Proximal-Langevin Samplers for Nonsmooth Composite Posteriors: Application to the Estimation of Covid19 Reproduction Number
2023 31st European Signal Processing Conference (EUSIPCO)(2023)
摘要
Providing a level of confidence in the estimation of epidemiological indicators during pandemics is essential to inform decision makers. Monitoring the time evolution of the epidemic intensity despite the limited quality of the data is both crucial and challenging. For the estimation of the Covid-19 reproduction number through credibility intervals, a Bayesian model robust to errors in reported counts were proposed, yielding a non differentiable composite a posteriori log-density which required the design of advanced Proximal Langevin schemes. The first goal of this paper is to customize and compare on a pedagogically designed toy example, four different Hastings-Metropolis algorithms combining Langevin approaches and proximal operators. Then, the most efficient one is plugged into a Metropolis-within-Gibbs algorithm performing a credibility intervals-based estimation of Covid-19 pandemic indicators, exemplified for several countries worldwide.
更多查看译文
关键词
Markov chain Monte Carlo,log-concave composite density,Langevin Monte Carlo,Proximal operators,Bayesian credibility intervals,Covid-19,Reproduction number
AI 理解论文
溯源树
样例
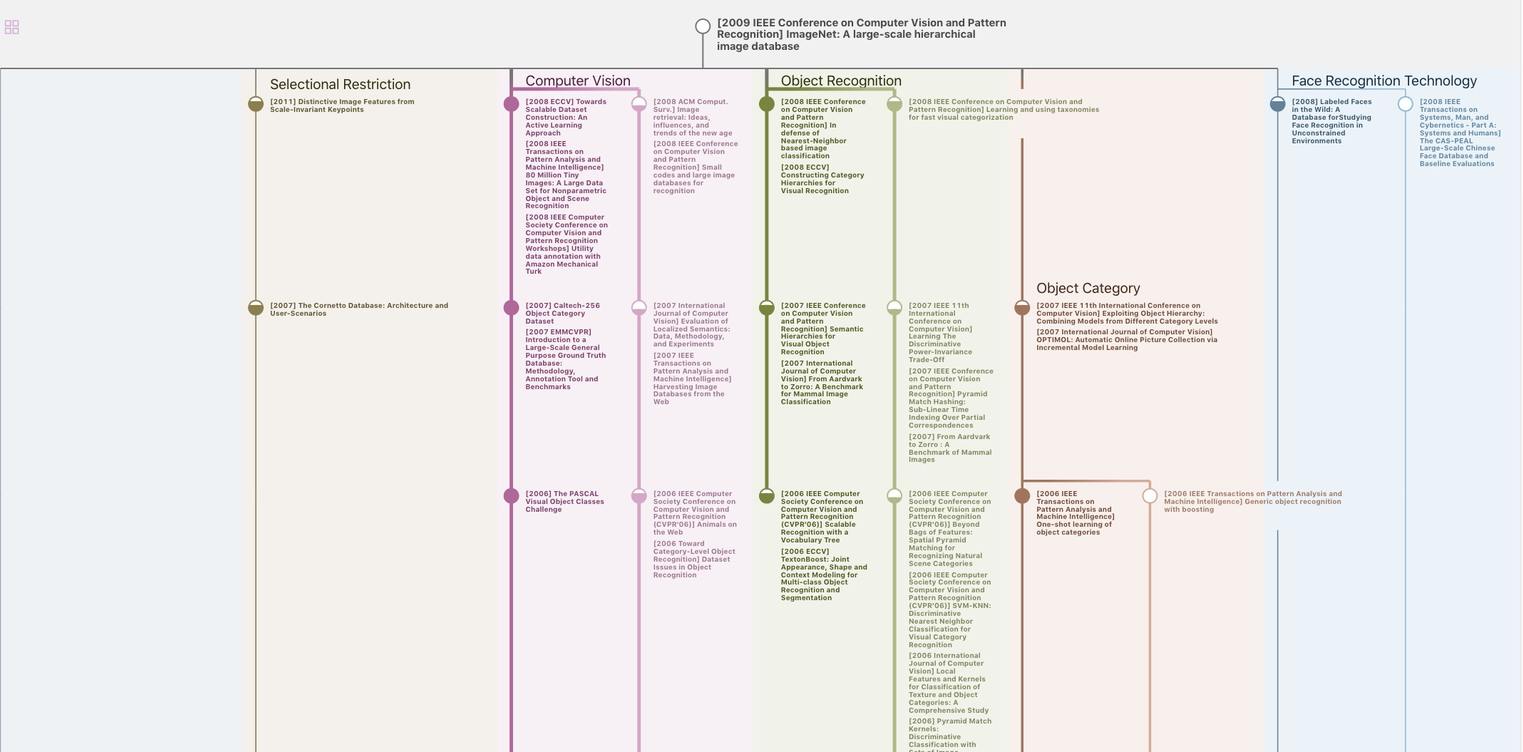
生成溯源树,研究论文发展脉络
Chat Paper
正在生成论文摘要