Resolution-Enhanced Electromagnetic Inverse Source: A Deep Learning Approach
IEEE ANTENNAS AND WIRELESS PROPAGATION LETTERS(2023)
摘要
We investigate the capabilities of deep learning based on a convolutional neural network (CNN) to improve the solution of an electromagnetic inverse source problem against a classical regularization scheme, the truncated singular value decomposition (TSVD). We consider a planar, scalar source and a far-zone observation domain, for which the unknown-to-data relation is provided by a two-dimensional Fourier-like operator. The exploited a priori information is a weak geometrical information for TSVD, whereas for CNN a priori information is the one embedded during the training stage. As long as the objects belong to a subset matching the information used for the training stage, the nonlinear processing of the neural network (NN) outperforms the linear processing of the TSVD by extrapolating out-of-band harmonics. On the other side, the NN performs poorly when the object does not match the a priori information. The results are of general interest for problems where the Fourier inversion is considered.
更多查看译文
关键词
Deep learning,inverse source,number of degrees freedom,singular value decomposition
AI 理解论文
溯源树
样例
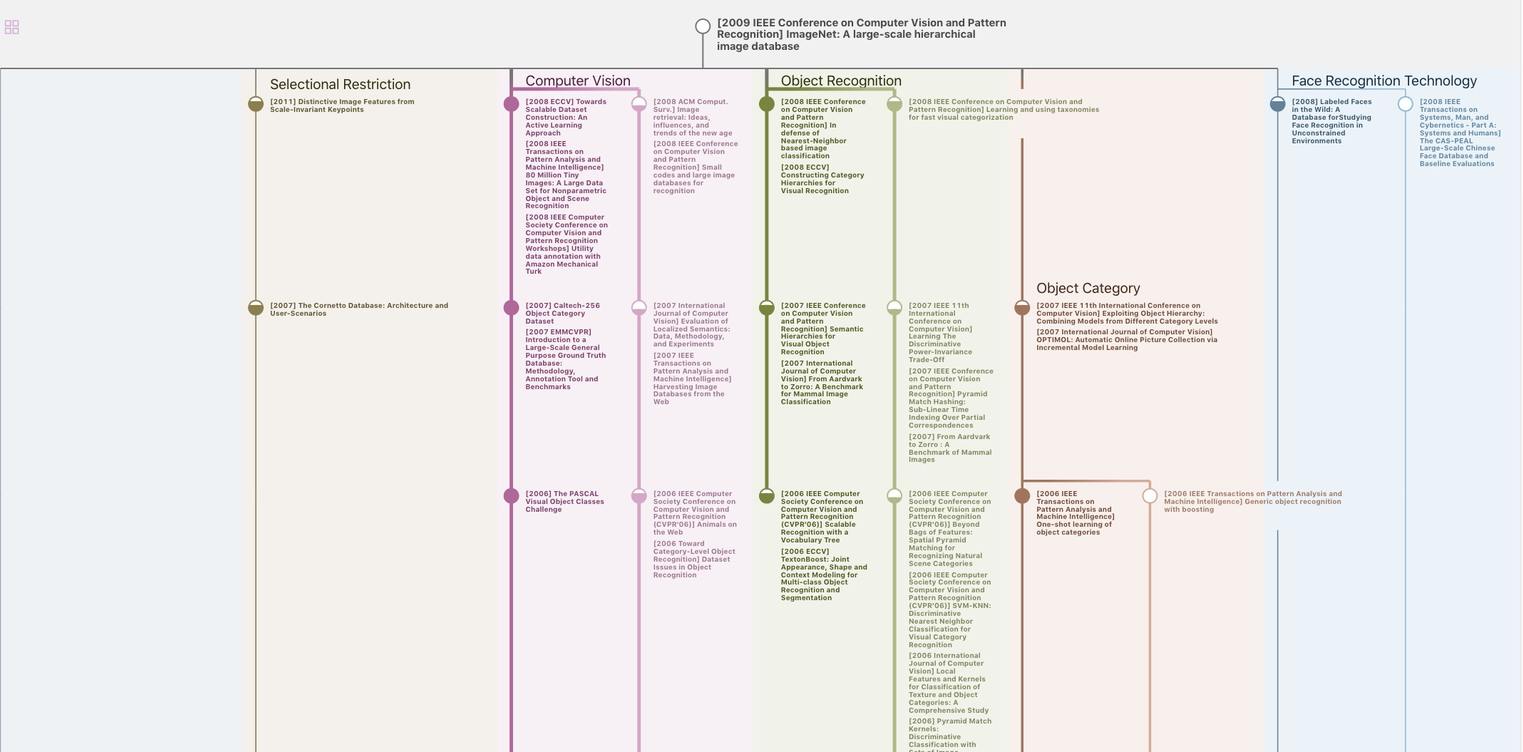
生成溯源树,研究论文发展脉络
Chat Paper
正在生成论文摘要