Standardizing fairness-evaluation procedures: interdisciplinary insights on machine learning algorithms in creditworthiness assessments for small personal loans
AI and Ethics(2023)
摘要
In the current European debate on the regulation of Artificial Intelligence there is a consensus that Artificial Intelligence (AI) systems should be fair. However, the multitude of existing indicators allowing an AI system to be labeled as “(un)fair” and the lack of standardized, application field specific criteria to choose among the various fairness-evaluation methods makes it difficult for potential auditors to arrive at a final, consistent judgment. Focusing on a concrete use case in the application field of finance, the main goal of this paper is to define standardizable minimal ethical requirements for AI fairness-evaluation. For the applied case of creditworthiness assessment for small personal loans, we highlighted specific distributive and procedural fairness issues inherent either to the computing process or to the system’s use in a real-world scenario: (1) the unjustified unequal distribution of predictive outcome; (2) the perpetuation of existing bias and discrimination practices; (3) the lack of transparency concerning the processed data and of an explanation of the algorithmic outcome for credit applicants. We addressed these issues proposing minimal ethical requirements for this specific application field: (1) regularly checking algorithmic outcome through the conditional demographic parity metric; (2) excluding from the group of processed parameters those that could lead to discriminatory outcome; (3) guaranteeing transparency about the processed data, in addition to counterfactual explainability of algorithmic decisions. Defining these minimal ethical requirements represents the main contribution of this paper and a starting point toward standards specifically addressing fairness issues in AI systems for creditworthiness assessments aiming at preventing unfair algorithmic outcomes, in addition to unfair practices related to the use of these systems. As a final result, we indicate the next steps that can be taken to begin the standardization of the three use case-specific fairness requirements we propose.
更多查看译文
关键词
Artificial intelligence,Data science,Fairness,Fairness metric,Standardization
AI 理解论文
溯源树
样例
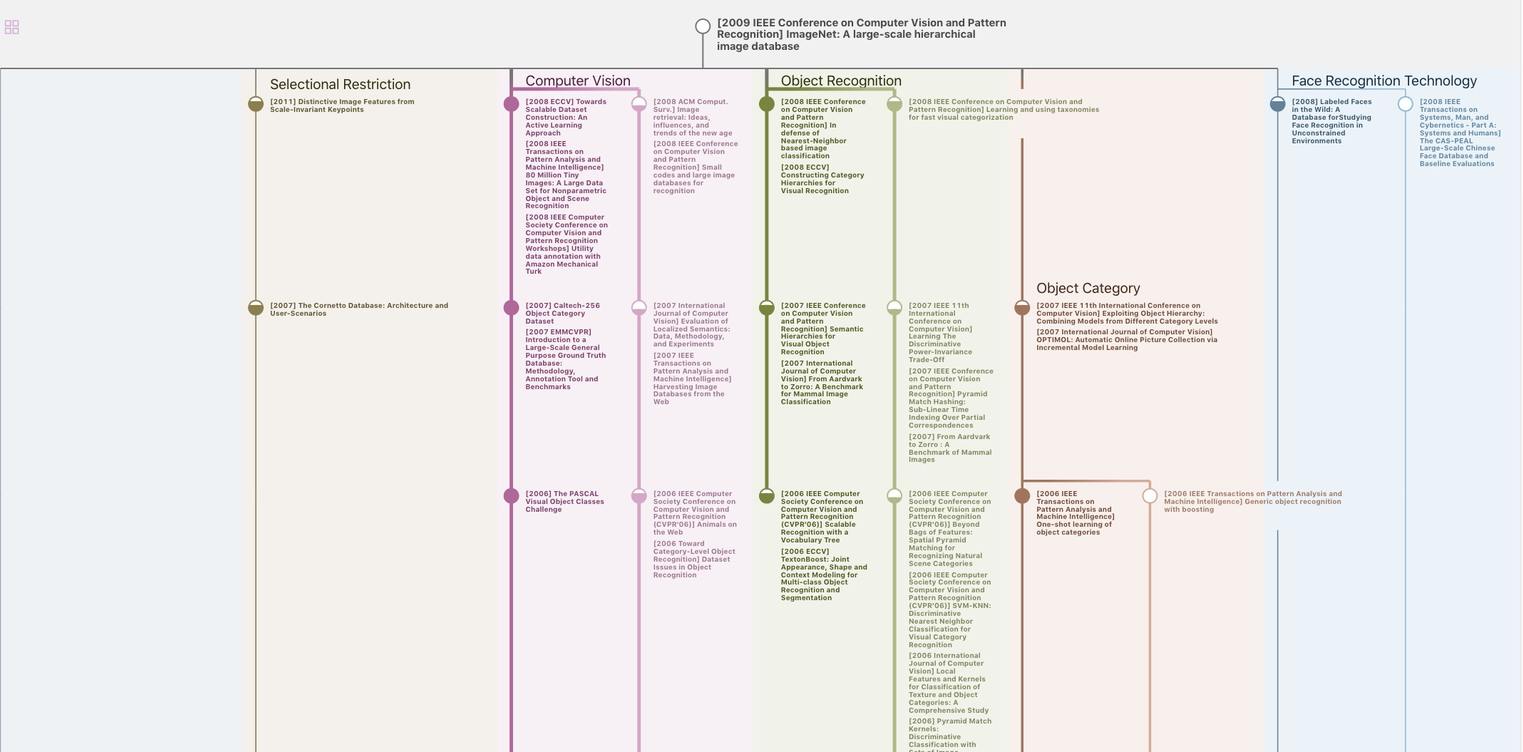
生成溯源树,研究论文发展脉络
Chat Paper
正在生成论文摘要