Uncertainty-Informed On-Device Personalisation Using Early Exit Networks on Sensor Signals
2023 31st European Signal Processing Conference (EUSIPCO)(2023)
摘要
As their computational capabilities improve, attention has turned towards deploying deep learning models on edge devices to process the locally generated sensor signals. While these devices remain comparatively resource-constrained, early exit approaches have been shown to reduce the computational demands of on-device model personalisation training, which improves the accuracy and latency of a generalised model by fitting it to a specific use scenario. However, existing methods provide no mechanism to select the most informative signals for training. This work aims to improve prior approaches by interpreting the early exits as an ensemble of models trained with a joint loss function, retaining prior approaches' energy and latency savings while improving the accuracy. Additionally, it provides a principled mechanism to choose the signals that introduce higher uncertainty to the prediction due to the distributional shift and include them in the personalisation procedure, reducing energy consumption and latency. The key findings are a 42 % energy saving with exit-only retraining versus a standard (without intermediate exits) model, which increases up to 79 % when a subset of training samples was chosen according to the uncertainty estimation, alongside a 4.23pp increase in F1 score.
更多查看译文
关键词
Personalisation,Uncertainty,Early Exit,Sensor Signal
AI 理解论文
溯源树
样例
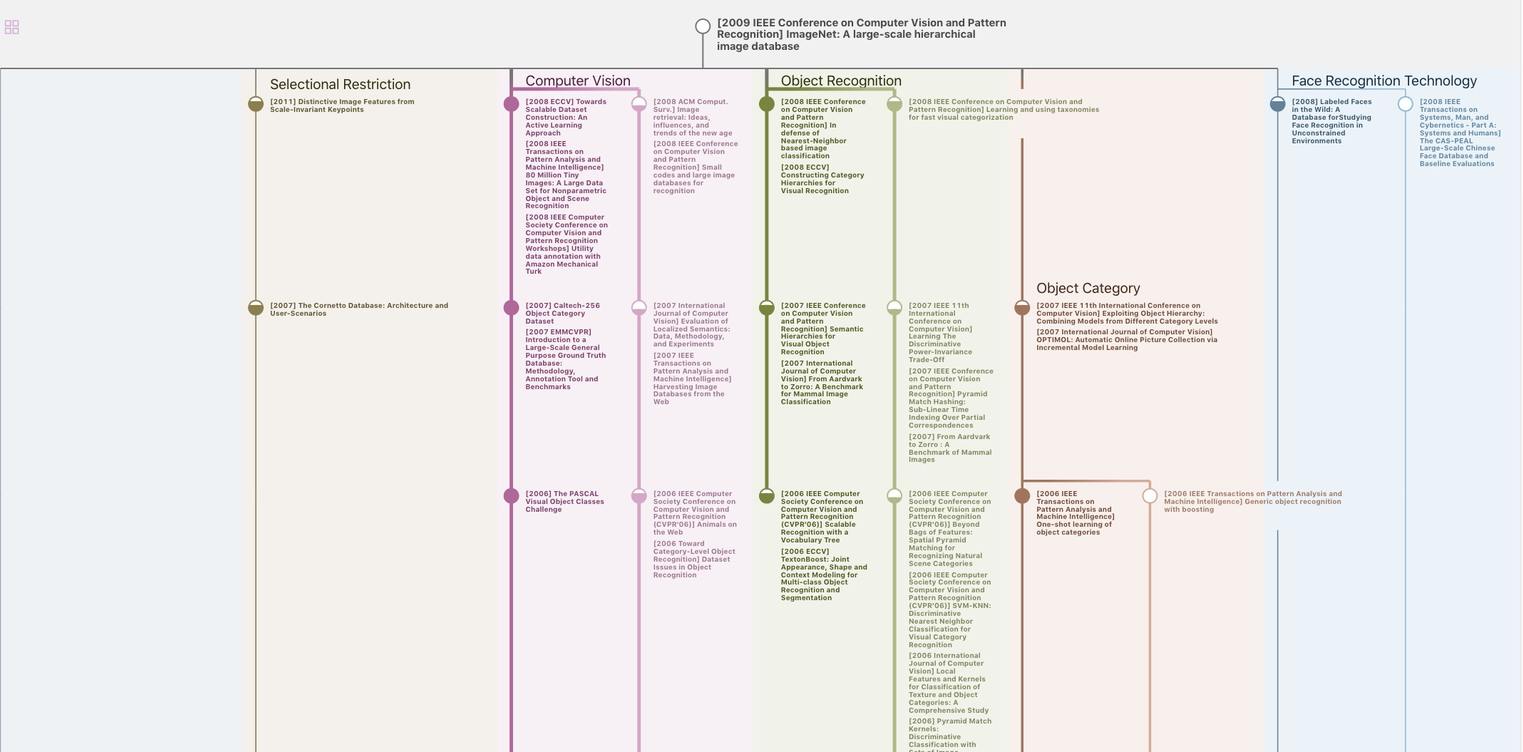
生成溯源树,研究论文发展脉络
Chat Paper
正在生成论文摘要