Label-Consistent Convolutional Dictionary Learning for Machine Inspection
2023 31st European Signal Processing Conference (EUSIPCO)(2023)
摘要
Machine fault diagnosis is crucial for predicting and preventing unexpected failures. In most practical application scenarios of machine inspection, access to data is limited. Moreover, the labeled instances are few, which makes it challenging for the existing data-driven techniques to learn efficient representations. To address this issue, we propose a novel label-consistent convolutional dictionary learning for machine fault classification. This method employs a joint optimization formulation for learning the dictionary atoms, corresponding coefficients, and weights associated with a label-consistency term. The label-consistency term added in the joint formulation helps in learning class-discriminative dictionaries. The features generated from the class-discriminative dictionaries result in robust inferencing when used with an external classifier. The performance of the proposed method is evaluated using the publicly available CWRU dataset for bearing fault diagnosis. The results demonstrate the superior performance of the proposed method compared to other state-of-the-art deep learning and dictionary learning techniques for the data limited scenario. Furthermore, with only 10% of training data, the proposed method achieved an accuracy of around 85%, while the other methods provided less than 50%.
更多查看译文
关键词
Convolutional dictionary learning,Classification,Sparse representation,Supervised learning,Machine fault diagnosis
AI 理解论文
溯源树
样例
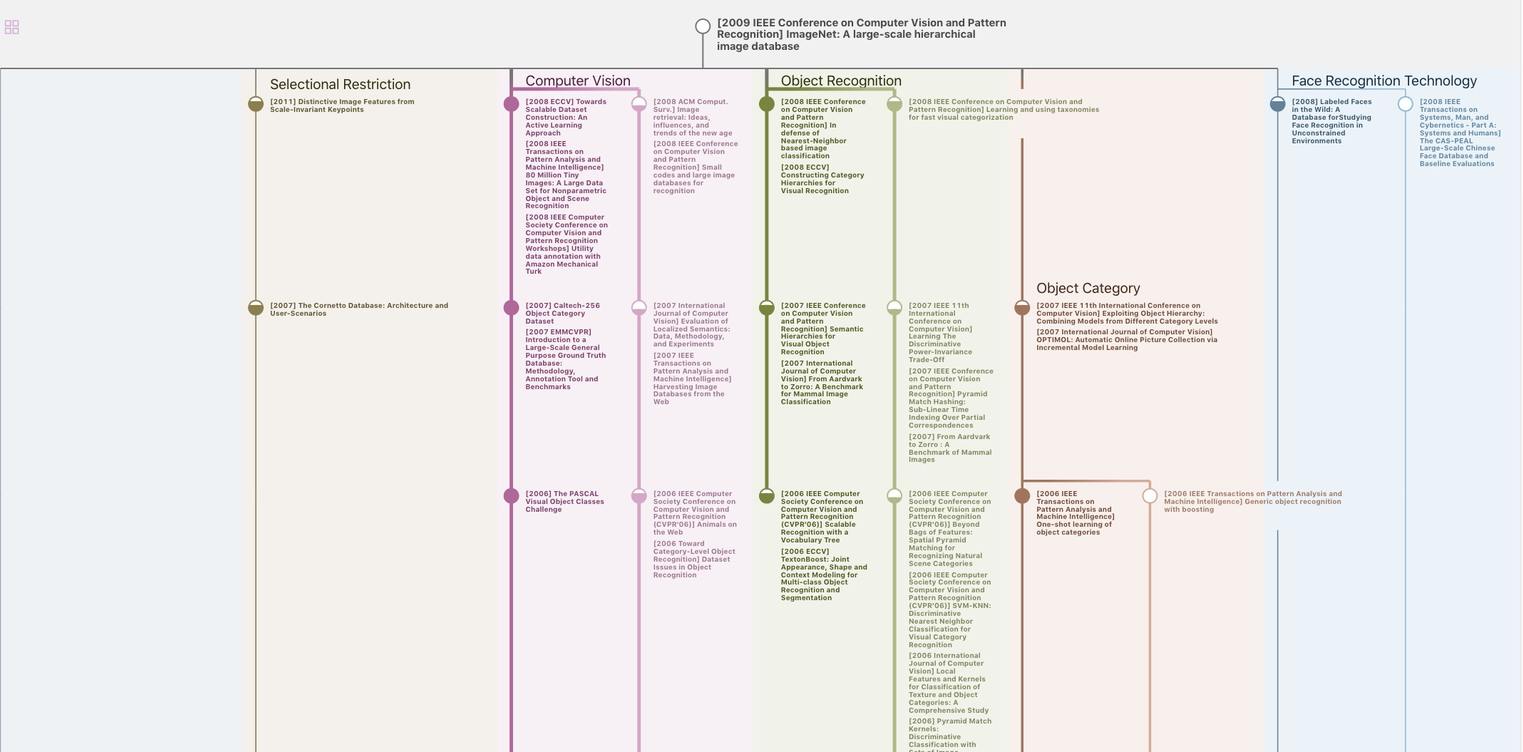
生成溯源树,研究论文发展脉络
Chat Paper
正在生成论文摘要