Self-Supervised Learning for Ultrasound Probe Angle Prediction in Plantar Fascia Images
2023 IEEE 19TH INTERNATIONAL CONFERENCE ON BODY SENSOR NETWORKS, BSN(2023)
摘要
This paper presents a self-supervised learning (SSL) approach for estimating the angular offset of freehand ultrasound (US) probe movement relative to an ideal viewing angle. Proper US probe angle is essential for acquiring high-quality images for diagnostics. This can be challenging, however, due to the high variability in anatomy and the subjectivity in interpretation. The ability to guide a user to an optimal probe angle can greatly improve the quality of image acquired which in turn will facilitate an accurate assessment. Probe angle is particularly important for musculoskeletal US since the probe often needs to be perpendicularly aligned with the target anatomy (e.g., bone, tendon, or ligament). In this paper, we used plantar fascia (PF) as the use case to develop a probe angle guidance pipeline. It has an immediate application for PF thickness measurement, which is used for assessing plantar fasciitis. In this SSL pipeline, circularized versions of over 3,600 US images of PF were each rotated by a varied amount, and an encoder-support vector machine pair was trained to predict this known angle of rotation. In a cross-validation from 13 subjects, the model predicted rotation angle with an average error of 2.98 degrees (SD: 2.62), and the SSL-trained model accurately predicted the relative rotation angles of images acquired while rotating the US probe. The key contribution of this work is the SSL approach on synthetically generated image and probe angle pairs to emulate angular motion relative to a standard view. This approach for estimating angular offset without labelled data has the potential to be applied to future work guiding users to an optimal view for acquiring US images.
更多查看译文
关键词
ultrasound,guidance,musculoskeletal injuries,self-supervised learning
AI 理解论文
溯源树
样例
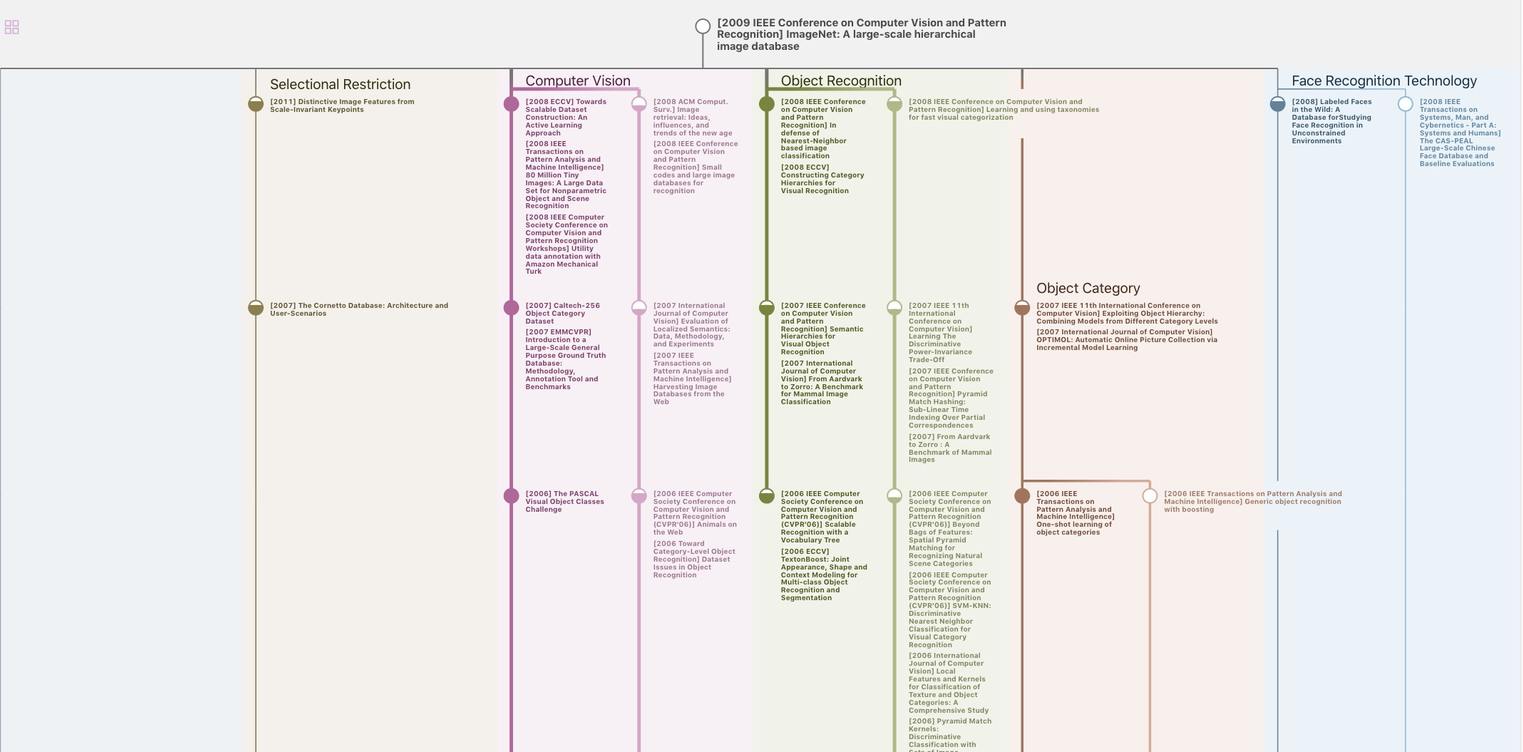
生成溯源树,研究论文发展脉络
Chat Paper
正在生成论文摘要