Weighting Factors Optimization for FCS-MPC in PMSM Drives Using Aggregated Residual Network
IEEE TRANSACTIONS ON POWER ELECTRONICS(2024)
摘要
Artificial neural network (ANN) has recently been considered in weighting factors (WFs) optimization for finite control-set model predictive control (FCS-MPC). However, the training of the traditional ANN is complicated and the predictive accuracy is limited by gradient vanishing. For this motivation, this article proposes a WFs optimization method based on an aggregated residual network (ResNet), which combines the advantages of residual connection and aggregated transformation. Specifically, the residual connection avoids the gradient vanishing of ANN while the aggregated transformation simplifies the training procedure. Furthermore, the input layer of the designed network incorporates the parameters and working conditions of permanent magnet synchronous motor (PMSM). Consequently, the proposed method is capable of precisely predicting the optimal WFs to enhance the performance of FCS-MPC under various operating conditions. Comparative studies are conducted to highlight the training and generalization ability of the aggregated ResNet. Finally, the feasibility and the dynamic adjustment of the predicted WFs are experimentally validated through a platform of PMSM drives fed by three-level neutral-point-clamped inverter.
更多查看译文
关键词
Cost function,Inverters,Voltage control,Switches,Optimization,Stators,Torque,Aggregated residual network,model predictive control,neutral-point-clamped inverter,permanent magnet synchronous motor,weighting factors
AI 理解论文
溯源树
样例
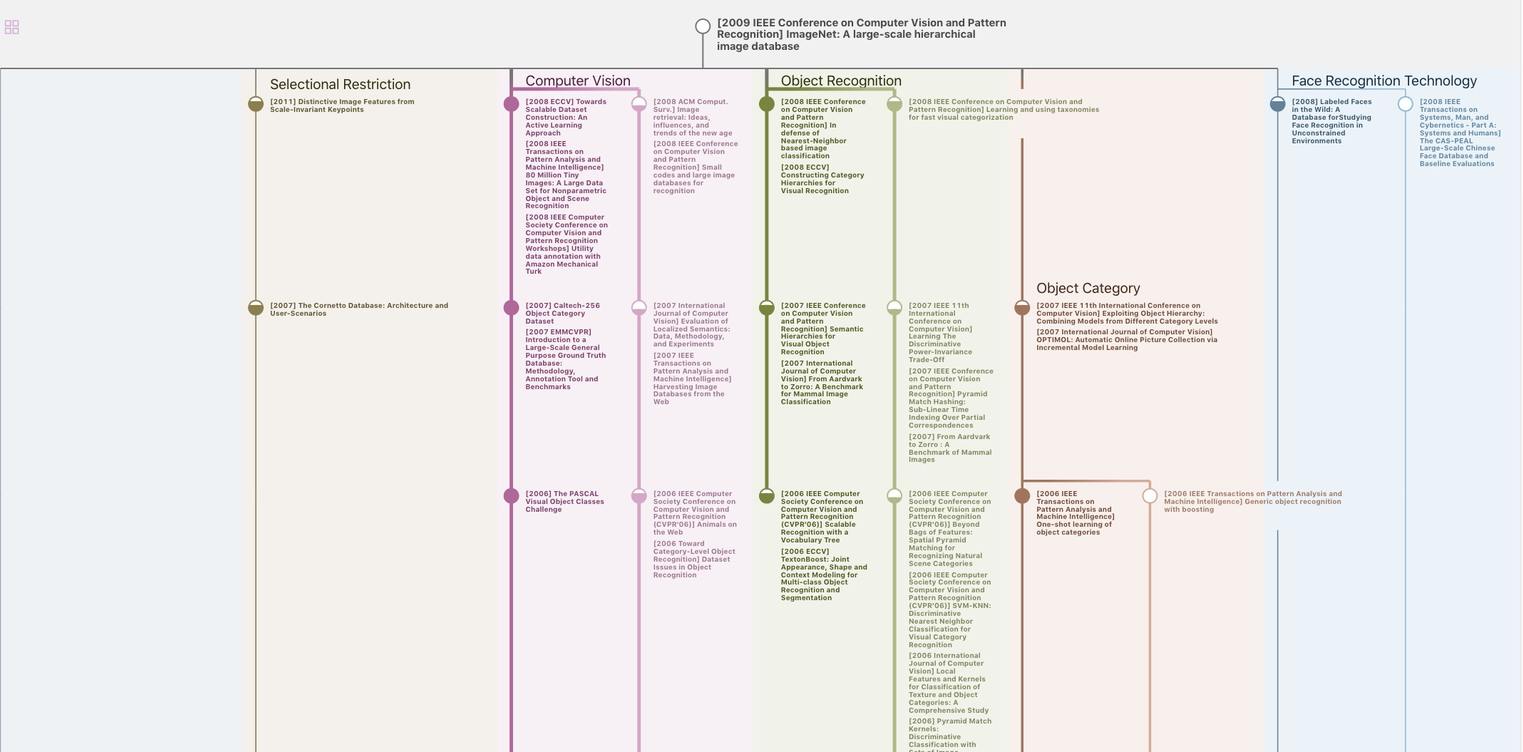
生成溯源树,研究论文发展脉络
Chat Paper
正在生成论文摘要