MRL-Seg: Overcoming Imbalance in Medical Image Segmentation With Multi-Step Reinforcement Learning.
IEEE journal of biomedical and health informatics(2024)
摘要
Medical image segmentation is a critical task for clinical diagnosis and research. However, dealing with highly imbalanced data remains a significant challenge in this domain, where the region of interest (ROI) may exhibit substantial variations across different slices. This presents a significant hurdle to medical image segmentation, as conventional segmentation methods may either overlook the minority class or overly emphasize the majority class, ultimately leading to a decrease in the overall generalization ability of the segmentation results. To overcome this, we propose a novel approach based on multi-step reinforcement learning, which integrates prior knowledge of medical images and pixel-wise segmentation difficulty into the reward function. Our method treats each pixel as an individual agent, utilizing diverse actions to evaluate its relevance for segmentation. To validate the effectiveness of our approach, we conduct experiments on four imbalanced medical datasets, and the results show that our approach surpasses other state-of-the-art methods in highly imbalanced scenarios. These findings hold substantial implications for clinical diagnosis and research.
更多查看译文
关键词
imbalanced medical image segmentation,deep learning,radiomics,reinforcement learning
AI 理解论文
溯源树
样例
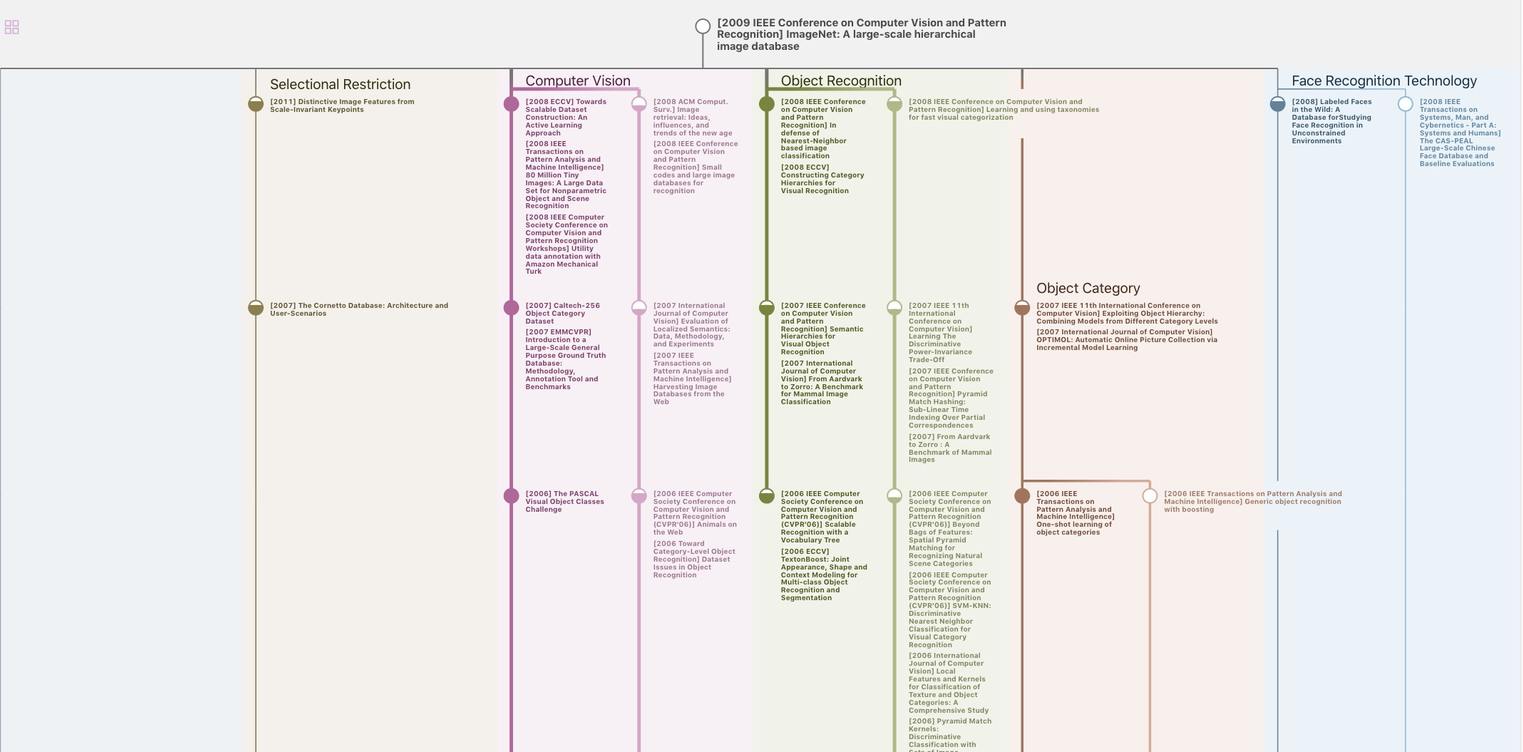
生成溯源树,研究论文发展脉络
Chat Paper
正在生成论文摘要