Use of Evolutionary Optimization Algorithms for the Design and Analysis of Low Bias, Low Phase Noise Photodetectors
JOURNAL OF LIGHTWAVE TECHNOLOGY(2023)
摘要
With the rapid advance of machine learning techniques and the increased availability of high-speed computing resources, it has become possible to exploit machine-learning technologies to aid in the design of photonic devices. In this work we use evolutionary optimization algorithms, machine learning techniques, and the drift-diffusion equations to optimize a modified uni-traveling-carrier (MUTC) photodetector for low phase noise at a relatively low bias of 5 V. We compare the particle swarm optimization (PSO), genetic, and surrogate optimization algorithms. We find that PSO yields the solution with the lowest phase noise, with an improvement over a current design of 4.4 dBc/Hz. We then analyze the machine-optimized design to understand the physics behind the phase noise reduction and show that the optimized design removes electrical bottlenecks in the current design.
更多查看译文
关键词
Photodetectors,Optimization,Phase noise,Doping,Mathematical models,Semiconductor process modeling,Computational modeling,Frequency combs,optimization,photodetectors
AI 理解论文
溯源树
样例
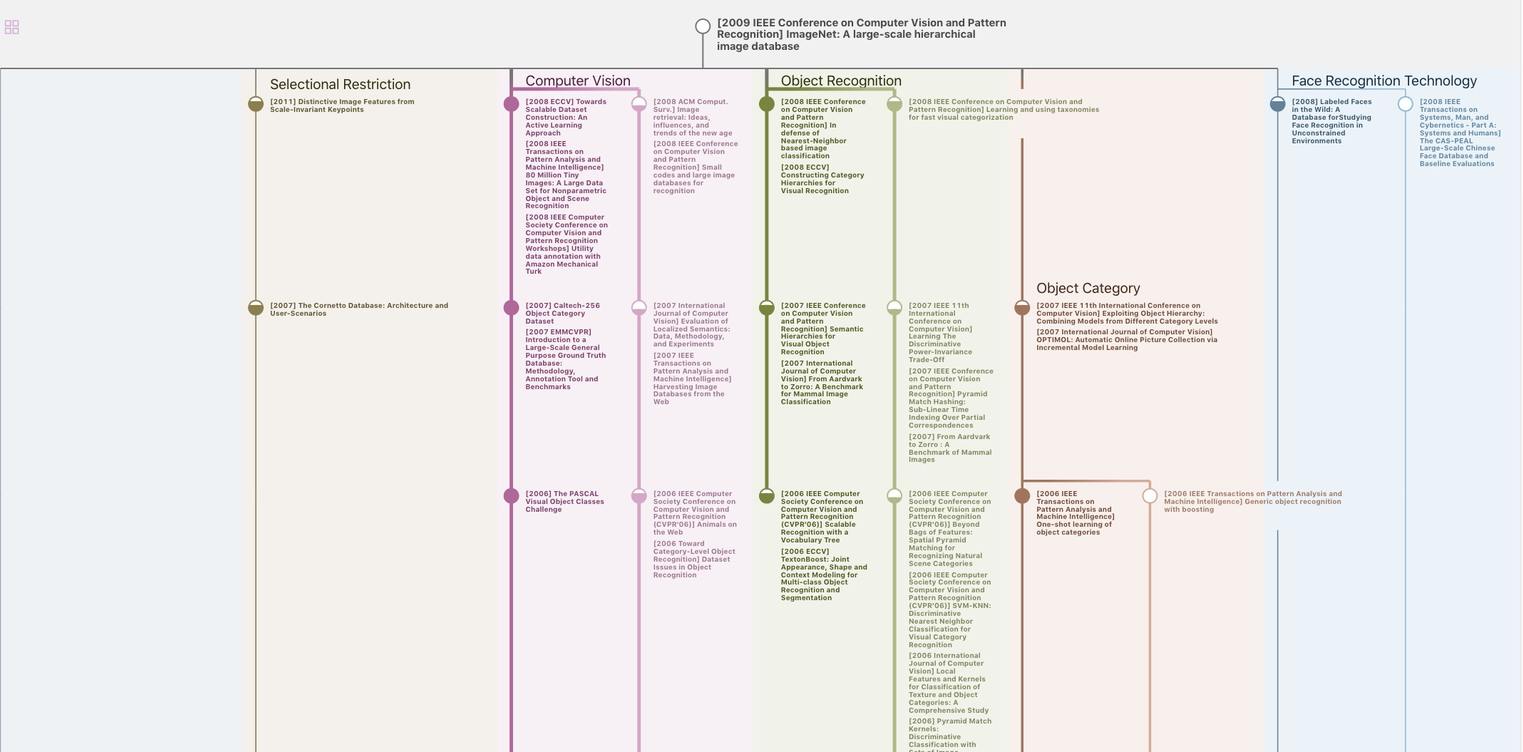
生成溯源树,研究论文发展脉络
Chat Paper
正在生成论文摘要