Combining Knee Acoustic Emissions, Patient-Reported Measures, and Machine Learning to Assess Osteoarthritis Severity
2023 IEEE 19th International Conference on Body Sensor Networks (BSN)(2023)
摘要
Current methods for quantifying osteoarthritis severity have limited resolution and accessibility. Patient-recorded outcome measures such as the Knee Injury and Osteoarthritis Outcome Score (KOOS) capture symptom severity, but are subjectively reported and have little correlation with quantifiable metrics of disease such as Kellgren-Lawrence x-ray grade or MRI findings. Knee acoustic emissions (KAEs) offer a convenient, noninvasive option for quantifying joint health. Here, we use machine learning and wearable design to create an interpretable two-stage algorithm for combining KAEs and KOOS scores into an objective, more accessible method of quantifying disease severity. Our algorithm successfully discriminated between early and late-stage osteoarthritis (balanced accuracy = 85%, ROC-AUC = 0.88). The addition of KAEs improved classification of osteoarthritis severity over the use of KAEs (balanced accuracy = 53%, ROC-AUC = 0.786) or KOOS scores alone (balanced accuracy = 63%, ROC-AUC = 0.593). The findings suggest that KAEs combined with patient-recorded metrics can be used to make a more objective and accessible metric for digitally monitoring knee joint health.
更多查看译文
关键词
osteoarthritis,knee acoustic emissions,KOOS,musculoskeletal health
AI 理解论文
溯源树
样例
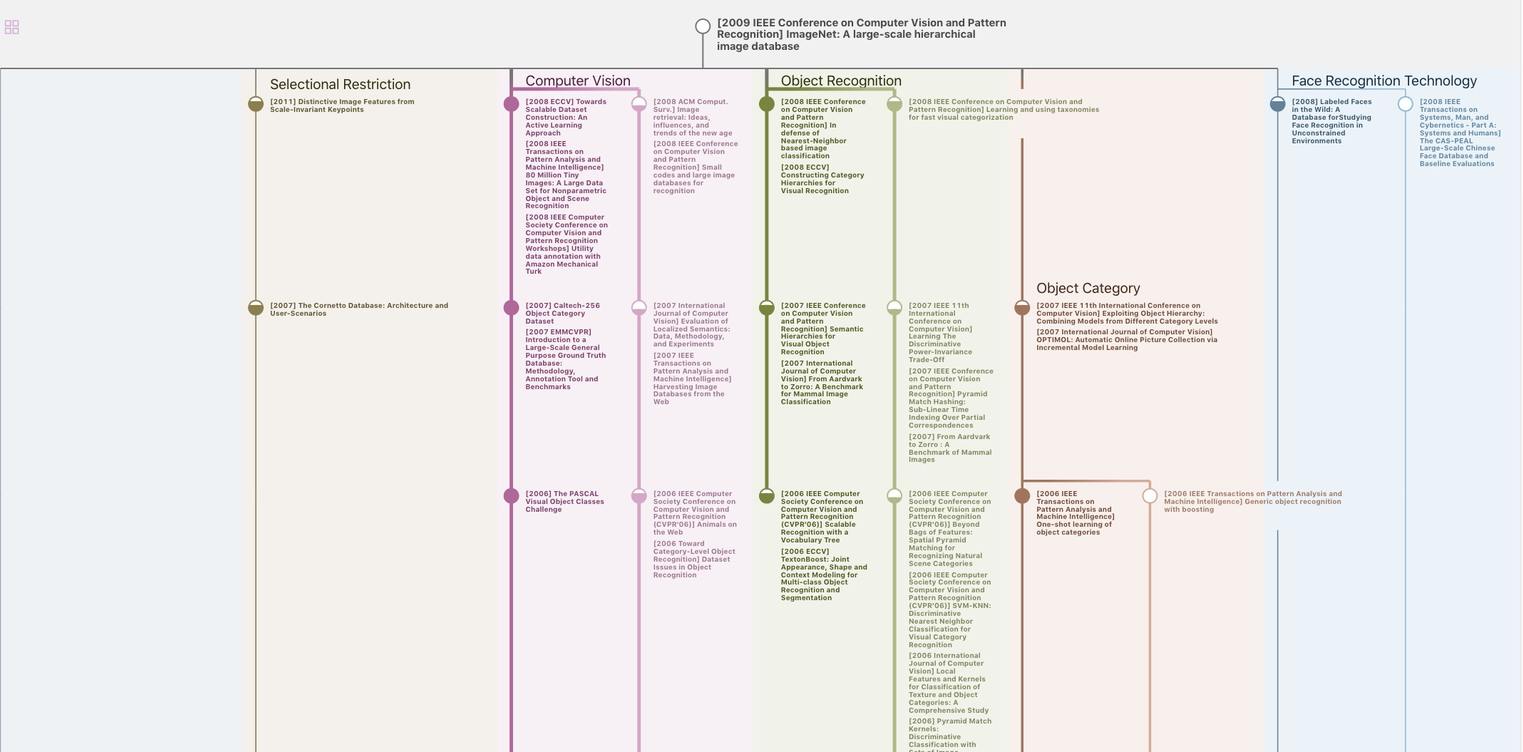
生成溯源树,研究论文发展脉络
Chat Paper
正在生成论文摘要