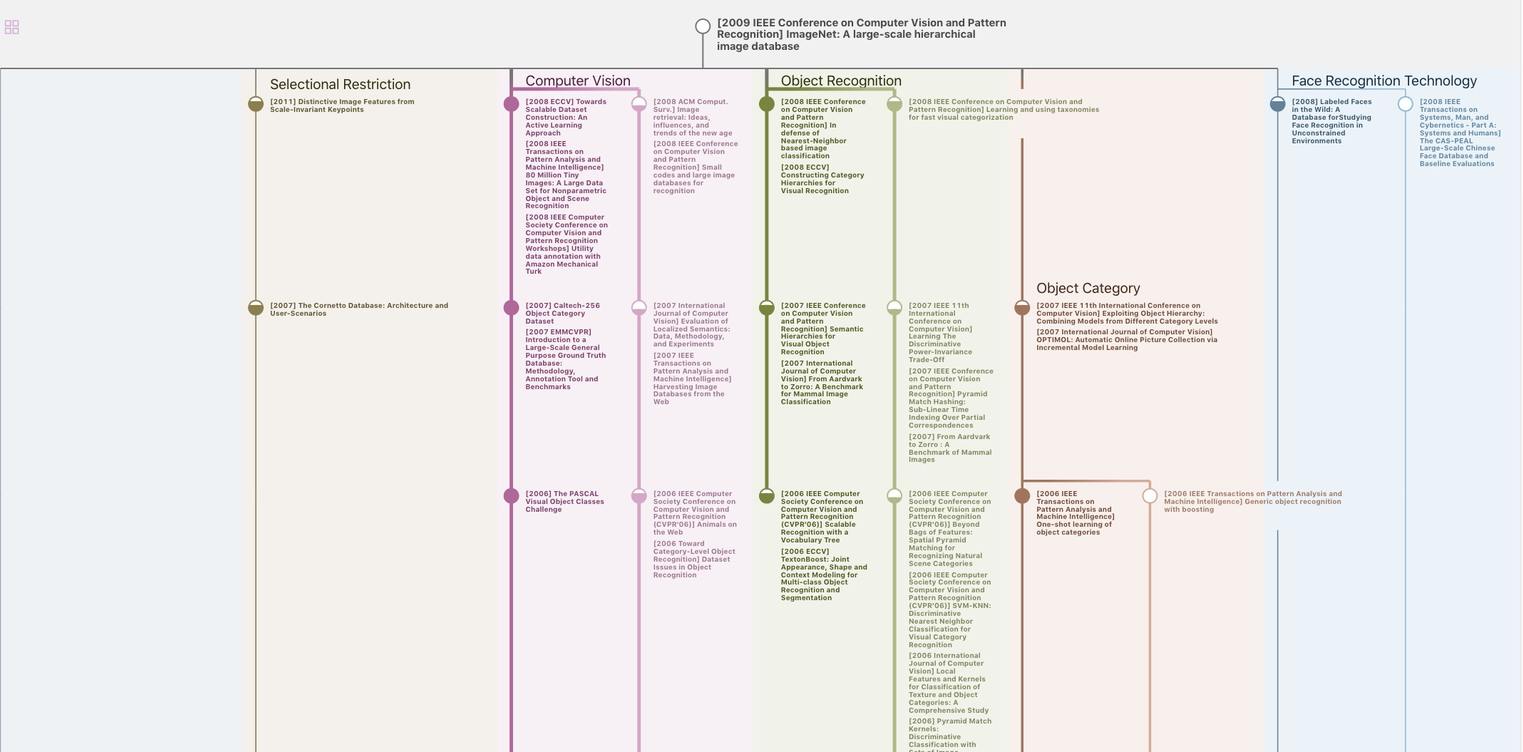
生成溯源树,研究论文发展脉络
Chat Paper
正在生成论文摘要