Random Quantum Neural Networks for Noisy Image Recognition
2023 IEEE International Conference on Quantum Computing and Engineering (QCE)(2023)
摘要
The application of classical Random Neural Networks (RNN) has been restricted to deterministic digital systems that generate probability distributions instead of the stochastic characteristics of random spiking signals. To optimize the utilization of the stochastic properties inherent in RNN, we propose a new category of supervised Random Quantum Neural Networks (RQNN) that incorporate a resilient training methodology. The RQNN under consideration utilizes a combination of classical and quantum algorithms, incorporating superposition state and angle encoding characteristics that draw inspiration from quantum information theory. Additionally, the model incorporates the stochastic random spiking property of neuron information encoding, which is known to exhibit spatial-temporal features similar to those observed in the brain. The proposed RQNN model has undergone thorough validation, relying on hybrid classical-quantum algorithms through a real IBM quantum processor. The proposed RQNN model is tested on the MNIST, Fashion-MNIST, and KMNIST datasets. The results indicate that it achieved an average classification accuracy of 59.6% when presented with unseen noisy test images. The experimental results demonstrate the efficacy and robustness of the proposed RQNN
1
1
The early version of the work is posted in arXiv: https://doi.org/10.48550/arXiv.2203.01764. in classifying noisy images that have not been previously encountered.
更多查看译文
关键词
Quantum computing,NISQ devices,Qubits,Random Neural Networks
AI 理解论文
溯源树
样例
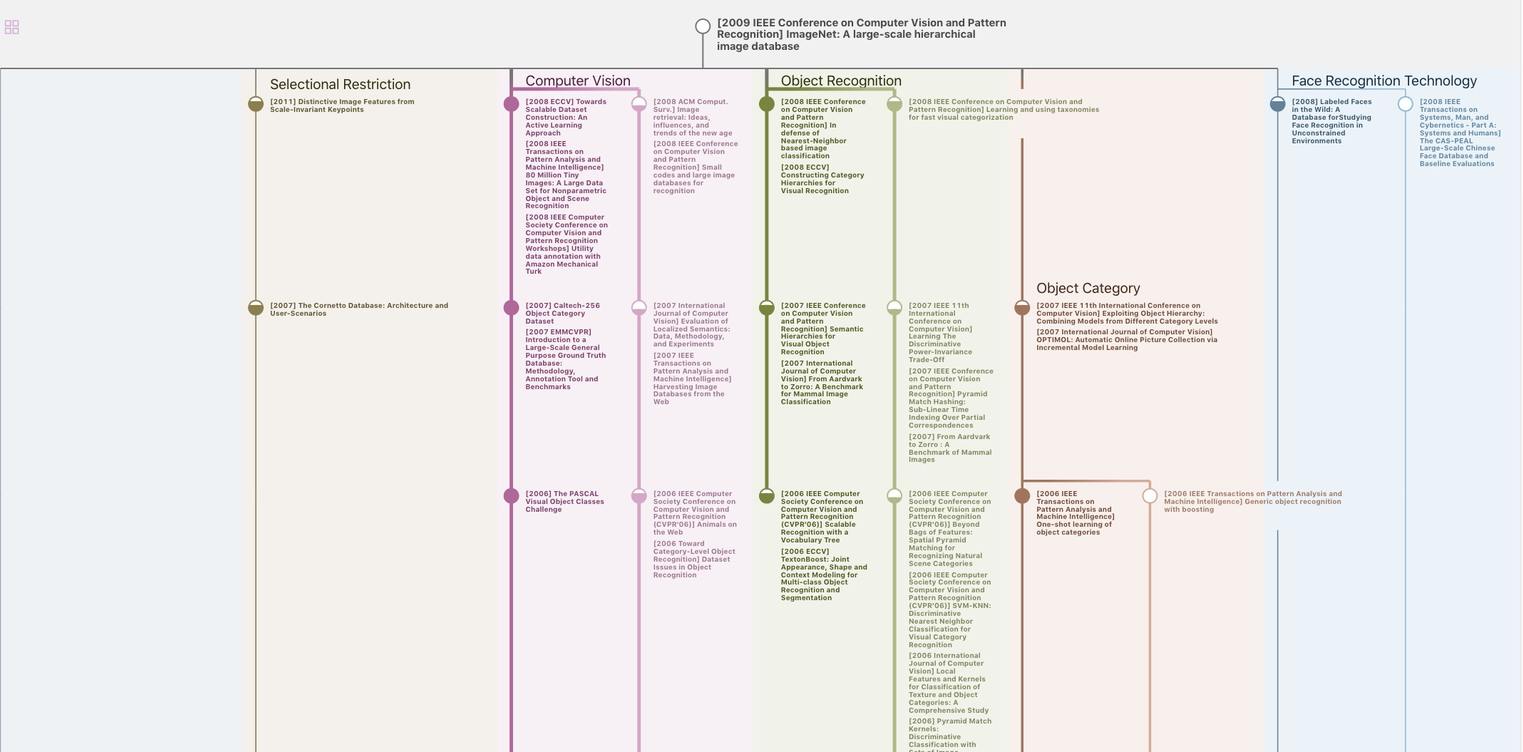
生成溯源树,研究论文发展脉络
Chat Paper
正在生成论文摘要