Time-Frequency Domain Deep Convolutional Neural Network for Li-Ion Battery SoC Estimation
IEEE TRANSACTIONS ON POWER ELECTRONICS(2024)
摘要
The state of charge (SoC) estimation is essential for many battery-related applications, such as electric vehicles, unmanned aerial vehicles, and uninterruptible power supplies. This article presents a novel deep neural network for the SoC estimation on the time-frequency domain. Contrary to previous studies operating only in the time domain or extracting features using a 1-D convolutional neural network (CNN), the proposed model extracts high-level information features for more accurate SoC estimation through 2-D time-frequency domain spectrogram analysis using CNN. The spectrogram helped improve the model's generalization performance through the SpecAugment technique. The proposed model aggregates intermediate features and captures long-term hierarchical context information by introducing modified atrous spatial pyramid pooling. In addition, by introducing CNN with depthwise separable operations, the proposed model improves the estimation error score and reduces the computational cost compared with existing competing models. Experimental results indicate that the proposed approach outperforms the previous baseline methods and achieves remarkable performance in SoC estimation.
更多查看译文
关键词
Convolutional neural network (CNN),deep learning (DL),depthwise-separable convolution (DWS CNN),lithium-ion battery,state-of-charge (SoC)
AI 理解论文
溯源树
样例
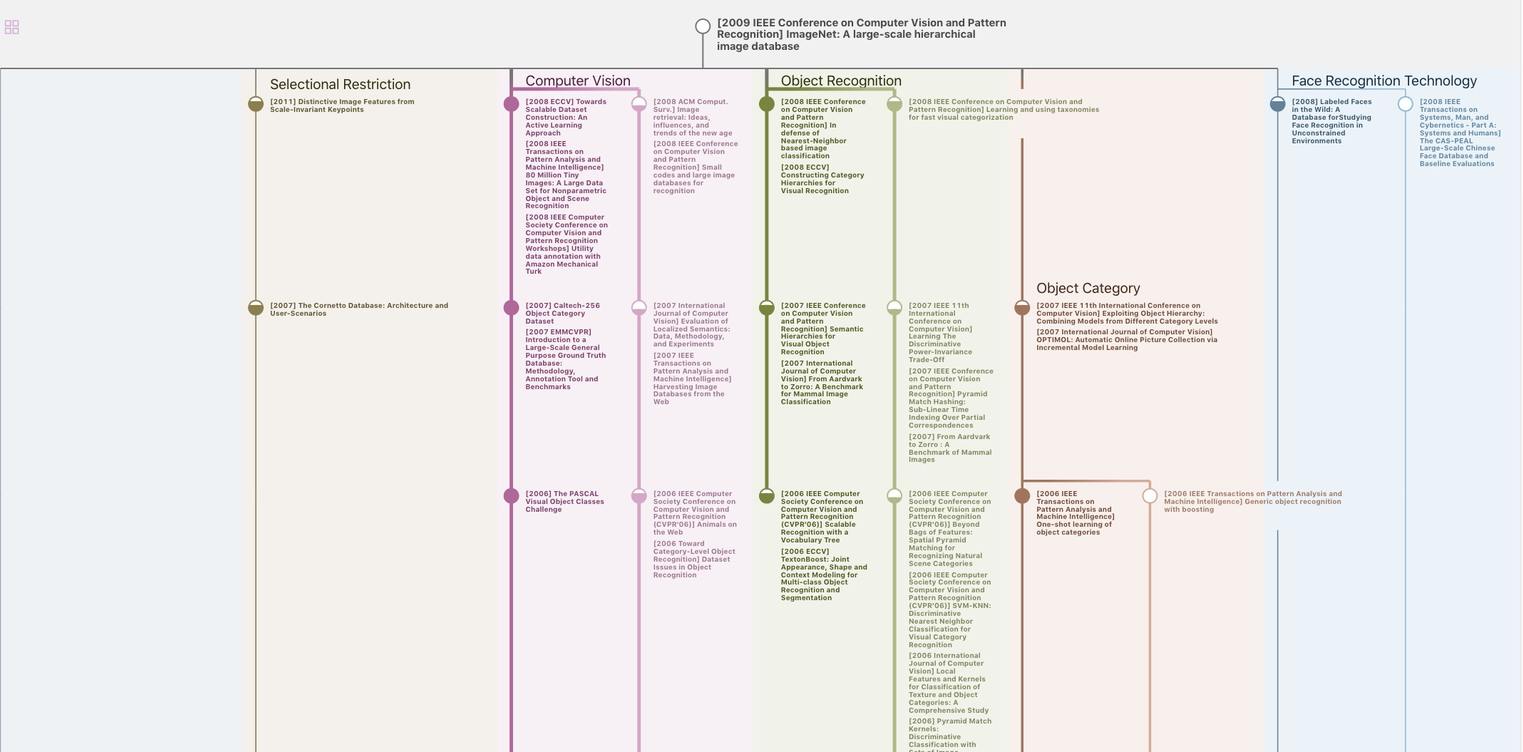
生成溯源树,研究论文发展脉络
Chat Paper
正在生成论文摘要